Chi Squared Goodness Of Fit Test Calculator
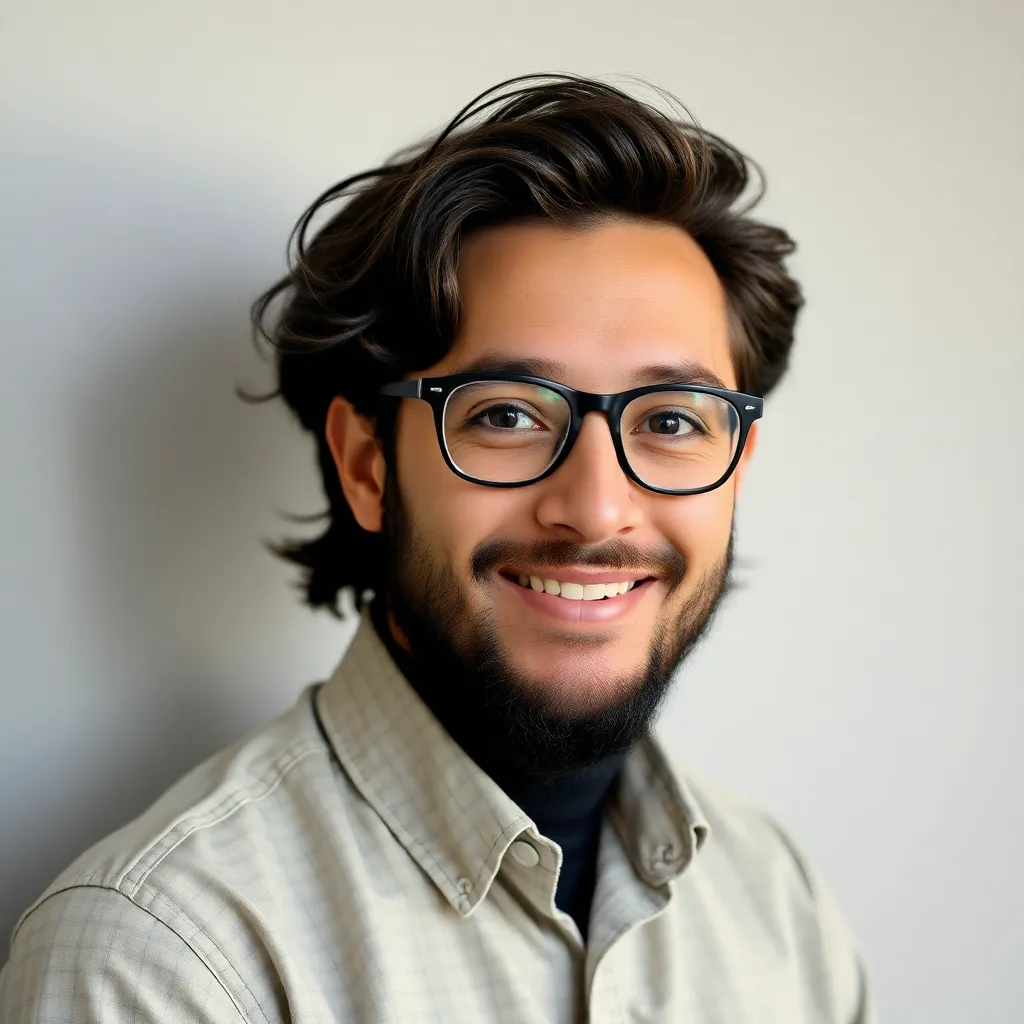
Treneri
May 12, 2025 · 6 min read
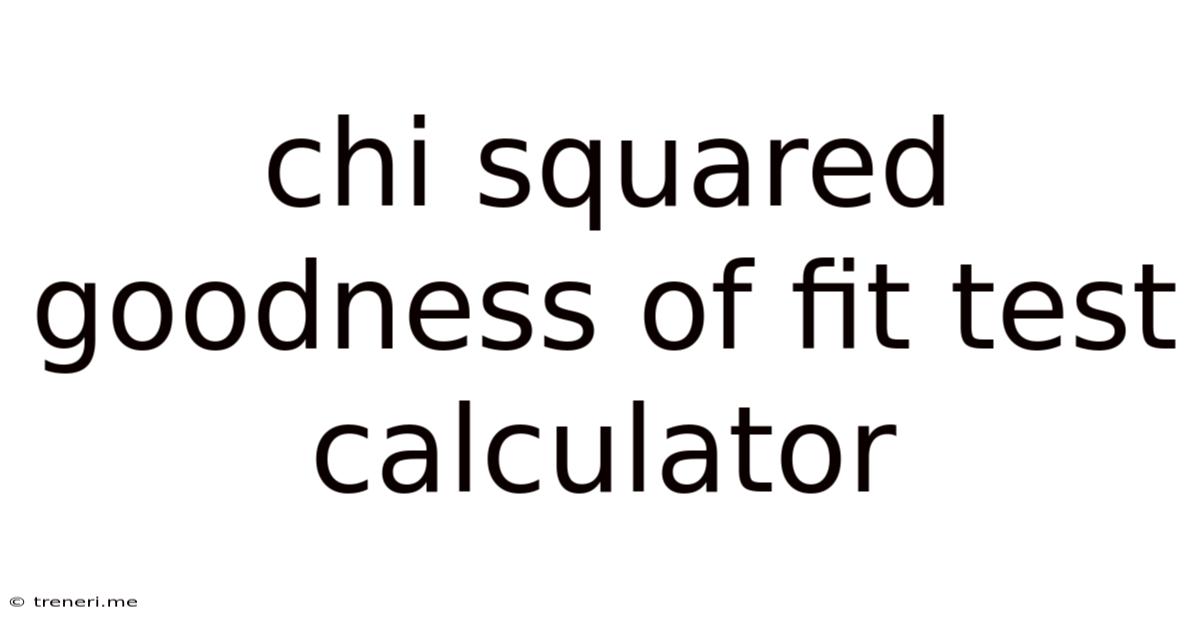
Table of Contents
Chi-Squared Goodness-of-Fit Test Calculator: A Comprehensive Guide
The chi-squared goodness-of-fit test is a powerful statistical tool used to determine if a sample data set matches a hypothesized population. It's particularly useful when dealing with categorical data, allowing us to assess how well observed frequencies align with expected frequencies based on a specific distribution or model. This comprehensive guide will delve into the intricacies of this test, explaining its applications, the underlying calculations, and how to effectively use a chi-squared goodness-of-fit test calculator.
Understanding the Chi-Squared Goodness-of-Fit Test
At its core, the chi-squared goodness-of-fit test compares observed frequencies (the actual counts from your sample) with expected frequencies (the counts you'd anticipate if your data perfectly matched your hypothesized distribution). A significant difference between these frequencies suggests your data doesn't fit the hypothesized distribution well.
Key Concepts:
- Observed Frequency (O): The actual number of observations in each category of your data.
- Expected Frequency (E): The number of observations expected in each category based on your hypothesis. This is calculated using your hypothesized distribution.
- Degrees of Freedom (df): This represents the number of independent pieces of information used to calculate the chi-squared statistic. For a goodness-of-fit test, it's typically the number of categories minus 1 (df = k - 1, where k is the number of categories).
- Chi-Squared Statistic (χ²): The calculated value that measures the discrepancy between observed and expected frequencies. A larger χ² indicates a greater difference between observed and expected values.
- P-value: The probability of observing the obtained χ² value (or a more extreme value) if the null hypothesis (that the data fits the hypothesized distribution) is true. A small p-value (typically below a significance level, often 0.05) leads to rejection of the null hypothesis.
Calculating the Chi-Squared Statistic
The chi-squared statistic is calculated using the following formula:
χ² = Σ [(O - E)² / E]
Where:
- Σ represents the sum across all categories.
- O is the observed frequency in each category.
- E is the expected frequency in each category.
Let's illustrate with an example:
Suppose you're testing if a six-sided die is fair. You roll the die 60 times and obtain the following results:
Face | Observed Frequency (O) |
---|---|
1 | 8 |
2 | 12 |
3 | 9 |
4 | 10 |
5 | 11 |
6 | 10 |
If the die is fair, the expected frequency for each face is 60/6 = 10. Now, we can calculate the chi-squared statistic:
χ² = [(8-10)²/10] + [(12-10)²/10] + [(9-10)²/10] + [(10-10)²/10] + [(11-10)²/10] + [(10-10)²/10] = 0.8
Using a Chi-Squared Goodness-of-Fit Test Calculator
Manually calculating the chi-squared statistic, especially with numerous categories, can be time-consuming and prone to errors. This is where a chi-squared goodness-of-fit test calculator becomes invaluable. These calculators streamline the process, allowing you to input your observed frequencies and expected frequencies, and instantly receive the chi-squared statistic, degrees of freedom, and p-value.
Features of a Good Calculator:
- Ease of Use: The calculator should have a clear and intuitive interface, making it easy to input data and interpret results.
- Multiple Input Methods: The ability to input data in various formats (e.g., tables, lists) enhances flexibility.
- Detailed Output: The calculator should provide not only the χ², df, and p-value but also a clear interpretation of the results, including whether the null hypothesis is rejected or not.
- Customization Options: Options to adjust the significance level (alpha) are crucial for adapting the test to different contexts.
Interpreting the Results
Once you obtain the chi-squared statistic and p-value from the calculator, you need to interpret the results in relation to your chosen significance level (alpha). Typically, alpha is set at 0.05.
-
If the p-value is less than alpha (e.g., p < 0.05): You reject the null hypothesis. This means there is sufficient evidence to suggest that your observed data does not fit the hypothesized distribution. The difference between observed and expected frequencies is statistically significant.
-
If the p-value is greater than or equal to alpha (e.g., p ≥ 0.05): You fail to reject the null hypothesis. This means there is not enough evidence to conclude that your observed data deviates significantly from the hypothesized distribution. The differences observed could be due to random chance.
Important Considerations:
- Expected Frequency Assumption: The chi-squared test is most reliable when expected frequencies in each category are reasonably large (generally, E ≥ 5). If expected frequencies are too low, the chi-squared approximation might be inaccurate. In such cases, consider alternative methods or combining categories to meet the assumption.
- Sample Size: A larger sample size provides more statistical power, increasing the likelihood of detecting a true difference between observed and expected frequencies.
- Data Independence: The observations in your data must be independent of each other.
Applications of the Chi-Squared Goodness-of-Fit Test
The chi-squared goodness-of-fit test finds widespread application across various fields:
- Genetics: Testing if observed genotype frequencies match Hardy-Weinberg equilibrium expectations.
- Quality Control: Assessing if the distribution of a product characteristic conforms to specifications.
- Market Research: Comparing observed customer preferences against a hypothesized distribution.
- Medicine: Determining if the incidence of a disease is consistent across different demographic groups.
- Ecology: Evaluating if species distribution follows a particular pattern.
Beyond the Basics: Advanced Applications
The chi-squared goodness-of-fit test can be extended to more complex scenarios:
- Testing for Specific Distributions: You can test if your data fits specific probability distributions such as the normal distribution, Poisson distribution, or binomial distribution. This requires calculating expected frequencies based on the parameters of the chosen distribution.
- Analyzing Multiple Variables: Although the basic test focuses on a single categorical variable, extensions exist to handle multiple variables using techniques like contingency tables and log-linear models.
Conclusion
The chi-squared goodness-of-fit test is a fundamental tool for assessing the fit between observed and expected frequencies in categorical data. Utilizing a chi-squared goodness-of-fit test calculator simplifies the calculations and ensures accuracy, allowing researchers and analysts to focus on interpreting the results and drawing meaningful conclusions. Understanding the underlying principles, assumptions, and limitations of the test is crucial for proper application and interpretation, ensuring reliable and valid inferences about the data being analyzed. Remember to always consider the context of your data and choose the appropriate statistical test to answer your research question effectively. By mastering this test, you'll enhance your ability to analyze categorical data and gain valuable insights from various datasets.
Latest Posts
Latest Posts
-
How Many Square Feet Is 24 By 24
May 13, 2025
-
How Many Tbsp Is 5 Cloves Of Garlic
May 13, 2025
-
Average 0 60 Time For A Car
May 13, 2025
-
How Much Is 140 Ml In Cups
May 13, 2025
-
Cuadro De Punnett Color De Ojos
May 13, 2025
Related Post
Thank you for visiting our website which covers about Chi Squared Goodness Of Fit Test Calculator . We hope the information provided has been useful to you. Feel free to contact us if you have any questions or need further assistance. See you next time and don't miss to bookmark.