Mean And Standard Deviation Of Sampling Distribution Calculator
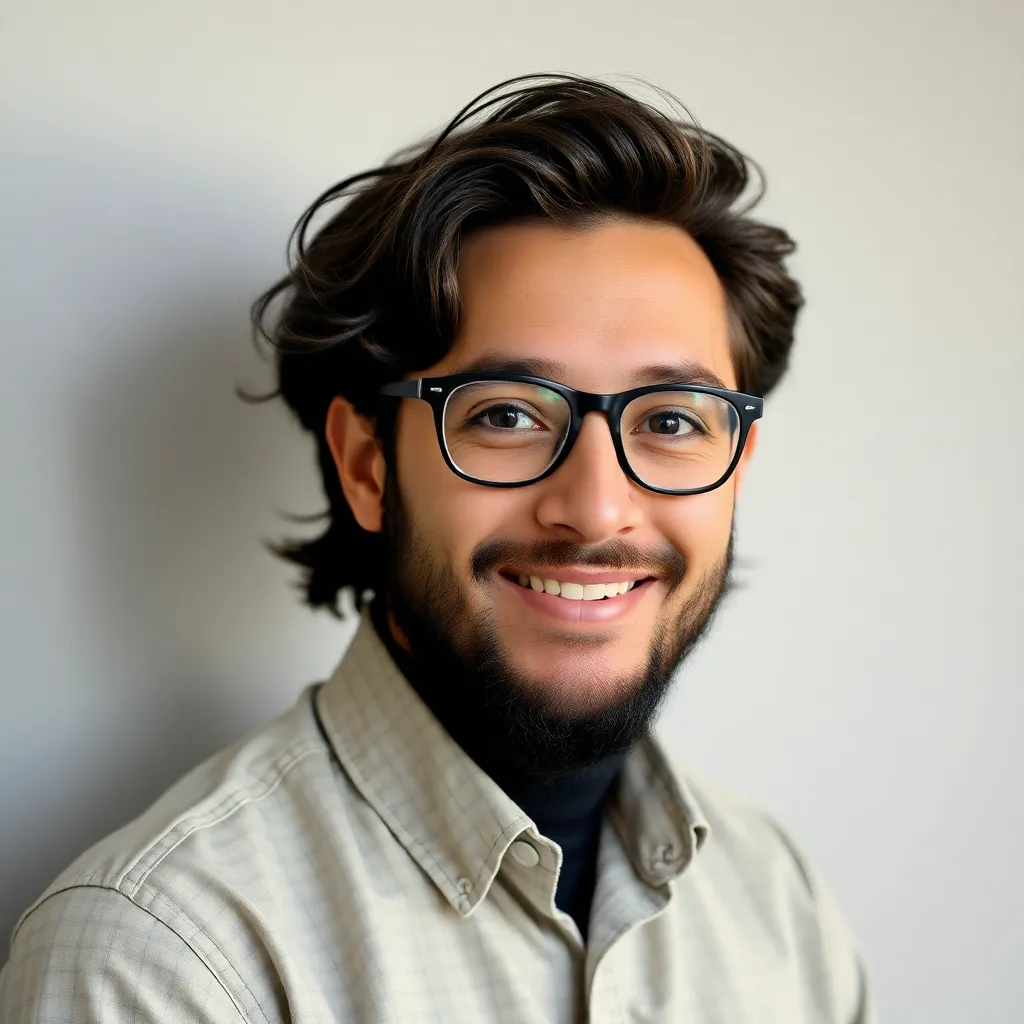
Treneri
Apr 04, 2025 · 6 min read
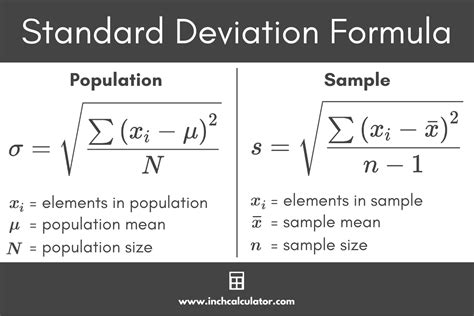
Table of Contents
Mean and Standard Deviation of Sampling Distribution Calculator: A Comprehensive Guide
Understanding the mean and standard deviation of a sampling distribution is crucial in statistics. These values help us infer properties about a population based on a sample, a fundamental concept in inferential statistics. This comprehensive guide delves into the intricacies of these concepts, explaining their meaning, calculation, and practical applications. We will also explore the utility of a sampling distribution calculator and how it simplifies the process.
What is a Sampling Distribution?
Before diving into the mean and standard deviation, let's establish a firm grasp on the concept of a sampling distribution. Imagine you have a large population, let's say the heights of all adults in a country. It's impractical, if not impossible, to measure the height of every single person. Instead, we take a sample – a smaller, manageable subset of the population.
Now, imagine taking numerous samples (say, 1000 samples) of the same size from this population. For each sample, we calculate a statistic, such as the mean height. The distribution of these sample means (or any other statistic) is called the sampling distribution. This distribution provides valuable information about the population parameter we're interested in.
The Mean of the Sampling Distribution (Expected Value)
The mean of the sampling distribution, often denoted as μ<sub>x̄</sub> (mu sub x-bar), represents the average of all possible sample means. A remarkable property of the sampling distribution of the mean is that it's unbiased. This means the mean of the sampling distribution is equal to the population mean (μ). In simpler terms:
μ<sub>x̄</sub> = μ
This implies that if you were to take countless samples and average their means, you'd get a value very close to the true population mean. This fact is fundamental to inferential statistics, allowing us to estimate the population mean based on the sample mean.
Calculating the Mean of the Sampling Distribution
Calculating the true mean of the sampling distribution requires knowing the population mean, which is often unknown in real-world scenarios. However, the equation μ<sub>x̄</sub> = μ establishes the theoretical foundation for estimating the population mean using sample data. This is why the sample mean (x̄) serves as an unbiased estimator of the population mean (μ).
The Standard Deviation of the Sampling Distribution (Standard Error)
The standard deviation of the sampling distribution, known as the standard error, represents the variability or spread of the sample means. It indicates how much the sample means tend to deviate from the population mean. A smaller standard error suggests that the sample means are clustered tightly around the population mean, signifying a more precise estimation. A larger standard error implies greater variability among sample means, indicating less precision. The standard error is denoted by σ<sub>x̄</sub> (sigma sub x-bar) or SE.
Calculating the Standard Error
The standard error is calculated using the following formula:
σ<sub>x̄</sub> = σ / √n
Where:
- σ is the population standard deviation.
- n is the sample size.
This formula reveals a crucial relationship:
- Larger sample size (n) leads to a smaller standard error. This is because larger samples provide more accurate estimates of the population mean.
- Smaller population standard deviation (σ) leads to a smaller standard error. This indicates less variability within the population itself.
Note: In many cases, the population standard deviation (σ) is unknown. In such situations, we use the sample standard deviation (s) as an estimate. The formula then becomes:
s<sub>x̄</sub> = s / √n
This is an approximation of the standard error and is often used in practice.
The Central Limit Theorem
The Central Limit Theorem (CLT) is a cornerstone of inferential statistics. It states that the sampling distribution of the mean approaches a normal distribution as the sample size increases, regardless of the shape of the population distribution. This holds true as long as the sample size is sufficiently large (generally considered to be n ≥ 30). This is incredibly powerful because it allows us to use the properties of the normal distribution to make inferences about the population mean, even if we don't know the population distribution.
Using a Sampling Distribution Calculator
Calculating the mean and standard deviation of a sampling distribution manually can be tedious, especially with large datasets or complex scenarios. This is where a sampling distribution calculator comes in handy. These calculators automate the process, allowing you to input your sample data or parameters and instantly obtain the mean and standard error. They often include additional features, such as calculating confidence intervals, which are crucial for hypothesis testing.
Features of a Typical Sampling Distribution Calculator
A typical calculator would include:
- Input fields for sample data: You can either manually input your data points or upload a data file.
- Options for specifying population parameters: If you know the population mean and standard deviation, you can input these values directly.
- Calculation of the sample mean (x̄): This is a fundamental statistic.
- Calculation of the sample standard deviation (s): Used to estimate the population standard deviation when unknown.
- Calculation of the standard error (σ<sub>x̄</sub> or s<sub>x̄</sub>): The key output of the calculator.
- Visualization of the sampling distribution: Some calculators provide a graphical representation of the sampling distribution, giving a visual understanding of the spread and shape.
- Confidence interval calculation: Based on the standard error and the desired confidence level, the calculator computes the confidence interval for the population mean.
Applications of Sampling Distribution Calculations
The concepts of the mean and standard deviation of a sampling distribution are applied extensively across various fields:
- Quality Control: In manufacturing, sampling distributions help assess the quality of products by estimating the mean and variability of a specific characteristic (e.g., weight, length).
- Opinion Polls: Understanding sampling distributions is crucial for accurately estimating public opinion based on a sample survey. The standard error helps gauge the margin of error in the poll results.
- Medical Research: In clinical trials, sampling distributions are essential for analyzing treatment effectiveness by comparing the means and variability of outcomes between different treatment groups.
- Finance: Financial analysts use sampling distributions to estimate the average return and risk (standard deviation) of investments based on historical data.
- Environmental Science: Researchers use sampling distributions to analyze environmental data, such as pollution levels, estimating average levels and variability across different locations.
Understanding the Limitations
While powerful, it’s crucial to understand the limitations of using sampling distributions and calculators:
- Sample Bias: The accuracy of the results heavily relies on the representativeness of the sample. A biased sample can lead to inaccurate estimates of the population parameters.
- Assumption of Random Sampling: The theoretical framework of sampling distributions assumes random sampling. Non-random sampling techniques can distort the results.
- Large Sample Size Assumption (CLT): The Central Limit Theorem's applicability depends on a sufficiently large sample size. For small sample sizes, the sampling distribution may not be normal, potentially affecting the accuracy of the calculations.
- Estimation Errors: When the population standard deviation is unknown and the sample standard deviation is used as an estimate, there's inherent estimation error in the calculated standard error.
Conclusion
The mean and standard deviation of a sampling distribution are fundamental concepts in statistics with wide-ranging applications. Understanding these concepts allows us to make inferences about a population based on sample data. While manual calculations can be cumbersome, using a sampling distribution calculator streamlines the process, enabling quicker and more efficient analysis. However, always remember the importance of ensuring a representative sample and acknowledging the limitations of the approach for accurate and reliable results. By carefully considering these factors and utilizing the tools available, you can effectively leverage sampling distributions to gain valuable insights from your data.
Latest Posts
Latest Posts
-
2 Cups Dry Macaroni Equals How Much Cooked
Apr 05, 2025
-
How Much Is 15 Ml In Tablespoons
Apr 05, 2025
-
How Many Miles Is 8200 Steps
Apr 05, 2025
Related Post
Thank you for visiting our website which covers about Mean And Standard Deviation Of Sampling Distribution Calculator . We hope the information provided has been useful to you. Feel free to contact us if you have any questions or need further assistance. See you next time and don't miss to bookmark.