How To Find Upper Control Limit
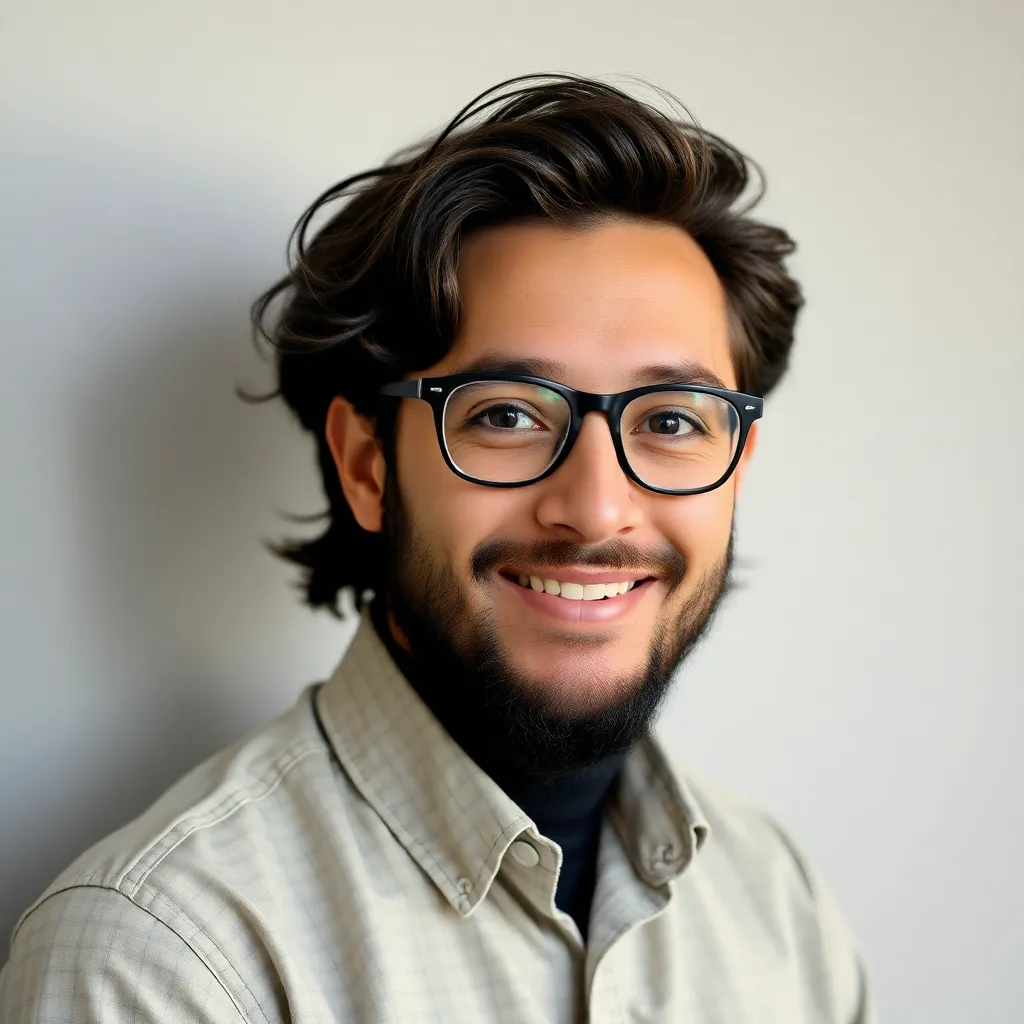
Treneri
Apr 10, 2025 · 6 min read

Table of Contents
How to Find the Upper Control Limit (UCL) in Statistical Process Control (SPC)
Statistical Process Control (SPC) is a powerful methodology used to monitor and improve processes by identifying and addressing sources of variation. A key component of SPC is the control chart, a graphical tool that displays data over time, allowing for the identification of trends and patterns. Central to understanding control charts is the concept of the Upper Control Limit (UCL), a crucial threshold indicating the acceptable upper bound of process variation. This comprehensive guide delves into the methods for calculating UCLs for various scenarios, explaining the underlying principles and practical applications.
Understanding Control Charts and the Upper Control Limit (UCL)
Control charts are designed to visually distinguish between common cause variation (inherent, random variation within a process) and special cause variation (assignable causes, indicating a problem needing attention). The UCL, along with the Lower Control Limit (LCL) and the Central Line (CL), forms the boundaries of what's considered statistically acceptable process variation. Data points falling outside these limits signal potential problems requiring investigation.
The UCL specifically represents the upper boundary. Any data point exceeding this limit suggests the process might be out of control, indicating that special cause variation is influencing the process. This could be due to factors such as faulty equipment, incorrect material, or operator errors. Identifying these causes is crucial for corrective action and process improvement.
Methods for Calculating the Upper Control Limit (UCL)
The method for calculating the UCL depends on the type of data being analyzed and the chosen control chart. The most common types are:
1. X-bar and R Charts (for continuous data)
These charts are used when monitoring the average (X-bar) and range (R) of a process. The UCL for both charts is calculated differently:
a) X-bar Chart (UCL for the average):
The formula for the UCL of an X-bar chart is:
UCL = X-double bar + A2 * R-bar
Where:
- X-double bar (X̄): The average of the subgroup averages. This is calculated by averaging all the subgroup means.
- A2: A constant factor dependent on the subgroup size (n). These values are available in statistical control chart tables.
- R-bar (R̄): The average range of the subgroups. This is the average of the ranges of all the subgroups. The range is the difference between the largest and smallest values within a subgroup.
b) R Chart (UCL for the range):
The formula for the UCL of an R chart is:
UCL = D4 * R-bar
Where:
- D4: A constant factor dependent on the subgroup size (n), also found in statistical control chart tables.
- R-bar (R̄): The average range of the subgroups, as defined above.
2. X-bar and s Charts (for continuous data, with a focus on standard deviation)
Similar to X-bar and R charts, but instead of using the range, these charts use the standard deviation (s) of each subgroup to measure variability.
a) X-bar Chart (UCL for the average):
UCL = X-double bar + A3 * s-bar
Where:
- X-double bar (X̄): The average of the subgroup averages.
- A3: A constant factor dependent on the subgroup size (n), obtained from statistical control chart tables.
- s-bar (s̄): The average standard deviation of the subgroups.
b) s Chart (UCL for the standard deviation):
UCL = B4 * s-bar
Where:
- B4: A constant factor dependent on the subgroup size (n), found in statistical control chart tables.
- s-bar (s̄): The average standard deviation of the subgroups.
3. p-Charts (for proportion data)
Used for monitoring the proportion of nonconforming units in a sample.
UCL = p-bar + 3 * sqrt(p-bar * (1 - p-bar) / n)
Where:
- p-bar (p̄): The average proportion of nonconforming units across all samples.
- n: The sample size.
4. c-Charts (for count data)
Used for monitoring the number of defects or occurrences in a sample of constant size.
UCL = c-bar + 3 * sqrt(c-bar)
Where:
- c-bar (c̄): The average number of defects or occurrences across all samples.
5. u-Charts (for count data with varying sample sizes)
Similar to c-charts, but used when sample sizes vary.
UCL = u-bar + 3 * sqrt(u-bar / n)
Where:
- u-bar (ū): The average number of defects per unit across all samples.
- n: The sample size.
Practical Considerations and Interpretation
-
Subgroup Size (n): The choice of subgroup size significantly impacts the calculation of UCL. Larger subgroups offer greater precision but might be less responsive to smaller shifts in the process. Smaller subgroups are more sensitive but can be more prone to random variation.
-
Data Distribution: The methods described above assume a normal distribution of the data. If the data deviates significantly from normality, transformations or alternative control chart methods might be necessary.
-
Choosing the Right Chart: Selecting the appropriate control chart is crucial for accurate analysis. The type of data (continuous, proportion, count) and the objective of the monitoring dictate the best chart to use.
-
Interpreting Results: When a data point exceeds the UCL, it doesn't automatically mean the process is broken. It triggers an investigation to identify the root cause of the deviation. It is essential to combine statistical analysis with process knowledge to understand the meaning of the results.
-
False Alarms: There's always a chance of a false alarm, where a data point exceeds the UCL due to random variation. This is why investigating the causes of out-of-control points is crucial, not just relying solely on the UCL value.
-
Software and Tools: Many statistical software packages (like Minitab, JMP, R) provide tools to easily calculate UCLs and generate control charts. These tools automate calculations, reduce error, and enhance the efficiency of the analysis.
Advanced Topics and Extensions
-
Using historical data: Sometimes, sufficient data to estimate the process parameters may not be readily available. Techniques for estimating the parameters from historical data or using prior knowledge exist, but their implementation requires a careful understanding of the process and statistical assumptions.
-
Time-Weighted Moving Average: This is a method of smoothing the data over time to reduce the impact of random fluctuations, potentially improving the identification of trends.
-
Exponentially Weighted Moving Average (EWMA): This approach assigns exponentially decreasing weights to older data points, giving more emphasis to recent observations. It can be more sensitive to smaller shifts in the process than traditional control charts.
Conclusion
The Upper Control Limit (UCL) is a fundamental component of Statistical Process Control, providing a vital threshold for identifying potential problems in a process. Understanding how to calculate and interpret the UCL for different types of data and control charts is essential for effective process monitoring and improvement. While the calculations might seem complex, leveraging the right tools and knowledge ensures accurate analysis, leading to better decision-making and enhanced operational efficiency. Remember that a thorough understanding of your process and the contextual interpretation of the statistical results are paramount to using UCLs effectively. By mastering these principles, you can unlock the full potential of SPC for optimizing your processes and achieving continuous improvement.
Latest Posts
Latest Posts
-
How To Compute Average Operating Assets
May 09, 2025
-
240 000 Rounded To The Nearest Hundred Thousand
May 09, 2025
-
What Percentage Is 10 Out Of 17
May 09, 2025
-
How To Find Z Score Without Standard Deviation
May 09, 2025
-
Microwave Conversion Chart 1100 To 1000
May 09, 2025
Related Post
Thank you for visiting our website which covers about How To Find Upper Control Limit . We hope the information provided has been useful to you. Feel free to contact us if you have any questions or need further assistance. See you next time and don't miss to bookmark.