How To Find A Point Estimate
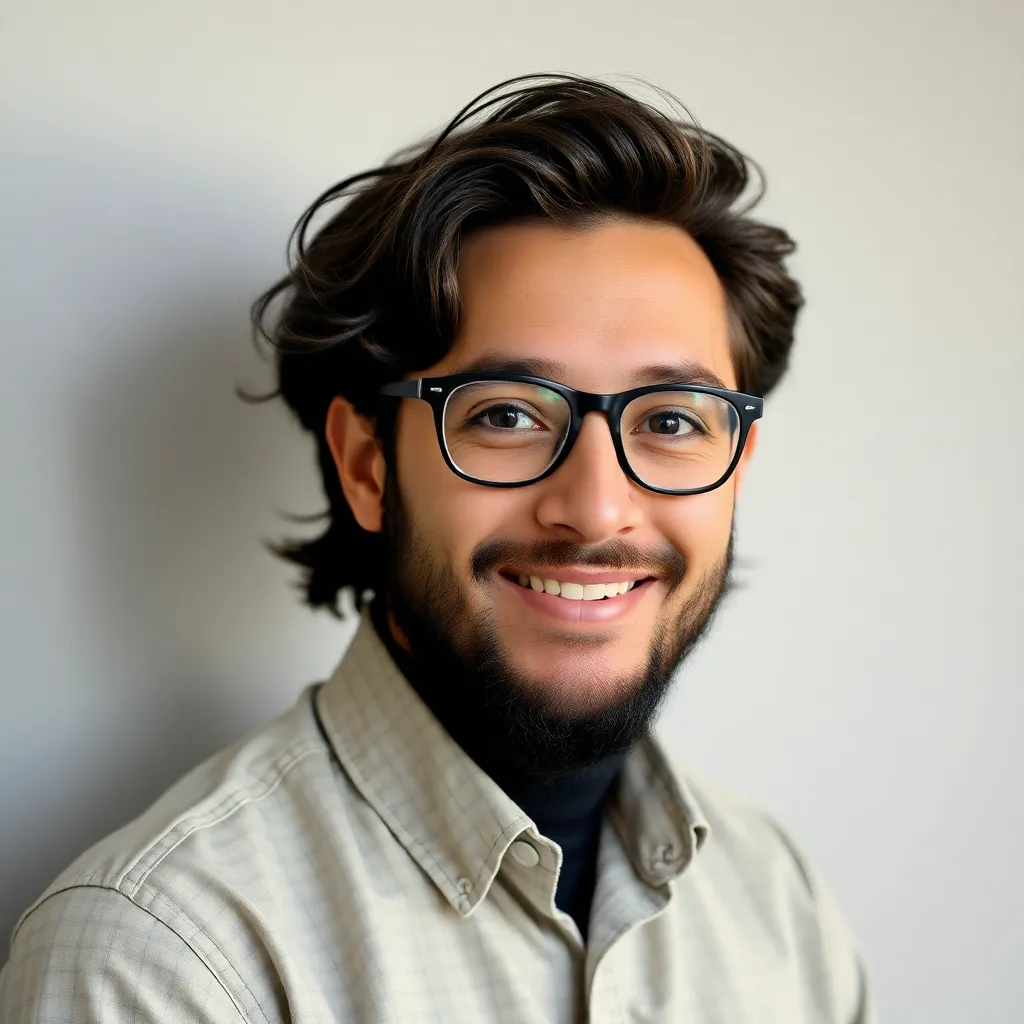
Treneri
Apr 06, 2025 · 6 min read

Table of Contents
- How To Find A Point Estimate
- Table of Contents
- How to Find a Point Estimate: A Comprehensive Guide
- Understanding Point Estimates: The Core Concept
- Key Population Parameters and Their Corresponding Point Estimates
- 1. Population Mean (μ):
- 2. Population Proportion (p):
- 3. Population Variance (σ²) and Population Standard Deviation (σ):
- 4. Population Median:
- 5. Population Correlation Coefficient (ρ):
- Choosing the Right Point Estimate: Considerations and Context
- Beyond Point Estimates: Confidence Intervals
- Practical Examples: Illustrating Point Estimation
- Conclusion: Accuracy, Precision, and Best Practices
- Latest Posts
- Latest Posts
- Related Post
How to Find a Point Estimate: A Comprehensive Guide
Finding the right point estimate is crucial in statistical inference. It's the cornerstone of making informed decisions based on data, whether you're analyzing market trends, conducting medical research, or evaluating the effectiveness of a new marketing campaign. This comprehensive guide delves deep into the process, exploring various methods and offering practical examples to solidify your understanding.
Understanding Point Estimates: The Core Concept
A point estimate is a single value used to estimate an unknown population parameter. Think of it as a "best guess" based on the available sample data. Instead of dealing with the entire population (which is often impossible or impractical), we use a sample to infer properties about the whole. This "best guess" is the point estimate.
For instance, if you want to know the average height of all adult women in a country, you wouldn't measure every single woman. Instead, you'd take a representative sample and calculate the average height of that sample. That average height would be your point estimate for the average height of the entire population.
The accuracy of your point estimate depends heavily on the sample size and how representative your sample is of the overall population. A larger, more representative sample generally leads to a more accurate estimate.
Key Population Parameters and Their Corresponding Point Estimates
Several key population parameters are commonly estimated using point estimates. Here are some of the most prevalent ones:
1. Population Mean (μ):
The population mean represents the average value of a variable across the entire population. Its point estimate is the sample mean (x̄), calculated by summing all values in the sample and dividing by the sample size (n):
x̄ = Σxᵢ / n
Where:
- x̄ is the sample mean
- Σxᵢ is the sum of all values in the sample
- n is the sample size
Example: If you collect the heights of 100 women and their average height is 5'4", then 5'4" is your point estimate for the average height of all women in the population.
2. Population Proportion (p):
The population proportion represents the fraction of the population possessing a specific characteristic. Its point estimate is the sample proportion (p̂), calculated by dividing the number of individuals in the sample with the characteristic by the sample size:
p̂ = x / n
Where:
- p̂ is the sample proportion
- x is the number of individuals in the sample with the characteristic
- n is the sample size
Example: If you survey 500 people and 200 of them prefer brand A, then 200/500 = 0.4 is your point estimate for the proportion of the population who prefer brand A.
3. Population Variance (σ²) and Population Standard Deviation (σ):
The population variance measures the spread or dispersion of the data around the population mean. The population standard deviation is the square root of the variance. Their point estimates are the sample variance (s²) and the sample standard deviation (s), respectively:
s² = Σ(xᵢ - x̄)² / (n - 1)
s = √s²
Where:
- s² is the sample variance
- s is the sample standard deviation
- xᵢ is each individual value in the sample
- x̄ is the sample mean
- n is the sample size
Note the use of (n-1) in the denominator of the sample variance calculation. This is known as Bessel's correction, which provides an unbiased estimate of the population variance. Using 'n' would underestimate the population variance.
Example: Calculating the variance and standard deviation from a sample of test scores gives you point estimates of the population variance and standard deviation of test scores.
4. Population Median:
The population median is the middle value when the data is ordered. The point estimate is the sample median, which is found by arranging the sample data in ascending order and identifying the middle value (or the average of the two middle values if the sample size is even).
5. Population Correlation Coefficient (ρ):
The population correlation coefficient measures the linear relationship between two variables in the population. The point estimate is the sample correlation coefficient (r), which is calculated using various methods, typically involving the covariance and standard deviations of the two variables in the sample.
Choosing the Right Point Estimate: Considerations and Context
Selecting the appropriate point estimate depends heavily on the nature of your data and the research question. Here are some key considerations:
-
Data Type: The type of data (continuous, discrete, categorical) dictates the relevant point estimates. For example, the mean is suitable for continuous data, while the proportion is appropriate for categorical data.
-
Distribution: If your data is normally distributed, the mean is often a robust point estimate. However, for skewed distributions, the median might be a more representative measure of central tendency.
-
Research Question: The specific question you are trying to answer will guide your choice of parameter and, consequently, the point estimate.
-
Sample Size: A larger sample generally leads to a more accurate point estimate.
-
Outliers: Outliers can significantly impact the mean. If outliers are present, the median might be a more robust point estimate.
Beyond Point Estimates: Confidence Intervals
While point estimates provide a single value, they lack information about the uncertainty associated with the estimate. This uncertainty arises because the point estimate is based on a sample, not the entire population. Therefore, it's crucial to complement point estimates with confidence intervals.
A confidence interval provides a range of plausible values for the population parameter, along with a specified level of confidence (e.g., 95%). A 95% confidence interval means that if you were to repeat the sampling process many times, 95% of the resulting confidence intervals would contain the true population parameter.
Confidence intervals are calculated using the point estimate, the standard error of the estimate, and the critical value from the appropriate probability distribution (e.g., t-distribution or z-distribution).
Practical Examples: Illustrating Point Estimation
Let's illustrate point estimation with a couple of detailed examples:
Example 1: Estimating the Average Income of Households
Suppose you want to estimate the average annual income of households in a city. You collect data from a random sample of 100 households, obtaining the following sample statistics:
- Sample mean (x̄) = $65,000
- Sample standard deviation (s) = $15,000
Your point estimate for the average annual income of households in the city is $65,000. This is a single value representing your best guess based on the sample. However, you would likely also calculate a confidence interval to account for sampling error and provide a range of plausible values for the true population mean.
Example 2: Estimating the Proportion of Voters Favoring a Candidate
Imagine you're conducting a poll to estimate the proportion of voters who favor a particular candidate in an upcoming election. You survey 500 randomly selected voters, and 275 indicate they support the candidate.
Your point estimate for the proportion of voters favoring the candidate is 275/500 = 0.55 or 55%. Again, a confidence interval should accompany this point estimate to provide a more complete picture, quantifying the uncertainty associated with the estimate.
Conclusion: Accuracy, Precision, and Best Practices
The accuracy and precision of your point estimate depend on factors like sample size, sampling method, and the variability within the population. Employing robust sampling techniques and using appropriate statistical methods is crucial to minimize bias and improve the reliability of your point estimates. Always remember that a point estimate is just a single value, and a confidence interval provides a more complete and informative picture by incorporating the inherent uncertainty associated with estimating population parameters from sample data. Finally, clearly communicate the limitations of your point estimate alongside its value within the context of your research.
Latest Posts
Latest Posts
-
How To Calculate Days Into Months
Apr 12, 2025
-
How Many Drops In 5ml Bottle
Apr 12, 2025
-
Tablespoon Minced Garlic To Garlic Powder
Apr 12, 2025
-
How Much Is 40ml Of Water
Apr 12, 2025
-
Cuanto Es El 10 Por Ciento De 10000
Apr 12, 2025
Related Post
Thank you for visiting our website which covers about How To Find A Point Estimate . We hope the information provided has been useful to you. Feel free to contact us if you have any questions or need further assistance. See you next time and don't miss to bookmark.