Find And Interpret The Z-score For The Data Value Given
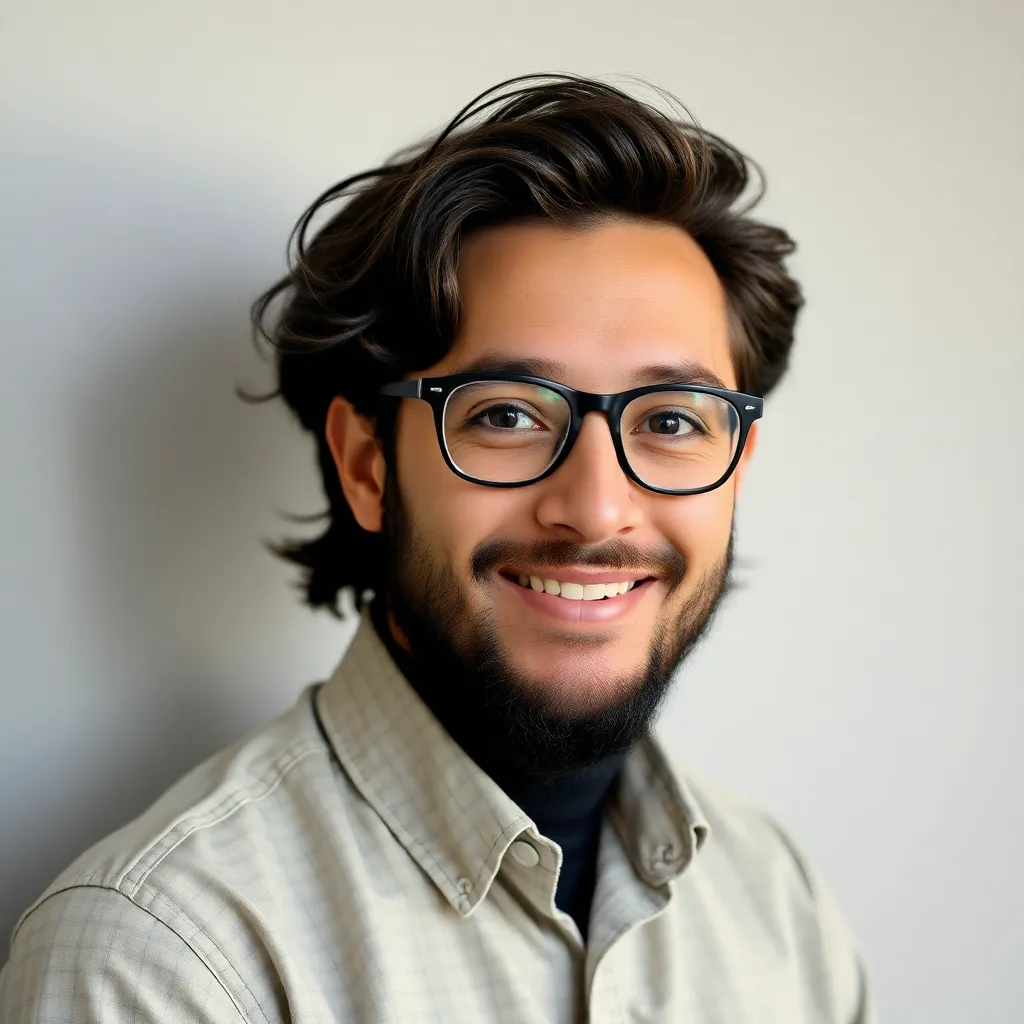
Treneri
Apr 26, 2025 · 6 min read

Table of Contents
Find and Interpret the Z-Score for a Given Data Value
Understanding and utilizing z-scores is crucial in statistics for a variety of applications. This comprehensive guide will walk you through the process of finding and interpreting z-scores for given data values, explaining the underlying concepts and providing practical examples. We'll cover the formula, its interpretation, and how z-scores facilitate comparisons across different datasets.
What is a Z-Score?
A z-score, also known as a standard score, represents the number of standard deviations a data point lies above or below the mean of a data set. It standardizes data, allowing for meaningful comparisons between different distributions, even if they have different means and standard deviations. A positive z-score indicates the data point is above the mean, while a negative z-score indicates it's below the mean. A z-score of 0 means the data point is exactly at the mean.
The Z-Score Formula
The formula for calculating a z-score is straightforward:
z = (x - μ) / σ
Where:
- z is the z-score
- x is the individual data point
- μ (mu) is the population mean
- σ (sigma) is the population standard deviation
Important Note: If you are working with a sample instead of the entire population, you would use the sample mean (x̄) and sample standard deviation (s) in place of μ and σ respectively. However, the interpretation remains the same.
Step-by-Step Calculation of a Z-Score
Let's illustrate the z-score calculation with a concrete example. Suppose we have a dataset representing the heights of adult women in a particular city. The mean height (μ) is 65 inches, and the standard deviation (σ) is 3 inches. We want to find the z-score for a woman who is 70 inches tall (x = 70).
-
Identify the Data Point (x): x = 70 inches
-
Identify the Population Mean (μ): μ = 65 inches
-
Identify the Population Standard Deviation (σ): σ = 3 inches
-
Apply the Z-Score Formula:
z = (70 - 65) / 3 = 5 / 3 ≈ 1.67
Therefore, the z-score for a woman who is 70 inches tall is approximately 1.67. This means her height is 1.67 standard deviations above the average height of women in that city.
Interpreting Z-Scores
The interpretation of a z-score provides valuable insights into the relative position of a data point within its distribution. Here's a breakdown:
-
Z-score close to 0: The data point is very close to the mean. A z-score of 0 indicates the data point is exactly at the mean.
-
Positive Z-score: The data point lies above the mean. The larger the positive z-score, the further above the mean the data point is.
-
Negative Z-score: The data point lies below the mean. The larger the absolute value of the negative z-score (e.g., -2 is larger than -1), the further below the mean the data point is.
-
Z-scores and Probability: Z-scores are closely linked to probabilities. Using a standard normal distribution table (also known as a z-table) or statistical software, you can determine the probability of observing a data point with a z-score less than or equal to a specific value. For example, a z-score of 1.67 corresponds to a cumulative probability of approximately 0.9525, meaning there's roughly a 95.25% chance of observing a height less than or equal to 70 inches in this population.
Z-Scores and Outliers
Z-scores are also valuable in identifying potential outliers. Data points with unusually high or low z-scores (typically those exceeding ±3) are often considered outliers, suggesting they might be errors in data entry or represent truly exceptional cases. However, the threshold for identifying outliers using z-scores can depend on the context and the specific application. Some researchers might use a more lenient threshold (e.g., ±2), while others use more stringent criteria.
Applications of Z-Scores
Z-scores have a wide range of applications across diverse fields:
-
Standardizing Test Scores: Converting raw test scores into z-scores allows for fair comparisons between different tests or groups of test-takers, even if the tests have different scoring scales.
-
Quality Control: In manufacturing, z-scores can be used to monitor the quality of products by identifying items that fall outside acceptable ranges.
-
Finance: Z-scores are used in financial risk management to assess the probability of default for borrowers or investments.
-
Medical Research: Z-scores help researchers compare measurements across different patient populations or experimental groups.
-
Data Visualization: Z-scores can enhance data visualization by standardizing values for improved clarity and interpretability in charts and graphs.
Calculating Z-Scores with Sample Data
When working with sample data (a subset of the population), you'll use the sample mean (x̄) and sample standard deviation (s) in the z-score formula:
z = (x - x̄) / s
The interpretation of the z-score remains the same, but remember that you are making inferences about the population based on sample data. The accuracy of your inference depends on the size and representativeness of your sample.
Z-Scores and the Central Limit Theorem
The central limit theorem (CLT) plays a crucial role when dealing with z-scores and sample means. The CLT states that the distribution of sample means approaches a normal distribution as the sample size increases, regardless of the shape of the original population distribution. This makes z-scores incredibly useful for hypothesis testing and making inferences about population parameters based on sample data.
Limitations of Z-Scores
While z-scores are a powerful tool, it's essential to be aware of their limitations:
-
Assumption of Normality: The interpretation of z-scores relies on the assumption that the data is approximately normally distributed. If the data deviates significantly from normality, z-scores may not be a reliable measure of relative position.
-
Sensitivity to Outliers: Outliers can disproportionately influence the mean and standard deviation, potentially distorting the z-scores of other data points.
-
Interpretation Depends on Context: The meaning of a particular z-score is heavily dependent on the specific context of the data. A z-score of 2 might be considered large in one context but small in another.
Beyond Z-Scores: Other Standardization Methods
While z-scores are commonly used, other standardization methods exist, including:
-
T-scores: Similar to z-scores but have a mean of 50 and a standard deviation of 10.
-
Standardized Scores with Different Means and Standard Deviations: You can standardize data to have any desired mean and standard deviation. This allows for customization based on specific needs.
Conclusion: Mastering Z-Scores for Data Analysis
Understanding and applying z-scores is a cornerstone of statistical analysis. By mastering the calculation and interpretation of z-scores, you gain the ability to effectively standardize data, compare values across different distributions, identify outliers, and make informed decisions based on statistical evidence. Remember to always consider the assumptions underlying z-scores and be mindful of their limitations to ensure accurate and meaningful interpretations. This detailed guide equips you with the knowledge to confidently utilize z-scores in your own data analysis projects. Remember to always examine your data for normality and consider alternative methods if your data is significantly non-normal. The power of z-scores lies in its simplicity and broad applicability, making it an essential tool in any statistician's arsenal.
Latest Posts
Latest Posts
-
How Many Sq Ft Is 10x10
Apr 26, 2025
-
How Long For Drinks To Get Cold In Freezer
Apr 26, 2025
-
How Long Until 8 Pm Today
Apr 26, 2025
-
What Percent Of 80 Is 7
Apr 26, 2025
-
How Long Ago Was 1963 To 2024
Apr 26, 2025
Related Post
Thank you for visiting our website which covers about Find And Interpret The Z-score For The Data Value Given . We hope the information provided has been useful to you. Feel free to contact us if you have any questions or need further assistance. See you next time and don't miss to bookmark.