Mean Of The Distribution Of Sample Means Calculator
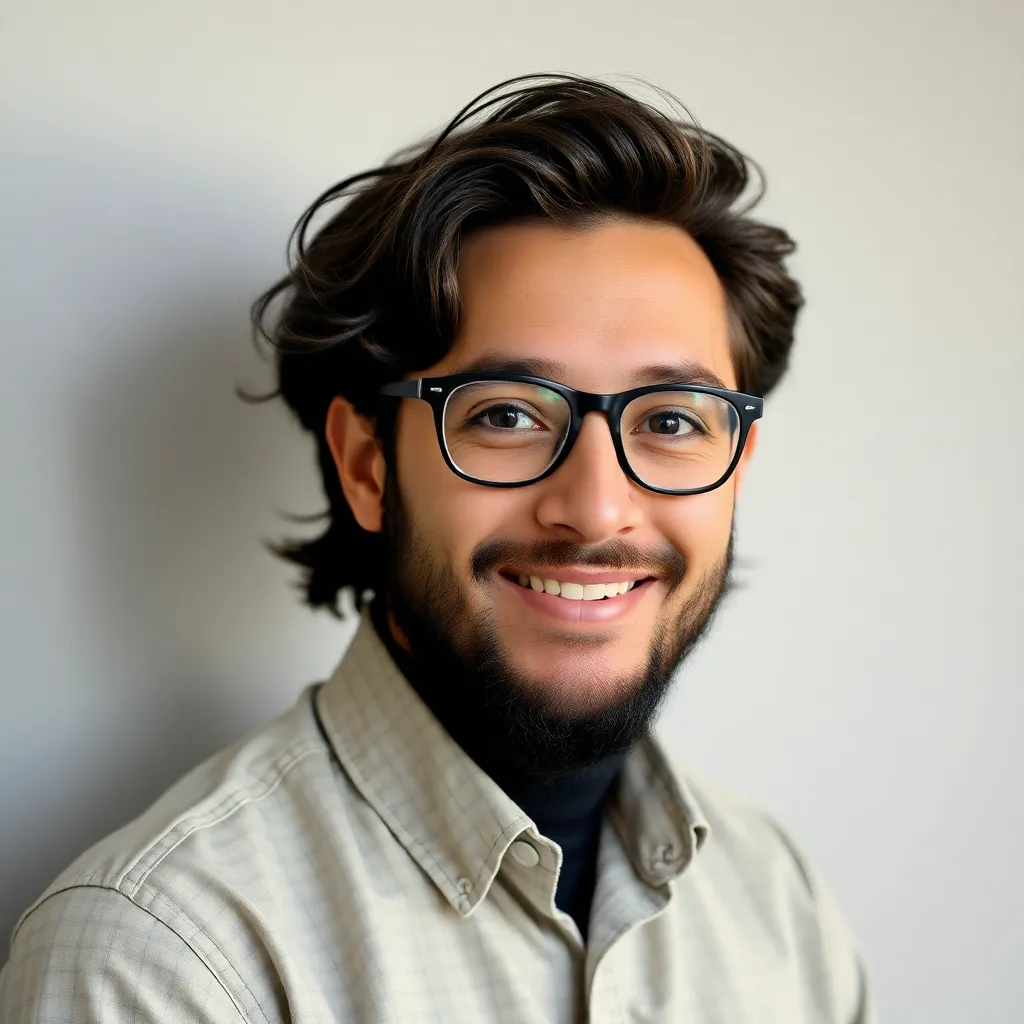
Treneri
Apr 05, 2025 · 6 min read

Table of Contents
Mean of the Distribution of Sample Means Calculator: A Comprehensive Guide
Understanding the distribution of sample means is crucial in statistics, forming the bedrock of inferential statistics and hypothesis testing. This article provides a comprehensive guide to the mean of the distribution of sample means, often denoted as μ<sub>x̄</sub> (mu sub x-bar), explaining its calculation, significance, and practical applications. We'll also explore how to use a hypothetical "mean of the distribution of sample means calculator" (although such a dedicated calculator might not exist in a single, readily available tool; the principles will apply to standard statistical software and online resources). Finally, we'll touch upon the relationship between the sample mean, population mean, and the Central Limit Theorem.
What is the Distribution of Sample Means?
Before diving into the mean of the distribution, let's understand the concept of the distribution of sample means itself. Imagine you're drawing repeated random samples of a fixed size (n) from a population. For each sample, you calculate the mean (x̄). If you plotted all these sample means, you'd create a distribution – the distribution of sample means. This distribution is not the same as the original population distribution; it has its own characteristics, including its own mean and standard deviation.
The Mean of the Distribution of Sample Means (μ<sub>x̄</sub>)
The mean of the distribution of sample means (μ<sub>x̄</sub>) represents the average of all possible sample means. Importantly, this mean is equal to the population mean (μ). This is a fundamental result in statistics.
μ<sub>x̄</sub> = μ
This equality holds true regardless of the sample size (n), provided that the samples are randomly selected. This property significantly simplifies statistical inference because it allows us to estimate the population mean based on the sample mean.
Significance of μ<sub>x̄</sub>
The equality of μ<sub>x̄</sub> and μ has profound implications:
-
Unbiased Estimator: The sample mean (x̄) is an unbiased estimator of the population mean (μ). This means that, on average, the sample mean will accurately reflect the population mean. There's no systematic overestimation or underestimation.
-
Foundation of Inference: This equality forms the basis for many statistical inference techniques. We use the sample mean to make inferences about the population mean, testing hypotheses, constructing confidence intervals, and drawing conclusions about the population based on sample data.
-
Central Limit Theorem Connection: The Central Limit Theorem (CLT) builds upon this concept. The CLT states that the distribution of sample means will approximate a normal distribution, regardless of the shape of the original population distribution, as the sample size (n) increases. This is true even if the population itself is not normally distributed.
Calculating μ<sub>x̄</sub>: A Hypothetical Calculator Approach
While a dedicated "mean of the distribution of sample means calculator" may not exist as a single tool, the calculation is straightforward. Since μ<sub>x̄</sub> = μ, you would need to know the population mean (μ). Let's outline a hypothetical calculator workflow:
1. Input: The hypothetical calculator would require you to input the population mean (μ).
2. Calculation: Internally, the calculator would simply return the input value (μ) as the mean of the distribution of sample means (μ<sub>x̄</sub>). There's no further calculation needed.
3. Output: The calculator would display the population mean (μ) as the output, labeled as the "Mean of the Distribution of Sample Means."
Practical Example (using real-world statistical software): In practice, you wouldn't use a hypothetical calculator. You'd use statistical software like R, Python (with libraries like NumPy and SciPy), SPSS, or Excel. If you have your data, these tools can calculate the sample mean (x̄), which is your best estimate of μ<sub>x̄</sub> and therefore of the population mean μ.
Standard Error of the Mean
While the mean of the distribution of sample means is equal to the population mean, the spread of the distribution is crucial. This spread is measured by the standard error of the mean (SEM), often denoted as σ<sub>x̄</sub> (sigma sub x-bar).
σ<sub>x̄</sub> = σ / √n
where:
- σ is the population standard deviation
- n is the sample size
The standard error of the mean represents the standard deviation of the distribution of sample means. Notice that as the sample size (n) increases, the standard error decreases. This implies that larger samples lead to a more precise estimate of the population mean.
Central Limit Theorem and its Implications
The Central Limit Theorem (CLT) is essential when dealing with the distribution of sample means. The CLT states that, for sufficiently large sample sizes (generally n ≥ 30), the distribution of sample means will be approximately normally distributed, regardless of the shape of the original population distribution. This is a powerful result because it allows us to use the normal distribution to make inferences about the population mean, even if the population distribution is non-normal.
This is where our hypothetical "calculator" would be particularly useful if it included CLT considerations. For example, it could display not only μ<sub>x̄</sub> but also the standard error and provide approximations based on the CLT.
Applications of μ<sub>x̄</sub> and the Distribution of Sample Means
The concept of the mean of the distribution of sample means and the distribution of sample means is fundamental across a vast range of statistical applications:
-
Hypothesis Testing: We use the distribution of sample means to conduct hypothesis tests about population means. For example, we might test whether a new drug significantly lowers blood pressure compared to a placebo, or whether a new teaching method improves student test scores.
-
Confidence Intervals: We construct confidence intervals to estimate the range of values within which the population mean is likely to fall, based on the sample mean and the standard error. These intervals quantify the uncertainty associated with our estimate.
-
Sample Size Determination: Understanding the distribution of sample means helps determine the appropriate sample size needed for a study to achieve a desired level of precision.
-
Quality Control: In manufacturing and other industries, the distribution of sample means is used to monitor product quality and ensure that processes are operating within acceptable limits.
-
Polling and Surveys: In political polling and market research surveys, the distribution of sample means is essential for understanding the margin of error and the confidence level associated with estimates of population proportions or averages.
Beyond the Mean: Other Properties of the Distribution of Sample Means
While we've focused heavily on μ<sub>x̄</sub>, it's important to remember that the distribution of sample means has other key characteristics:
- Shape: As per the Central Limit Theorem, the distribution's shape approaches normality as sample size (n) increases.
- Spread (Standard Error): The standard error of the mean (SEM) quantifies the spread. A smaller SEM indicates greater precision in estimating the population mean.
- Skewness and Kurtosis: These measures describe the asymmetry and peakedness of the distribution, respectively. While the CLT ensures approximate normality for large n, smaller samples might retain some skewness or kurtosis from the original population.
Conclusion
The mean of the distribution of sample means (μ<sub>x̄</sub>), which equals the population mean (μ), is a cornerstone of statistical inference. Understanding this concept and the associated distribution of sample means, along with the implications of the Central Limit Theorem, is crucial for anyone working with statistical data. While a dedicated "mean of the distribution of sample means calculator" might not be readily available as a standalone tool, standard statistical software readily calculates sample means and provides tools for inferential statistics, allowing us to easily derive estimations and confidence intervals related to the population mean. The principles outlined here equip you to confidently navigate these essential statistical concepts and effectively utilize them in data analysis.
Latest Posts
Latest Posts
-
How Many Metres Is 12 Foot
Apr 06, 2025
-
Which Line Segment Is Drawn In The Figure
Apr 06, 2025
-
16 Is What Percent Of 8
Apr 06, 2025
-
If You Born In 1982 How Old Are You
Apr 06, 2025
-
How To Measure The Pitch Of A Thread
Apr 06, 2025
Related Post
Thank you for visiting our website which covers about Mean Of The Distribution Of Sample Means Calculator . We hope the information provided has been useful to you. Feel free to contact us if you have any questions or need further assistance. See you next time and don't miss to bookmark.