Find The Best Point Estimate Of The Population Proportion P
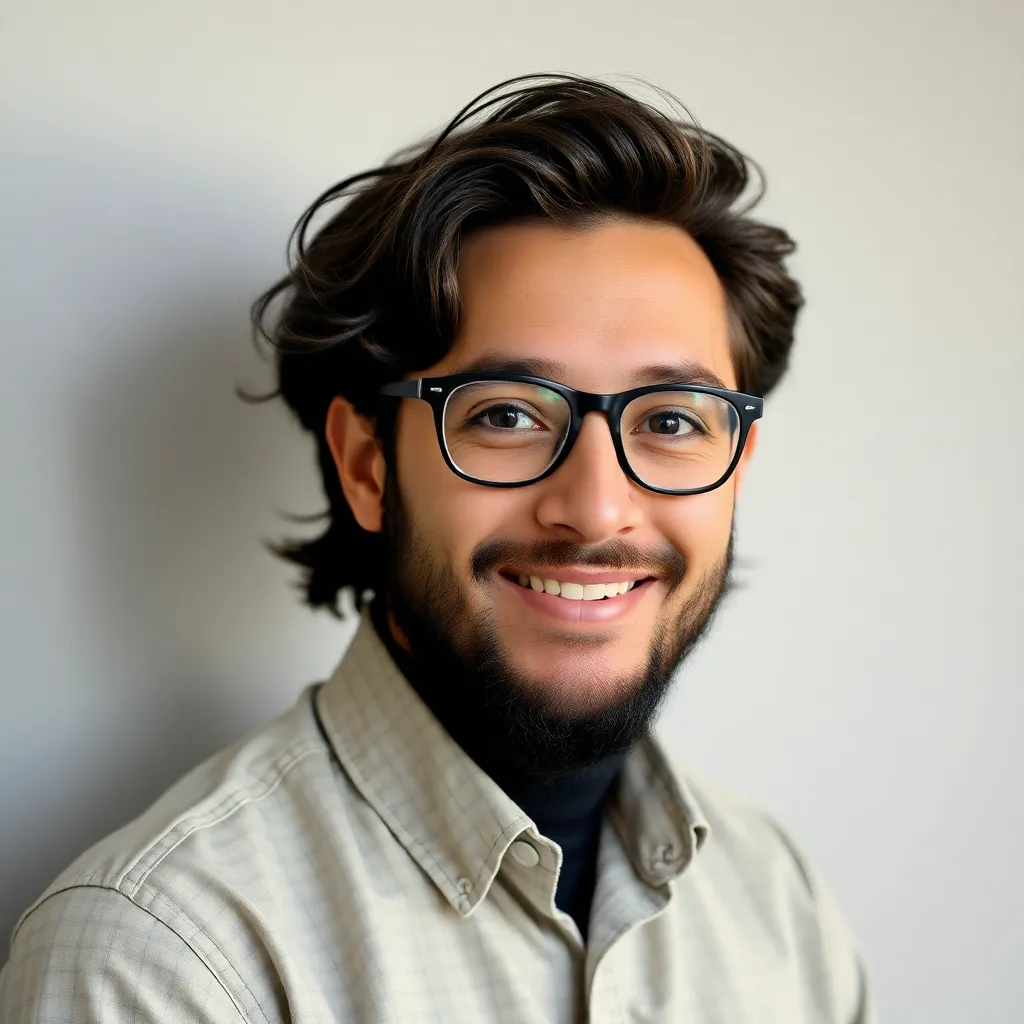
Treneri
May 12, 2025 · 5 min read
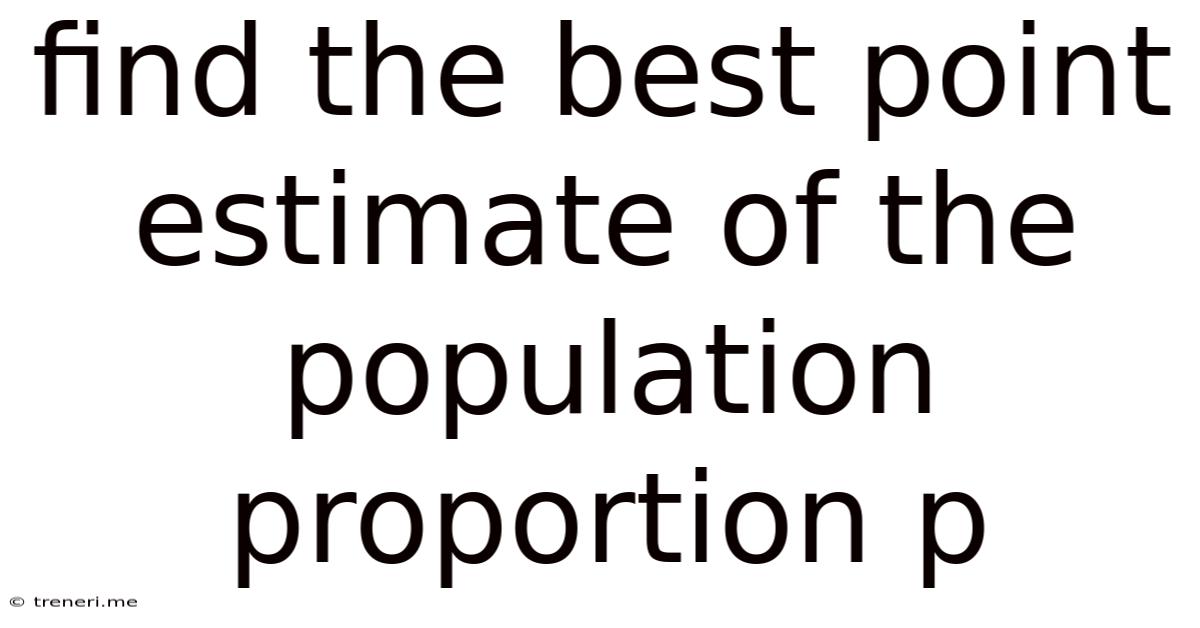
Table of Contents
Finding the Best Point Estimate of the Population Proportion (p)
Estimating population parameters from sample data is a cornerstone of statistical inference. One crucial parameter is the population proportion, denoted by 'p', which represents the fraction of individuals in a population possessing a specific characteristic. Since examining an entire population is often impractical or impossible, we rely on sample data to estimate this proportion. This article delves into the methods for finding the best point estimate of the population proportion, exploring its properties, limitations, and practical applications.
Understanding Point Estimation
Before diving into the specifics of estimating 'p', let's clarify the concept of point estimation. Point estimation involves using a single value calculated from sample data to estimate an unknown population parameter. In our case, the parameter is 'p', and the point estimate will be a single number representing our "best guess" of its value. This is different from interval estimation, which provides a range of plausible values for the parameter.
The Sample Proportion: Our Best Point Estimate
The most commonly used and statistically sound point estimate for the population proportion 'p' is the sample proportion, denoted by 'p̂' (pronounced "p-hat"). The sample proportion is simply the number of individuals in the sample possessing the characteristic of interest, divided by the total sample size.
Formula:
p̂ = x / n
Where:
x
is the number of individuals in the sample with the characteristic.n
is the total sample size.
For example, if we survey 100 people (n=100) and find that 30 of them (x=30) prefer a particular brand of coffee, our sample proportion is:
p̂ = 30 / 100 = 0.3
This means our best point estimate for the population proportion of people preferring that coffee brand is 0.3, or 30%.
Properties of a Good Estimator
A good point estimator should possess several desirable properties:
-
Unbiasedness: An unbiased estimator's expected value is equal to the true population parameter. In simpler terms, if we were to repeatedly take samples and calculate the sample proportion each time, the average of all those sample proportions would converge to the true population proportion. The sample proportion, p̂, is an unbiased estimator of p.
-
Consistency: As the sample size increases, the estimator converges towards the true population parameter. This means that with larger samples, our estimate becomes increasingly accurate. The sample proportion is a consistent estimator.
-
Efficiency: An efficient estimator has a smaller variance (less variability) compared to other estimators. This means that the sample proportion tends to be closer to the true population proportion than other estimators, given the same sample size. While there might be other estimators, p̂ is generally considered efficient among unbiased estimators.
-
Sufficiency: A sufficient estimator captures all the information about the parameter contained in the sample. The sample proportion is a sufficient estimator.
Sampling Distribution of the Sample Proportion
Understanding the sampling distribution of the sample proportion is crucial for assessing the reliability of our point estimate. The sampling distribution describes the probability distribution of all possible sample proportions we could obtain from repeated sampling.
Under certain conditions (a large enough sample size and the independence of observations), the sampling distribution of p̂ is approximately normally distributed with:
-
Mean (μ<sub>p̂</sub>) = p: The expected value of the sample proportion is equal to the true population proportion.
-
Standard Deviation (σ<sub>p̂</sub>) = √[p(1-p) / n]: This is also known as the standard error of the proportion. It quantifies the variability of the sample proportion. Notice that the standard error decreases as the sample size (n) increases.
Determining Sufficient Sample Size
The accuracy of our point estimate depends heavily on the sample size. A larger sample size generally leads to a more accurate estimate because it reduces the standard error. Determining the appropriate sample size is crucial before conducting any survey or study. The required sample size depends on:
-
Desired Margin of Error: How much error are you willing to tolerate in your estimate? A smaller margin of error requires a larger sample size.
-
Confidence Level: How confident do you want to be that your interval estimate contains the true population proportion? Higher confidence levels require larger sample sizes.
-
Estimated Population Proportion (p): If you have a prior estimate of p, this can be used to calculate the required sample size. If not, you can use a conservative estimate of 0.5 (which maximizes the standard error).
There are formulas to calculate the required sample size based on these factors. These calculations often involve the use of the z-score associated with the desired confidence level (e.g., 1.96 for a 95% confidence level).
Limitations and Considerations
While the sample proportion is a powerful and widely used estimator, it's essential to acknowledge its limitations:
-
Sampling Bias: If the sample is not representative of the population (e.g., due to selection bias or non-response bias), the sample proportion will not be a reliable estimate of the population proportion.
-
Finite Population Correction: The formula for the standard error assumes an infinitely large population. For smaller populations, a finite population correction factor should be applied to adjust the standard error.
-
Non-Random Sampling: The assumption of random sampling is crucial. If the sampling method is not random, the properties of the estimator may not hold.
-
Data Quality: The accuracy of the estimate relies heavily on the quality of the data. Errors in data collection or recording can lead to inaccurate estimates.
Practical Applications
Estimating the population proportion finds applications in numerous fields:
-
Market Research: Estimating the proportion of consumers who prefer a particular product.
-
Public Opinion Polls: Estimating the proportion of voters who support a particular candidate.
-
Medical Research: Estimating the proportion of patients who respond positively to a new treatment.
-
Quality Control: Estimating the proportion of defective items in a production batch.
-
Environmental Studies: Estimating the proportion of a population affected by pollution.
Conclusion
The sample proportion (p̂) serves as the best point estimate for the population proportion (p) under many circumstances. Its unbiasedness, consistency, efficiency, and sufficiency make it a statistically robust estimator. However, careful attention to sampling methods, sample size determination, and potential biases is crucial for obtaining reliable and accurate estimates. Remember to consider the limitations and context of your data to ensure your estimations are meaningful and valid. Understanding the sampling distribution and applying the appropriate formulas are essential steps in accurately estimating the population proportion. By carefully considering these factors, researchers and analysts can use sample data to confidently estimate population proportions in diverse areas of application.
Latest Posts
Latest Posts
-
114 Divided By 19 With Remainder
May 12, 2025
-
What Is The Gcf Of 12 And 27
May 12, 2025
-
What Is 9 10 As A Grade
May 12, 2025
-
How Many Days Left Until Christmas 2024
May 12, 2025
-
Least Common Multiple Of 11 And 10
May 12, 2025
Related Post
Thank you for visiting our website which covers about Find The Best Point Estimate Of The Population Proportion P . We hope the information provided has been useful to you. Feel free to contact us if you have any questions or need further assistance. See you next time and don't miss to bookmark.