How To Find Point Estimate Of Population Proportion
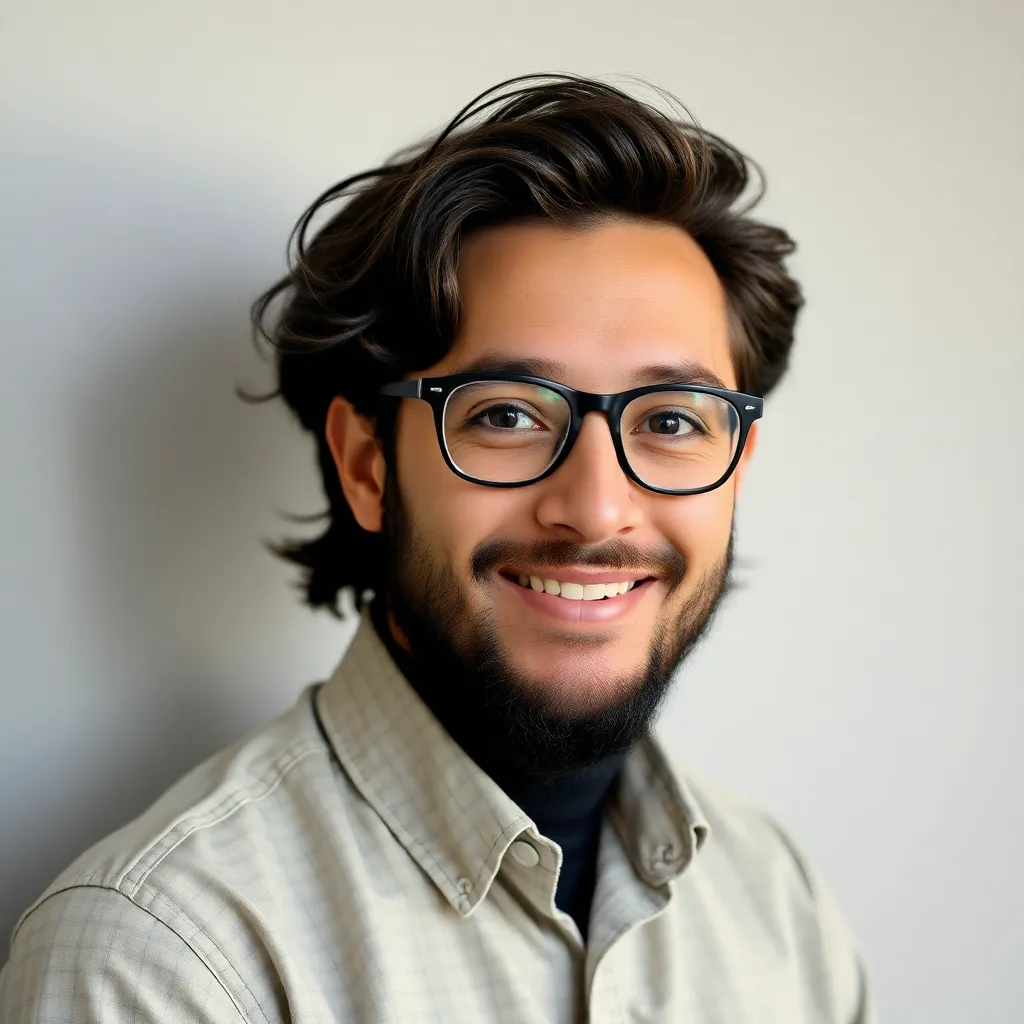
Treneri
May 11, 2025 · 6 min read

Table of Contents
How to Find the Point Estimate of a Population Proportion
Understanding and calculating the point estimate of a population proportion is crucial in statistics. It allows researchers and analysts to make inferences about a larger population based on a smaller sample. This comprehensive guide will delve into the intricacies of finding this key statistical measure, covering everything from defining the concept to tackling practical examples and potential pitfalls. We'll also explore the relationship between sample size and the accuracy of your point estimate.
What is a Point Estimate?
A point estimate is a single value that serves as the "best guess" for an unknown population parameter. In the context of population proportions, this parameter represents the true proportion of individuals in the population possessing a specific characteristic. For example, if we're interested in the proportion of voters who favor a particular candidate, the population proportion (denoted as p) represents the true percentage of all voters who support that candidate. Since surveying every single voter is often impractical, we rely on a sample to estimate this unknown p.
The point estimate of the population proportion, denoted as p̂ (p-hat), is calculated from a sample and serves as our best guess for the true population proportion. It's important to remember that this is just an estimate; it's unlikely to be exactly equal to the true population proportion, but a well-drawn sample will give us a reasonably close approximation.
How to Calculate the Point Estimate of a Population Proportion
The calculation itself is straightforward. The point estimate is simply the proportion of individuals in your sample who possess the characteristic of interest. The formula is:
p̂ = x / n
Where:
- p̂ is the sample proportion (point estimate)
- x is the number of individuals in the sample with the characteristic of interest
- n is the total number of individuals in the sample
Let's illustrate with an example:
Example 1: Customer Satisfaction
A company surveys 200 customers (n = 200) regarding their satisfaction with a new product. 150 customers (x = 150) report being satisfied. The point estimate of the population proportion of satisfied customers is:
p̂ = 150 / 200 = 0.75 or 75%
This means that based on the sample, the company estimates that 75% of all customers are satisfied with the new product.
Understanding Sampling Error and Confidence Intervals
It's crucial to understand that the sample proportion (p̂) is unlikely to be exactly equal to the true population proportion (p). This difference is called sampling error. Sampling error arises because we're only looking at a subset of the population, not the entire population itself. Larger sample sizes generally lead to smaller sampling errors.
To account for sampling error and provide a range of plausible values for the population proportion, we use confidence intervals. A confidence interval provides an upper and lower bound within which the true population proportion is likely to fall, with a specified level of confidence (e.g., 95% or 99%). The formula for a confidence interval is:
p̂ ± Z * √[(p̂(1-p̂))/n]
Where:
- p̂ is the sample proportion
- Z is the Z-score corresponding to the desired confidence level (e.g., 1.96 for a 95% confidence level)
- n is the sample size
Example 2: Calculating a Confidence Interval
Using the customer satisfaction example above (p̂ = 0.75, n = 200), let's calculate a 95% confidence interval:
-
Find the Z-score: For a 95% confidence level, the Z-score is approximately 1.96.
-
Calculate the margin of error: Margin of error = 1.96 * √[(0.75 * 0.25) / 200] ≈ 0.067
-
Calculate the confidence interval: 0.75 ± 0.067 This gives us a 95% confidence interval of (0.683, 0.817), or (68.3%, 81.7%).
This means we are 95% confident that the true proportion of satisfied customers lies between 68.3% and 81.7%.
Factors Affecting the Accuracy of the Point Estimate
Several factors influence the accuracy of the point estimate:
-
Sample Size: Larger samples generally lead to more accurate point estimates because they reduce sampling error. The relationship is not linear, but the increase in accuracy diminishes with larger and larger sample sizes.
-
Sampling Method: The way the sample is selected is crucial. A random sample is essential to ensure that the sample is representative of the population. Biased sampling methods can lead to inaccurate point estimates.
-
Population Variability: If the characteristic of interest is highly variable within the population, a larger sample size may be needed to achieve a given level of accuracy.
-
Confidence Level: A higher confidence level (e.g., 99% vs. 95%) requires a wider confidence interval, which means less precision in the point estimate. This is a trade-off between confidence and precision.
Choosing the Right Sample Size
Determining the appropriate sample size is a critical step in ensuring the accuracy of your point estimate. The required sample size depends on several factors including the desired margin of error, the confidence level, and an estimated population proportion (often a conservative estimate of 0.5 is used if no prior information is available). There are formulas to calculate the necessary sample size, but they are beyond the scope of this introductory explanation. Specialized statistical software or online calculators can assist with sample size determination.
Avoiding Common Pitfalls
-
Biased Sampling: Carefully consider your sampling method to avoid bias. Non-random sampling techniques can lead to significantly skewed results and inaccurate point estimates.
-
Misinterpreting Confidence Intervals: A confidence interval does not mean there's a 95% chance the true population proportion falls within the calculated range. The confidence level refers to the long-run reliability of the procedure used to construct the interval. If we were to repeat the sampling and estimation process many times, 95% of the resulting confidence intervals would contain the true population proportion.
-
Ignoring Margin of Error: Always consider the margin of error when interpreting the point estimate. A point estimate alone is insufficient; it must be accompanied by a measure of uncertainty like a confidence interval.
-
Using Point Estimates for Small Samples: When dealing with small samples, the point estimate may not be as reliable, and the use of exact methods (like the Clopper-Pearson interval) for calculating confidence intervals might be more appropriate. The standard formula for the confidence interval may not hold up well in cases where the expected number of successes or failures is too small (generally, less than 5).
Conclusion
The point estimate of a population proportion is a fundamental concept in statistical inference. It provides a valuable, albeit imperfect, summary of the unknown population parameter. By understanding the calculation, interpreting confidence intervals, and carefully considering potential sources of error, researchers and analysts can make more informed decisions based on sample data. Remember that the accuracy of your point estimate is directly linked to the careful planning and execution of your sampling procedure and a thorough consideration of the limitations of your analysis. Always strive for representative samples and appropriately interpret the results in light of the inherent uncertainty involved.
Latest Posts
Latest Posts
-
Convert The Weight Of 49 Ounces To Grams
May 13, 2025
-
Which Ratios Have A Unit Rate Of 3
May 13, 2025
-
60 Days From June 18th 2024
May 13, 2025
-
Can U Get Tan In Uv 6
May 13, 2025
-
How To Find The Volume Of A Slanted Cylinder
May 13, 2025
Related Post
Thank you for visiting our website which covers about How To Find Point Estimate Of Population Proportion . We hope the information provided has been useful to you. Feel free to contact us if you have any questions or need further assistance. See you next time and don't miss to bookmark.