Reject Or Fail To Reject The Null Hypothesis Calculator
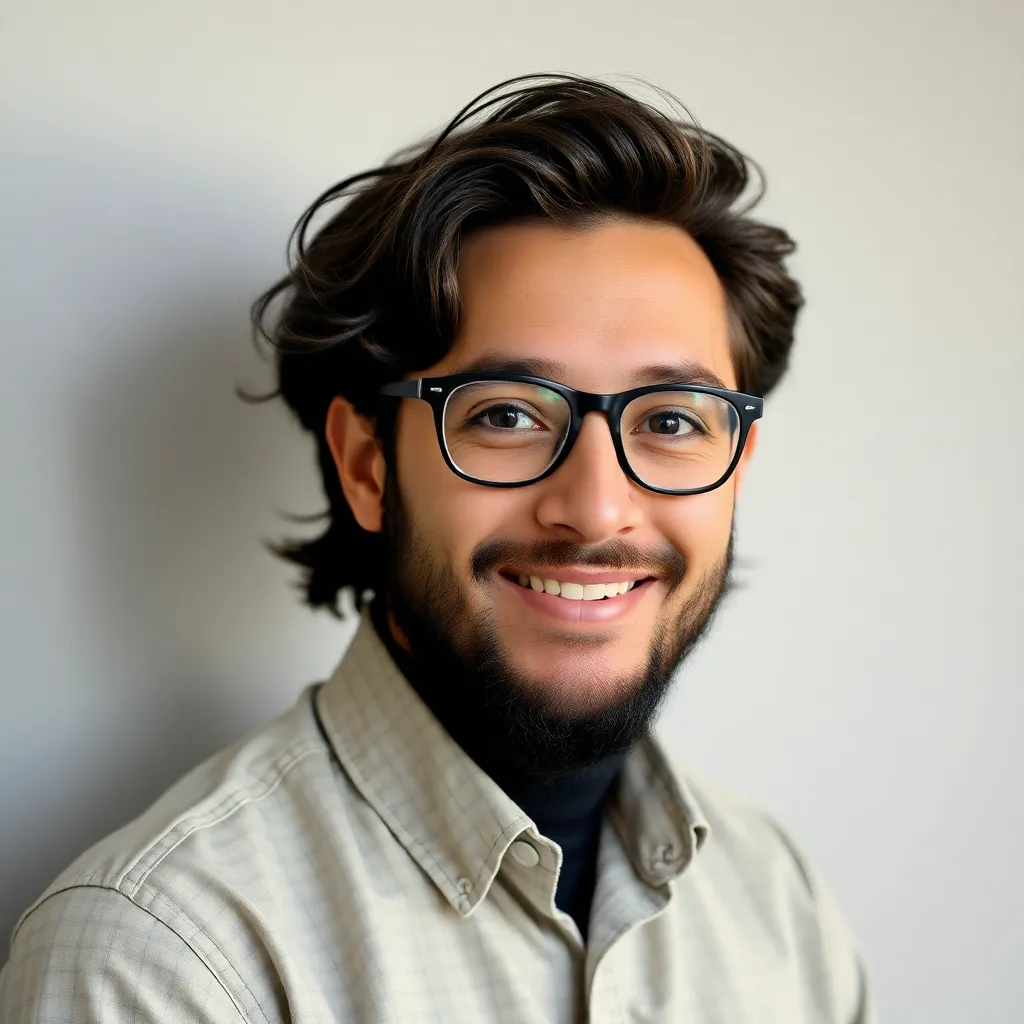
Treneri
Apr 22, 2025 · 6 min read

Table of Contents
Reject or Fail to Reject the Null Hypothesis Calculator: A Comprehensive Guide
Understanding statistical hypothesis testing is crucial for researchers across various fields. A core component of this process is deciding whether to reject or fail to reject the null hypothesis. While the concept is straightforward in theory, the practical application can be complex. This article will delve into the intricacies of hypothesis testing, explaining the role of p-values, significance levels, and the use of calculators (though we won't link to specific ones) to determine the ultimate conclusion. We'll explore different statistical tests and provide a clear, step-by-step guide to interpreting your results.
Understanding Hypothesis Testing: The Foundation
Hypothesis testing is a formal procedure used to determine whether there is enough evidence to support a claim about a population. It involves formulating a null hypothesis (H₀), which represents the status quo or no effect, and an alternative hypothesis (H₁ or Hₐ), which represents the claim being investigated. The goal is to assess the plausibility of the null hypothesis given the observed data.
Key Terminology:
- Null Hypothesis (H₀): A statement of no effect or no difference. For example, "There is no difference in average height between men and women."
- Alternative Hypothesis (H₁ or Hₐ): A statement that contradicts the null hypothesis. It could be one-tailed (e.g., "Men are taller than women") or two-tailed (e.g., "There is a difference in average height between men and women").
- Significance Level (α): The probability of rejecting the null hypothesis when it is actually true (Type I error). Commonly set at 0.05 (5%), meaning there's a 5% chance of incorrectly rejecting the null hypothesis.
- P-value: The probability of obtaining results as extreme as, or more extreme than, the observed results, assuming the null hypothesis is true. A smaller p-value suggests stronger evidence against the null hypothesis.
- Test Statistic: A numerical value calculated from the sample data that is used to assess the evidence against the null hypothesis.
- Critical Value: The value that separates the rejection region from the non-rejection region. If the test statistic falls within the rejection region, the null hypothesis is rejected.
- Degrees of Freedom (df): A parameter that reflects the number of independent pieces of information available to estimate a population parameter. This is particularly relevant for tests involving samples from populations with unknown variances.
The Role of a "Reject or Fail to Reject the Null Hypothesis Calculator"
While there isn't a single calculator specifically named "Reject or Fail to Reject the Null Hypothesis Calculator," numerous statistical software packages and online tools perform the calculations involved in hypothesis testing. These tools automate the process of calculating test statistics and p-values, making the decision-making process more efficient. The crucial understanding remains interpreting the output of these calculators, which ultimately determines whether to reject or fail to reject the null hypothesis.
These calculators typically require the following inputs:
- Type of Test: (e.g., t-test, z-test, chi-square test, ANOVA) The appropriate test depends on the type of data and the research question.
- Sample Data: This includes the sample means, standard deviations, sample sizes, and any other relevant statistics.
- Significance Level (α): Usually set at 0.05 but can be adjusted depending on the context.
- One-tailed or Two-tailed Test: This depends on the alternative hypothesis.
The calculator then outputs the test statistic, p-value, and potentially critical values. The decision to reject or fail to reject H₀ is made based on comparing the p-value to the significance level.
Decision Making: P-value vs. Significance Level
The core of the decision-making process lies in comparing the p-value to the pre-determined significance level (α).
- If p-value ≤ α: Reject the null hypothesis. There is sufficient evidence to support the alternative hypothesis. The results are statistically significant.
- If p-value > α: Fail to reject the null hypothesis. There is not enough evidence to reject the null hypothesis. The results are not statistically significant. This does not mean the null hypothesis is true; it simply means there is insufficient evidence to reject it.
It's crucial to remember that failing to reject the null hypothesis is not the same as accepting the null hypothesis. It simply means the data does not provide enough evidence to refute it.
Common Statistical Tests and Their Applications
Various statistical tests are used in hypothesis testing, each tailored to specific data types and research questions:
1. Z-test: Used to test hypotheses about population means when the population standard deviation is known or the sample size is large (n ≥ 30).
2. T-test: Used to test hypotheses about population means when the population standard deviation is unknown. There are different versions:
* **One-sample t-test:** Compares the mean of a single sample to a known population mean.
* **Independent samples t-test:** Compares the means of two independent groups.
* **Paired samples t-test:** Compares the means of two related groups (e.g., before and after measurements on the same individuals).
3. Chi-square test: Used to analyze categorical data and test for associations between categorical variables. There are different types:
* **Chi-square goodness-of-fit test:** Tests whether the observed frequencies of a categorical variable differ significantly from expected frequencies.
* **Chi-square test of independence:** Tests whether two categorical variables are independent.
4. ANOVA (Analysis of Variance): Used to compare the means of three or more groups.
Interpreting Results and Avoiding Common Pitfalls
Interpreting the results of a hypothesis test requires careful consideration of several factors:
-
Context: The statistical significance (p-value) should always be interpreted within the context of the research question and the practical implications of the findings. A statistically significant result might not be practically significant.
-
Sample Size: Larger sample sizes tend to produce more precise estimates and increase the power of the test, making it more likely to detect a true effect.
-
Effect Size: Statistical significance doesn't necessarily imply a large effect size. The effect size quantifies the magnitude of the difference between groups or the strength of the association between variables.
-
Type I and Type II Errors: It's important to understand the possibility of making errors in hypothesis testing:
- Type I error (false positive): Rejecting the null hypothesis when it is actually true. The significance level (α) controls the probability of making a Type I error.
- Type II error (false negative): Failing to reject the null hypothesis when it is actually false. The power of the test (1 - β) determines the probability of avoiding a Type II error.
Beyond the Calculator: The Importance of Statistical Understanding
While calculators expedite the calculations in hypothesis testing, it's crucial to understand the underlying statistical principles. Blindly using a calculator without grasping the concepts can lead to misinterpretations and incorrect conclusions. A thorough understanding of the chosen statistical test, its assumptions, and the limitations of the results is essential for drawing meaningful inferences from the data.
Remember to always critically assess the assumptions of the chosen test and ensure your data meets those assumptions before interpreting the results. Violating test assumptions can invalidate the results and lead to misleading conclusions.
Conclusion
The ability to reject or fail to reject the null hypothesis is a fundamental skill in statistical analysis. While "reject or fail to reject the null hypothesis calculator" tools simplify the numerical computations, a strong grasp of the underlying principles and the careful interpretation of the results are paramount. Understanding the different types of hypothesis tests, their assumptions, and the potential for errors is vital for conducting meaningful research and drawing accurate conclusions. Remember that statistical significance should always be considered in conjunction with practical significance and contextual understanding.
Latest Posts
Latest Posts
-
What Is 75 Off Of 30
Apr 22, 2025
-
How Time And A Half Is Calculated
Apr 22, 2025
-
How To Calculate Heat Gained By Water
Apr 22, 2025
-
How Many Centiliters Are There In A Liter
Apr 22, 2025
-
How Old Are You On Other Planets
Apr 22, 2025
Related Post
Thank you for visiting our website which covers about Reject Or Fail To Reject The Null Hypothesis Calculator . We hope the information provided has been useful to you. Feel free to contact us if you have any questions or need further assistance. See you next time and don't miss to bookmark.