Find P Value For Chi Square
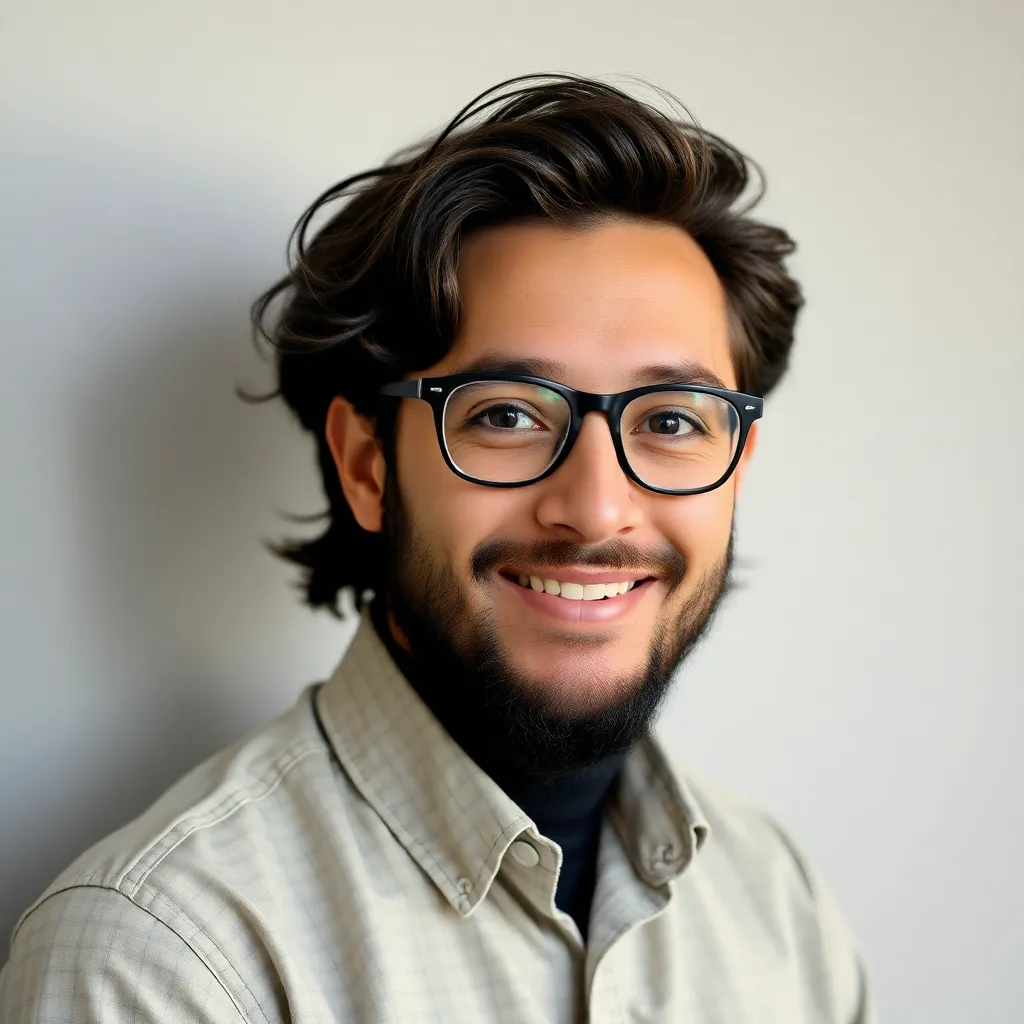
Treneri
May 14, 2025 · 7 min read
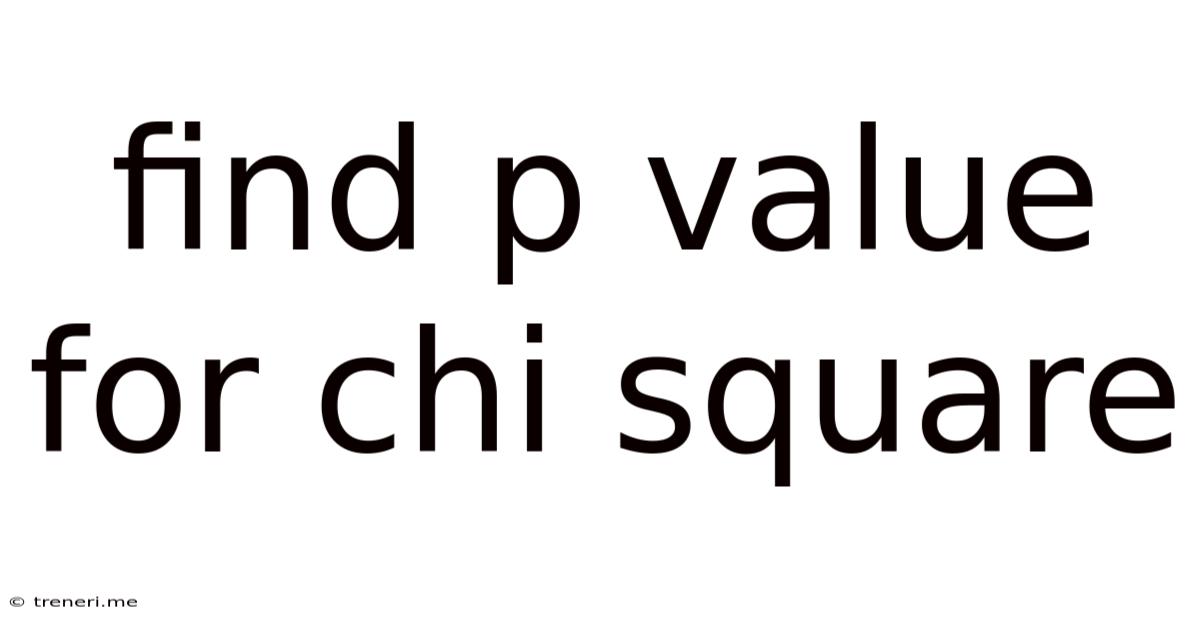
Table of Contents
Finding the P-Value for a Chi-Square Test: A Comprehensive Guide
The chi-square (χ²) test is a powerful statistical tool used to analyze categorical data. It determines whether there's a significant association between two categorical variables or whether a sample distribution matches an expected distribution. Understanding how to find the p-value associated with a chi-square test is crucial for interpreting the results and drawing valid conclusions. This comprehensive guide will walk you through the process, covering different types of chi-square tests and providing practical examples.
Understanding the Chi-Square Test and its P-Value
The chi-square test works by comparing observed frequencies with expected frequencies. A large difference between these frequencies indicates a statistically significant relationship or deviation from the expected distribution. The p-value, in this context, represents the probability of observing the obtained results (or more extreme results) if there were actually no association between the variables (the null hypothesis is true).
A low p-value (typically below 0.05) suggests strong evidence against the null hypothesis, indicating a statistically significant association or difference. Conversely, a high p-value (above 0.05) suggests insufficient evidence to reject the null hypothesis. It's important to note that a high p-value doesn't necessarily prove the null hypothesis is true; it simply means there's not enough evidence to reject it.
There are several types of chi-square tests, each serving a specific purpose:
-
Chi-Square Goodness-of-Fit Test: This test assesses whether a sample distribution conforms to a hypothesized distribution. For example, you might use this test to see if the distribution of colors in a bag of candy matches the manufacturer's stated proportions.
-
Chi-Square Test of Independence: This test examines whether two categorical variables are independent of each other. For instance, you could use this to see if there's an association between smoking status and lung cancer.
-
Chi-Square Test of Homogeneity: This test compares the distributions of a categorical variable across different groups or populations. For example, you might use it to determine if the distribution of political affiliations is the same across different age groups.
Calculating the Chi-Square Statistic
Before we delve into finding the p-value, let's briefly review how the chi-square statistic itself is calculated. The formula is:
χ² = Σ [(Oᵢ - Eᵢ)² / Eᵢ]
Where:
- χ² is the chi-square statistic.
- Oᵢ is the observed frequency for category i.
- Eᵢ is the expected frequency for category i.
- Σ denotes the sum across all categories.
Calculating expected frequencies varies depending on the type of chi-square test being performed. For the goodness-of-fit test, expected frequencies are derived from the hypothesized distribution. For tests of independence and homogeneity, expected frequencies are calculated using row and column totals.
Finding the P-Value: Different Approaches
Once you've calculated the chi-square statistic, you need to find the corresponding p-value. There are several ways to do this:
1. Using a Chi-Square Distribution Table
This is a traditional method, though less precise than software-based approaches. Chi-square distribution tables provide p-values for different degrees of freedom (df) and chi-square values. The degrees of freedom depend on the type of test and the number of categories.
- Goodness-of-Fit Test: df = number of categories - 1
- Test of Independence/Homogeneity: df = (number of rows - 1) * (number of columns - 1)
To use the table:
- Determine your degrees of freedom.
- Locate your calculated chi-square statistic in the table's row corresponding to your degrees of freedom.
- The p-value will be found in the column heading associated with your chi-square value. Note that tables typically only provide a range of p-values (e.g., 0.05 < p < 0.10).
2. Using Statistical Software
Statistical software packages like R, SPSS, SAS, Python (with libraries like SciPy), and Excel provide precise p-values for chi-square tests. These programs typically require you to input your observed data and the type of test you're performing. The software then automatically calculates the chi-square statistic and the associated p-value. This method is highly recommended due to its accuracy and efficiency.
3. Using Online Calculators
Numerous online chi-square calculators are available that can calculate the p-value based on the chi-square statistic and degrees of freedom. These calculators offer a convenient alternative to using tables or software, especially for quick calculations. However, always ensure that the calculator is reliable and from a reputable source.
Interpreting the P-Value and Drawing Conclusions
Once you have your p-value, you can compare it to your chosen significance level (alpha), typically set at 0.05.
-
If p ≤ α: You reject the null hypothesis. There is statistically significant evidence to suggest an association between the variables (for tests of independence and homogeneity) or a deviation from the expected distribution (for goodness-of-fit tests).
-
If p > α: You fail to reject the null hypothesis. There is insufficient evidence to suggest an association or deviation.
Important Considerations:
-
Statistical Significance vs. Practical Significance: A statistically significant result (low p-value) doesn't automatically mean the result is practically significant. The magnitude of the effect should also be considered. A small effect might be statistically significant with a large sample size but not practically meaningful.
-
Assumptions of the Chi-Square Test: The chi-square test assumes that the data are categorical, observations are independent, and expected frequencies are sufficiently large (generally, at least 5 in each cell). Violating these assumptions can affect the validity of the results.
-
Multiple Comparisons: When performing multiple chi-square tests, the risk of Type I error (false positive) increases. Adjustments like the Bonferroni correction might be necessary to control for this.
Practical Examples
Let's illustrate finding the p-value with a couple of examples:
Example 1: Goodness-of-Fit Test
A company claims that its bags of candy contain 40% red, 30% blue, and 30% green candies. You sample a bag and observe the following counts: Red: 80, Blue: 60, Green: 60. Is the company's claim accurate?
-
Calculate expected frequencies: Assume a sample size of 200 (80+60+60). Expected frequencies are: Red: 80, Blue: 60, Green: 60.
-
Calculate the chi-square statistic: Using the formula, you'd get a chi-square value.
-
Determine degrees of freedom: df = 3 - 1 = 2
-
Find the p-value: Using statistical software or an online calculator with the chi-square statistic and df=2, you'll obtain a p-value. If the p-value is greater than 0.05, you fail to reject the null hypothesis (the company's claim is plausible). If it's less than 0.05, you reject the null hypothesis (the company's claim is likely inaccurate).
Example 2: Test of Independence
You want to investigate whether there's an association between gender and preference for coffee or tea. You collect data from 100 individuals:
Coffee | Tea | Total | |
---|---|---|---|
Male | 30 | 20 | 50 |
Female | 40 | 10 | 50 |
Total | 70 | 30 | 100 |
-
Calculate expected frequencies: For example, the expected frequency of males preferring coffee is (50 * 70) / 100 = 35.
-
Calculate the chi-square statistic: Use the formula, considering all four cells.
-
Determine degrees of freedom: df = (2 - 1) * (2 - 1) = 1
-
Find the p-value: Using statistical software or an online calculator, determine the p-value. A p-value less than 0.05 would suggest a significant association between gender and beverage preference.
Conclusion
Finding the p-value for a chi-square test is a critical step in analyzing categorical data. By understanding the different types of chi-square tests, calculating the chi-square statistic, and employing appropriate methods to determine the p-value, you can draw meaningful conclusions from your data. Remember to always consider the context of your findings, the assumptions of the test, and the practical significance of your results. Utilizing statistical software is highly recommended for accurate and efficient p-value calculation. This guide provides a solid foundation for mastering this essential statistical technique.
Latest Posts
Latest Posts
-
Greatest Common Factor Of 18 And 20
May 14, 2025
-
How Many Seconds In 50 Years
May 14, 2025
-
What Year Was 53 Years Ago
May 14, 2025
-
What Is A 3 Out Of 7
May 14, 2025
-
1 Bar Is How Much Psi
May 14, 2025
Related Post
Thank you for visiting our website which covers about Find P Value For Chi Square . We hope the information provided has been useful to you. Feel free to contact us if you have any questions or need further assistance. See you next time and don't miss to bookmark.