Is Ml The Same As Cc
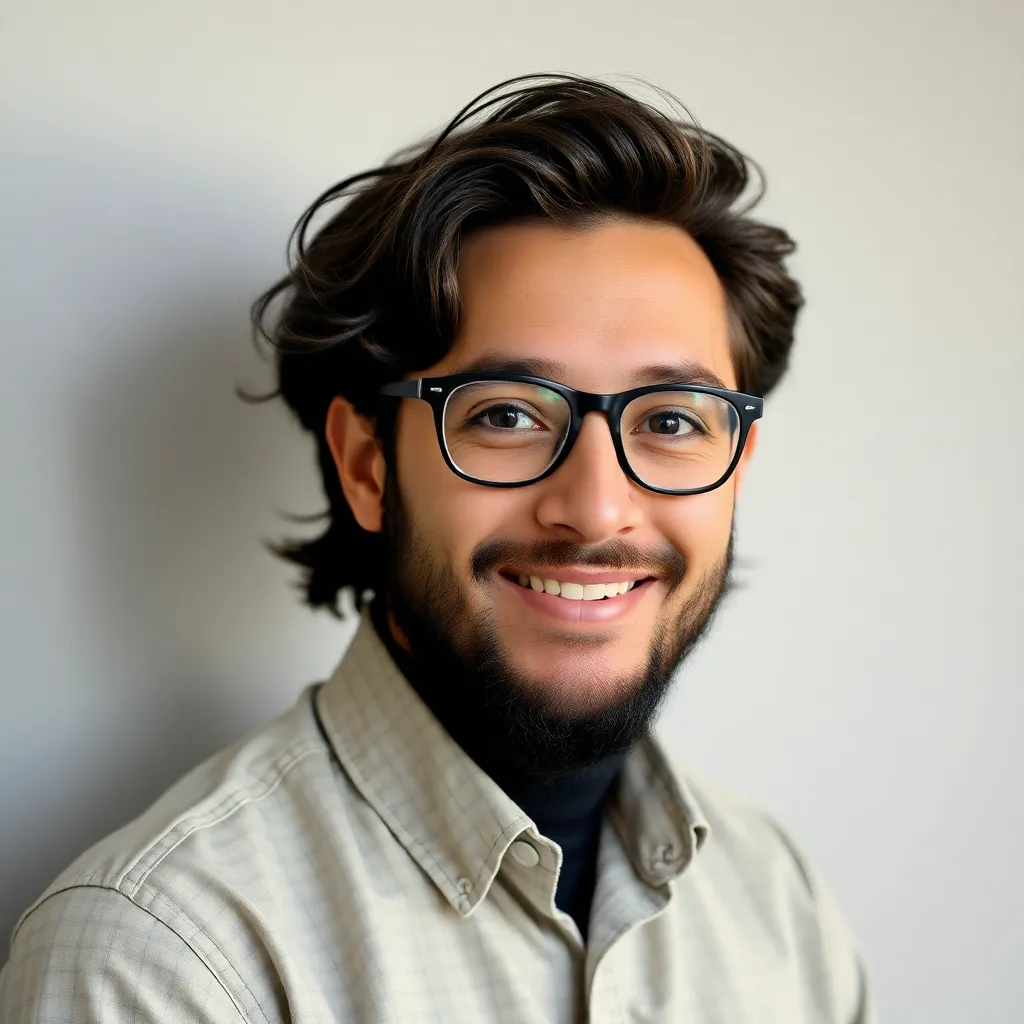
Treneri
Apr 09, 2025 · 5 min read

Table of Contents
Is ML the Same as CC? Understanding the Nuances of Machine Learning and Cloud Computing
The terms "machine learning" (ML) and "cloud computing" (CC) are frequently used in the tech world, often in the same breath. This often leads to confusion: are they the same thing? The short answer is no. While they are closely related and often work together, they are distinct concepts with separate functionalities and applications. This article will delve deep into the differences and similarities between ML and CC, exploring their individual strengths and how they synergistically power modern technological advancements.
What is Machine Learning (ML)?
Machine learning is a subfield of artificial intelligence (AI) that focuses on enabling computer systems to learn from data without being explicitly programmed. Instead of relying on pre-defined rules, ML algorithms identify patterns, make predictions, and improve their performance over time based on the data they are exposed to. This learning process involves several key components:
Key Components of Machine Learning:
- Data: The fuel for ML algorithms. The quality, quantity, and relevance of data significantly impact the accuracy and effectiveness of the model.
- Algorithms: The mathematical procedures that enable machines to learn from data. Different algorithms are suited for different types of learning tasks (e.g., classification, regression, clustering).
- Models: The output of the learning process, representing the knowledge extracted from the data. These models can be used to make predictions or decisions on new, unseen data.
- Evaluation: The process of assessing the performance of a model using metrics such as accuracy, precision, and recall. This helps to identify areas for improvement and fine-tune the model.
Types of Machine Learning:
- Supervised Learning: The algorithm learns from labeled data, where each data point is tagged with the correct output. Examples include image classification and spam detection.
- Unsupervised Learning: The algorithm learns from unlabeled data, identifying patterns and structures without explicit guidance. Examples include clustering and dimensionality reduction.
- Reinforcement Learning: The algorithm learns through trial and error, interacting with an environment and receiving rewards or penalties based on its actions. Examples include game playing and robotics.
What is Cloud Computing (CC)?
Cloud computing is a model for delivering IT services—including servers, storage, databases, networking, software, analytics, and intelligence—over the Internet ("the cloud"). Instead of owning and maintaining physical data centers and servers, users access these resources on demand from a cloud provider like Amazon Web Services (AWS), Microsoft Azure, or Google Cloud Platform (GCP).
Key Characteristics of Cloud Computing:
- On-demand self-service: Users can access computing resources as needed without requiring human interaction with each service provider.
- Broad network access: Resources are available via the internet and various devices.
- Resource pooling: The provider's computing resources are pooled to serve multiple users, with different physical and virtual resources dynamically assigned and reassigned according to user demand.
- Rapid elasticity: Resources can be rapidly and elastically provisioned and released, allowing for scaling up or down based on needs.
- Measured service: Cloud usage is monitored and reported, providing users with transparency and control over their costs.
Types of Cloud Computing Services:
- Infrastructure as a Service (IaaS): Provides basic computing resources like virtual machines, storage, and networking.
- Platform as a Service (PaaS): Offers a platform for developing, running, and managing applications without the need to manage the underlying infrastructure.
- Software as a Service (SaaS): Delivers software applications over the internet, eliminating the need for users to install or manage the software.
The Interplay Between ML and CC: A Powerful Synergy
While distinct, ML and CC are highly synergistic. Cloud computing provides the ideal infrastructure for machine learning, offering several key advantages:
How Cloud Computing Supports Machine Learning:
- Scalability and Elasticity: Cloud platforms enable ML models to scale effortlessly, handling massive datasets and high computational demands. This is crucial for training complex models and deploying them for real-time applications.
- Cost-Effectiveness: Cloud computing eliminates the need for expensive hardware investments, reducing the upfront cost of building and maintaining ML infrastructure. Users only pay for the resources they consume.
- Accessibility to Resources: Cloud providers offer a wide range of pre-trained models, libraries, and tools that simplify the development and deployment of ML applications. This reduces the technical barrier to entry for individuals and businesses.
- Data Storage and Management: Cloud storage solutions provide secure and scalable storage for large datasets, making data readily accessible to ML algorithms.
- High Performance Computing (HPC): Cloud platforms offer access to powerful GPUs and other specialized hardware, accelerating the training of complex ML models.
Examples of ML Applications Powered by the Cloud:
- Image Recognition: Cloud-based image recognition systems, powered by ML algorithms, are used in various applications, such as facial recognition, object detection, and medical image analysis.
- Natural Language Processing (NLP): Cloud platforms enable the development and deployment of NLP applications, including chatbots, machine translation, and sentiment analysis.
- Predictive Analytics: Cloud-based predictive analytics leverage ML algorithms to forecast future outcomes, helping businesses make data-driven decisions.
- Recommendation Systems: Cloud-based recommendation systems, powered by ML algorithms, are used by companies like Netflix and Amazon to personalize user experiences.
Key Differences Between ML and CC: A Comparative Overview
While they work well together, it's crucial to understand their core distinctions:
Feature | Machine Learning (ML) | Cloud Computing (CC) |
---|---|---|
Definition | A field of AI focusing on enabling computers to learn from data | A model for delivering IT services over the internet |
Focus | Algorithms, models, and data for learning and prediction | Infrastructure, platforms, and software delivery |
Output | Predictive models, classifications, insights from data | Computing resources, applications, and services |
Purpose | To build intelligent systems capable of learning | To provide on-demand access to IT resources |
Dependence | Can utilize CC for scalability and resource access | Independent of ML; ML can leverage CC for its advantages |
Conclusion: A Symbiotic Relationship
Machine learning and cloud computing are not the same, but their relationship is symbiotic. Cloud computing provides the infrastructure and resources that enable machine learning to flourish. The scalability, cost-effectiveness, and accessibility offered by the cloud are essential for developing and deploying powerful ML applications that drive innovation across various industries. Understanding the individual strengths and the synergistic relationship between ML and CC is crucial for anyone navigating the ever-evolving landscape of modern technology. As both fields continue to advance, we can expect even more powerful and transformative applications to emerge from their combined potential.
Latest Posts
Latest Posts
-
How To Tell How Big A Kitten Will Get
Apr 17, 2025
-
How Many Days Is 101 Hours
Apr 17, 2025
-
Cuanto Es 62 Kg En Libras
Apr 17, 2025
-
How Many Hours Is 25 Days
Apr 17, 2025
-
How To Calculate The Resistance Value
Apr 17, 2025
Related Post
Thank you for visiting our website which covers about Is Ml The Same As Cc . We hope the information provided has been useful to you. Feel free to contact us if you have any questions or need further assistance. See you next time and don't miss to bookmark.