How To Find Standard Deviation Of A Sampling Distribution
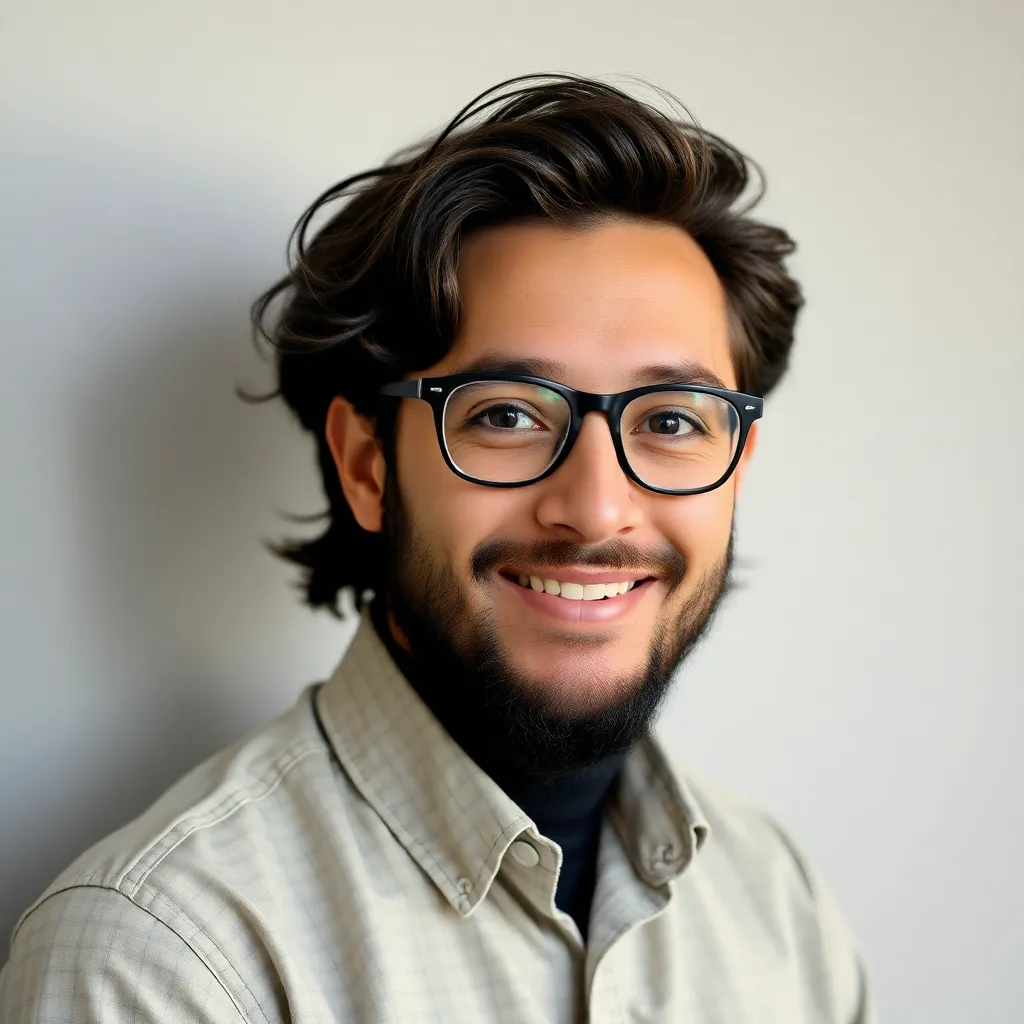
Treneri
May 11, 2025 · 6 min read
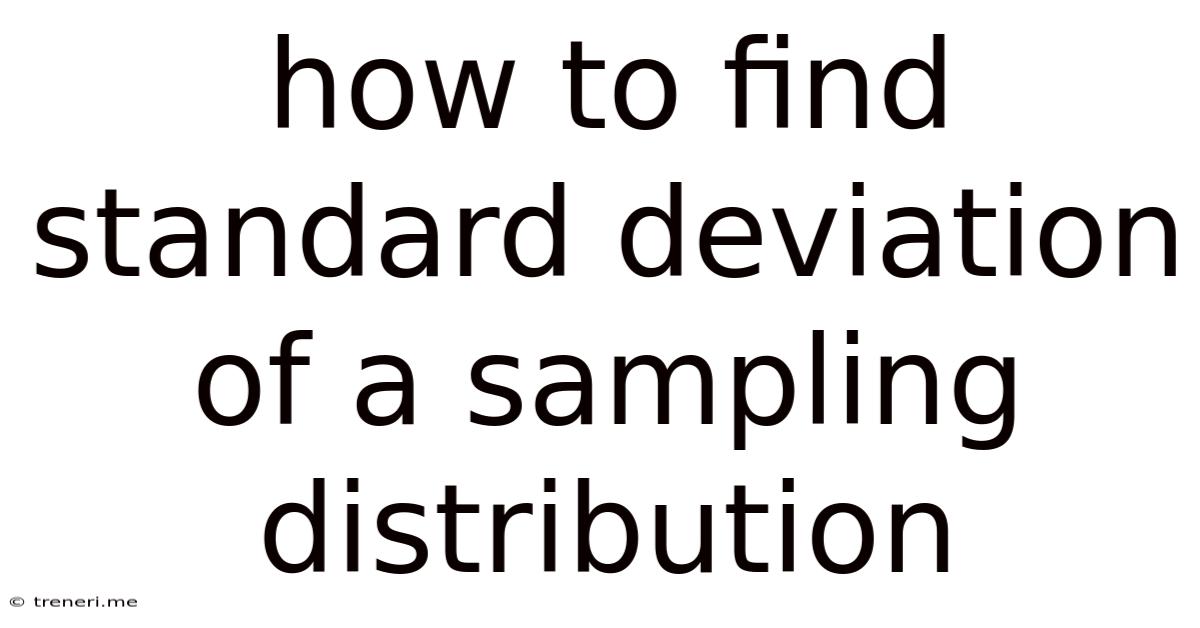
Table of Contents
How to Find the Standard Deviation of a Sampling Distribution (Standard Error)
Understanding the standard deviation of a sampling distribution, also known as the standard error, is crucial for statistical inference. It quantifies the variability between sample means (or other sample statistics) and the true population mean. A smaller standard error indicates that the sample means are clustered tightly around the population mean, suggesting greater precision in your estimations. Conversely, a larger standard error implies more variability, leading to less precise estimations. This article will thoroughly guide you through the process of calculating the standard error, exploring different scenarios and providing practical examples.
Understanding Sampling Distributions
Before diving into calculating the standard error, let's solidify our understanding of sampling distributions. A sampling distribution is the probability distribution of a statistic (like the sample mean, sample proportion, etc.) obtained from a large number of samples drawn from the same population. It's not the distribution of the population itself, but rather the distribution of the statistics calculated from those samples.
Imagine repeatedly drawing samples of a certain size (n) from a population, calculating the mean of each sample, and then plotting those means. The resulting distribution is the sampling distribution of the mean. This distribution will usually be approximately normal, regardless of the shape of the original population distribution, thanks to the Central Limit Theorem (CLT). The CLT states that the sampling distribution of the mean approaches a normal distribution as the sample size (n) increases, provided the population has a finite variance.
Calculating the Standard Error of the Mean
The standard error of the mean (SEM) is the most common type of standard error. It represents the standard deviation of the sampling distribution of the sample means. The formula for the SEM is:
SEM = σ / √n
Where:
- σ (sigma) is the population standard deviation.
- n is the sample size.
When the Population Standard Deviation (σ) is Known
If you know the population standard deviation, calculating the SEM is straightforward. Simply plug the values into the formula. For example:
Let's say we have a population with a known standard deviation (σ) of 10, and we're drawing samples of size (n) 25. The SEM would be:
SEM = 10 / √25 = 10 / 5 = 2
This means the standard deviation of the sampling distribution of the means is 2. Sample means will, on average, be within 2 units of the true population mean.
When the Population Standard Deviation (σ) is Unknown
More often, the population standard deviation is unknown. In this case, we estimate it using the sample standard deviation (s). The formula for the SEM then becomes:
SEM = s / √n
Where:
- s is the sample standard deviation.
- n is the sample size.
The use of 's' instead of 'σ' introduces some uncertainty, particularly with small sample sizes. For larger samples (generally n ≥ 30), the difference is usually negligible. However, for smaller samples, the t-distribution should be used for hypothesis testing and confidence interval construction instead of the normal distribution.
Example:
Suppose we collect a sample of 16 data points and calculate the sample standard deviation (s) to be 4. The SEM would be:
SEM = 4 / √16 = 4 / 4 = 1
Standard Error of the Proportion
The standard error of the proportion (SEP) is used when dealing with proportions (p) rather than means. It's particularly relevant in contexts like opinion polls or quality control, where you're interested in the proportion of a population possessing a certain characteristic. The formula is:
SEP = √[p(1-p) / n]
Where:
- p is the sample proportion.
- n is the sample size.
Example:
Let's say you conduct a survey and find that 60% (p = 0.6) of 100 respondents (n = 100) favor a particular candidate. The SEP would be:
SEP = √[0.6(1-0.6) / 100] = √(0.24 / 100) ≈ 0.049
This indicates that the standard deviation of the sampling distribution of sample proportions is approximately 0.049.
Factors Affecting the Standard Error
Several factors influence the magnitude of the standard error:
-
Sample Size (n): As the sample size increases, the standard error decreases. Larger samples provide more precise estimates of the population parameter. This is because a larger sample is more likely to be representative of the entire population.
-
Population Standard Deviation (σ or s): A larger population standard deviation (or sample standard deviation) leads to a larger standard error. This is intuitive; greater variability in the population translates to greater variability in sample statistics.
-
Type of Statistic: The standard error's formula varies depending on the statistic you're interested in (mean, proportion, etc.).
Importance of Standard Error in Statistical Inference
The standard error is a fundamental concept in statistical inference because it plays a vital role in:
-
Confidence Intervals: Standard error is used to construct confidence intervals, which provide a range of plausible values for the population parameter. A smaller standard error leads to a narrower confidence interval, implying a more precise estimate.
-
Hypothesis Testing: The standard error is the denominator in the test statistic for many hypothesis tests (e.g., t-test, z-test). A smaller standard error increases the power of the test, making it more likely to detect a true effect.
-
Sample Size Determination: The standard error is used to determine the appropriate sample size needed for a study to achieve a desired level of precision. Researchers often aim for a specific margin of error, which directly relates to the standard error.
Interpreting the Standard Error
It's crucial to understand that the standard error doesn't represent the error in a single measurement. Instead, it quantifies the variability you would expect to see across many sample means (or other statistics). A small standard error suggests that the sample statistic is a reliable estimate of the population parameter, while a large standard error indicates more uncertainty.
Advanced Considerations: Central Limit Theorem and Non-Normal Distributions
The Central Limit Theorem (CLT) is a cornerstone of the standard error calculation, especially when dealing with sample means. The CLT asserts that the sampling distribution of the mean will be approximately normal, irrespective of the underlying population distribution's shape, provided the sample size is sufficiently large (usually considered n ≥ 30).
However, when dealing with small sample sizes and non-normal populations, the standard error calculation might require adjustments. In these scenarios, the t-distribution is frequently used instead of the normal distribution for hypothesis testing and confidence interval calculation. The t-distribution accounts for the extra uncertainty introduced by estimating the population standard deviation from the sample.
Conclusion
The standard error is a vital tool in statistics, helping us understand the variability inherent in sample data and making more informed inferences about populations. By grasping the concepts explained in this article and understanding the relevant formulas, you can confidently calculate and interpret the standard error, enhancing your ability to analyze data and draw meaningful conclusions. Remember to always consider the sample size, population variability, and the type of statistic you're working with to ensure accurate and reliable calculations. Further exploration into topics like confidence intervals and hypothesis testing will build on this foundation and allow for even more robust statistical analysis.
Latest Posts
Latest Posts
-
How Much Is A 70 Percent Grade
May 12, 2025
-
An Inch Of Rain Is Equivalent To How Much Snow
May 12, 2025
-
How Many Days Since July 8 2022
May 12, 2025
-
9 Is What Percent Of 21
May 12, 2025
-
What Is The Gcf Of 9 And 24
May 12, 2025
Related Post
Thank you for visiting our website which covers about How To Find Standard Deviation Of A Sampling Distribution . We hope the information provided has been useful to you. Feel free to contact us if you have any questions or need further assistance. See you next time and don't miss to bookmark.