Standard Error Of The Estimate Calculator
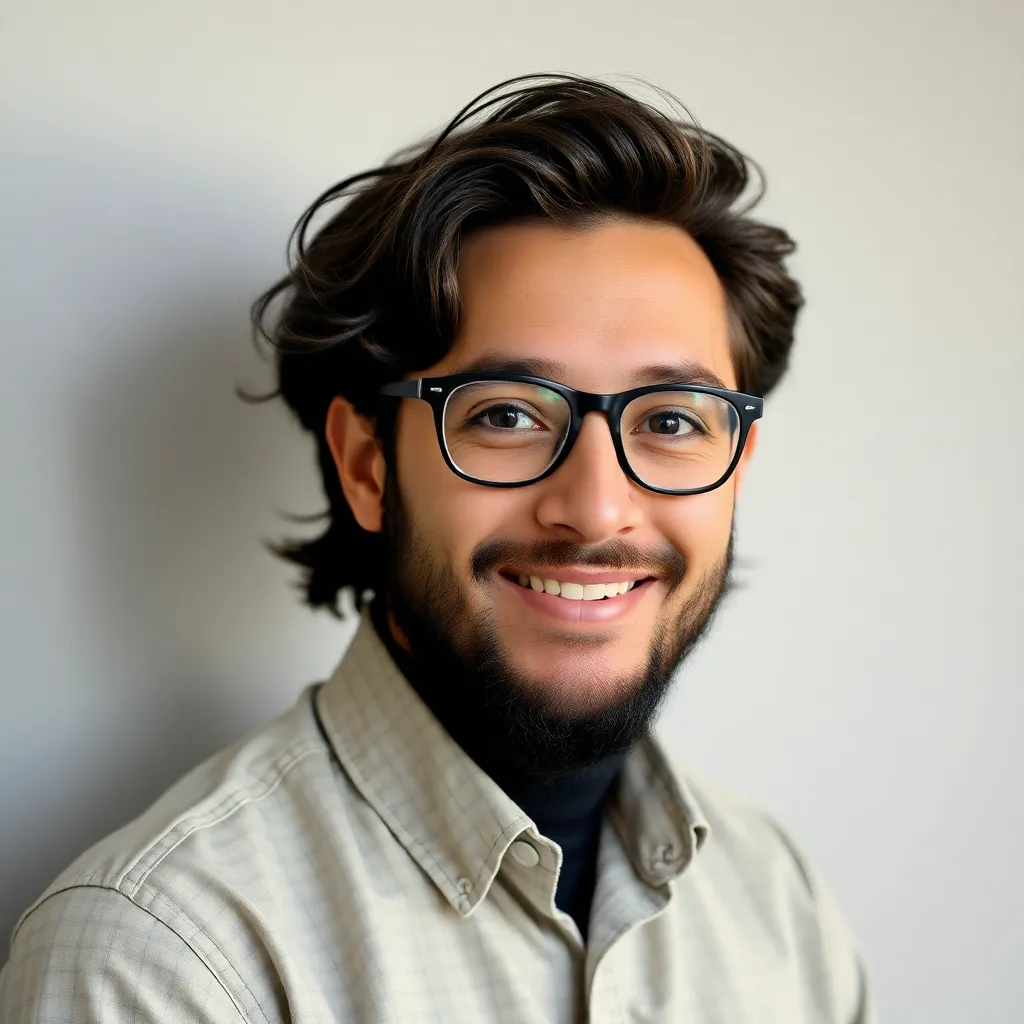
Treneri
Apr 17, 2025 · 6 min read

Table of Contents
Understanding and Utilizing a Standard Error of the Estimate Calculator
The standard error of the estimate (SEE), also known as the standard error of regression, is a crucial statistical measure that quantifies the accuracy of predictions made by a regression model. It essentially tells us how much the observed values deviate from the values predicted by the model. A lower SEE indicates a better-fitting model, implying that the model's predictions are more reliable and closer to the actual data. This article will delve into the intricacies of SEE, explain its calculation, explore its importance, and guide you through the effective use of a standard error of the estimate calculator.
What is the Standard Error of the Estimate?
In simpler terms, the SEE represents the average distance between the actual data points and the regression line. Imagine plotting your data points on a graph and fitting a regression line through them. The SEE measures the typical vertical distance between each data point and the line. A smaller SEE suggests that the data points cluster closely around the regression line, indicating a strong relationship between the variables and a highly accurate model. Conversely, a larger SEE signifies a greater scatter of data points around the line, suggesting a weaker relationship and less accurate predictions.
SEE vs. Standard Deviation: Key Differences
While both SEE and standard deviation measure dispersion, they are distinct concepts. Standard deviation measures the dispersion of a single variable around its mean, whereas SEE measures the dispersion of the residuals (the differences between observed and predicted values) around the regression line. The SEE specifically reflects the accuracy of the regression model in predicting the dependent variable.
Calculating the Standard Error of the Estimate
Calculating the SEE manually can be tedious, especially with larger datasets. Fortunately, statistical software and online calculators simplify this process. The formula for calculating SEE is:
SEE = √[Σ(yᵢ - ŷᵢ)² / (n - k)]
Where:
- yᵢ represents the actual value of the dependent variable for the i-th observation.
- ŷᵢ represents the predicted value of the dependent variable for the i-th observation based on the regression model.
- n represents the total number of observations.
- k represents the number of independent variables in the model (plus 1 for the intercept).
This formula involves several steps:
-
Calculate the predicted values (ŷᵢ): This requires fitting a regression model to your data, obtaining the regression equation, and using it to predict the dependent variable for each observation.
-
Calculate the residuals (yᵢ - ŷᵢ): Subtract each predicted value from its corresponding actual value.
-
Square the residuals: Square each residual to eliminate negative values and emphasize larger deviations.
-
Sum the squared residuals: Add up all the squared residuals.
-
Divide by (n - k): Divide the sum of squared residuals by the degrees of freedom (n - k), which accounts for the number of parameters estimated in the model.
-
Take the square root: Finally, take the square root of the result to obtain the SEE.
The Importance of the Standard Error of the Estimate
The SEE plays a vital role in various statistical analyses and applications:
-
Model Evaluation: The SEE is a key metric for assessing the goodness of fit of a regression model. A smaller SEE indicates a better fit, suggesting that the model accurately predicts the dependent variable.
-
Prediction Intervals: The SEE is used to construct prediction intervals around predicted values. These intervals provide a range within which the actual value is likely to fall, with a specified level of confidence. A smaller SEE leads to narrower prediction intervals, indicating more precise predictions.
-
Hypothesis Testing: The SEE is also involved in hypothesis testing related to regression models. It contributes to calculating test statistics used to determine the significance of the regression coefficients.
-
Comparison of Models: When comparing multiple regression models, the SEE can be used to choose the model that provides the most accurate predictions. The model with the smallest SEE is generally preferred.
-
Understanding Model Limitations: A large SEE indicates that the model may not accurately capture the underlying relationship between the variables. This might suggest the need to include additional variables, transform variables, or use a different type of model.
Utilizing a Standard Error of the Estimate Calculator
Standard error of the estimate calculators are readily available online. These calculators simplify the process by automating the calculations involved in determining the SEE. They typically require you to input your data (actual and predicted values) and then automatically compute the SEE. This eliminates the need for manual calculations, saving time and reducing the risk of errors.
Steps to Use an Online SEE Calculator:
-
Gather your data: Collect your actual values (yᵢ) and predicted values (ŷᵢ) from your regression analysis. Ensure your data is organized correctly, typically in paired sets of actual and predicted values.
-
Find a reliable calculator: Search online for "standard error of the estimate calculator." Several free calculators are available. Choose one that appears reputable and user-friendly. Look for calculators that clearly display the formula used and allow for various data input methods.
-
Input your data: Enter your actual and predicted values into the calculator. Most calculators allow data input through various methods, such as manual entry, uploading a CSV file, or pasting data from a spreadsheet. Double-check your entries to avoid errors.
-
Calculate the SEE: Click the "Calculate" button or equivalent to initiate the calculation. The calculator will compute the SEE and display the result.
-
Interpret the result: Analyze the SEE value. A lower SEE indicates a better-fitting model with more accurate predictions. Compare this value to the standard deviation of your dependent variable for context.
Interpreting the SEE Value
The SEE value itself doesn't have a direct interpretation in terms of units. However, it's crucial to consider it in relation to the scale of your dependent variable. A small SEE compared to the range of your dependent variable suggests a strong fit, while a large SEE relative to the range implies a weaker fit. It's also helpful to compare the SEE across different models to determine which model provides the best fit for your data.
Advanced Considerations and Applications
The SEE is a fundamental concept, but its application extends beyond simple linear regression. Its principles are applicable in various contexts, including:
-
Multiple Linear Regression: SEE is equally important in evaluating the accuracy of predictions made by multiple linear regression models, which include multiple independent variables.
-
Non-linear Regression: Although the calculation might differ slightly, the underlying principle of measuring prediction error remains the same in non-linear regression models.
-
Time Series Analysis: SEE can be used to evaluate the accuracy of forecasting models in time series analysis.
-
Model Selection: When comparing different models, the SEE can help you select the one that provides the best balance between model complexity and predictive accuracy. A more complex model might not always lead to a lower SEE, highlighting the importance of considering the trade-off between model complexity and predictive accuracy.
Conclusion: Mastering the Standard Error of the Estimate
The standard error of the estimate is a powerful tool for evaluating the accuracy and reliability of regression models. Understanding its calculation and interpretation is essential for anyone working with regression analysis. While manual calculation can be complex, utilizing a standard error of the estimate calculator streamlines the process, enabling researchers and analysts to focus on the interpretation and application of this vital statistical measure. By mastering the use of the SEE, you can improve your ability to build robust and accurate predictive models and draw meaningful conclusions from your data. Remember to always consider the context of your data and the limitations of your model when interpreting the SEE. This will lead to more reliable and informative analyses.
Latest Posts
Latest Posts
-
How Many Milligrams Is 500 Mcg
Apr 19, 2025
-
How Many Weeks Are In 19 Days
Apr 19, 2025
-
How Many Hours Is 1000 Seconds
Apr 19, 2025
-
90 Days From March 5 2024
Apr 19, 2025
-
How Many Cubic In A Gallon
Apr 19, 2025
Related Post
Thank you for visiting our website which covers about Standard Error Of The Estimate Calculator . We hope the information provided has been useful to you. Feel free to contact us if you have any questions or need further assistance. See you next time and don't miss to bookmark.