The Standard Deviation Of The Distribution Of Sample Means Is
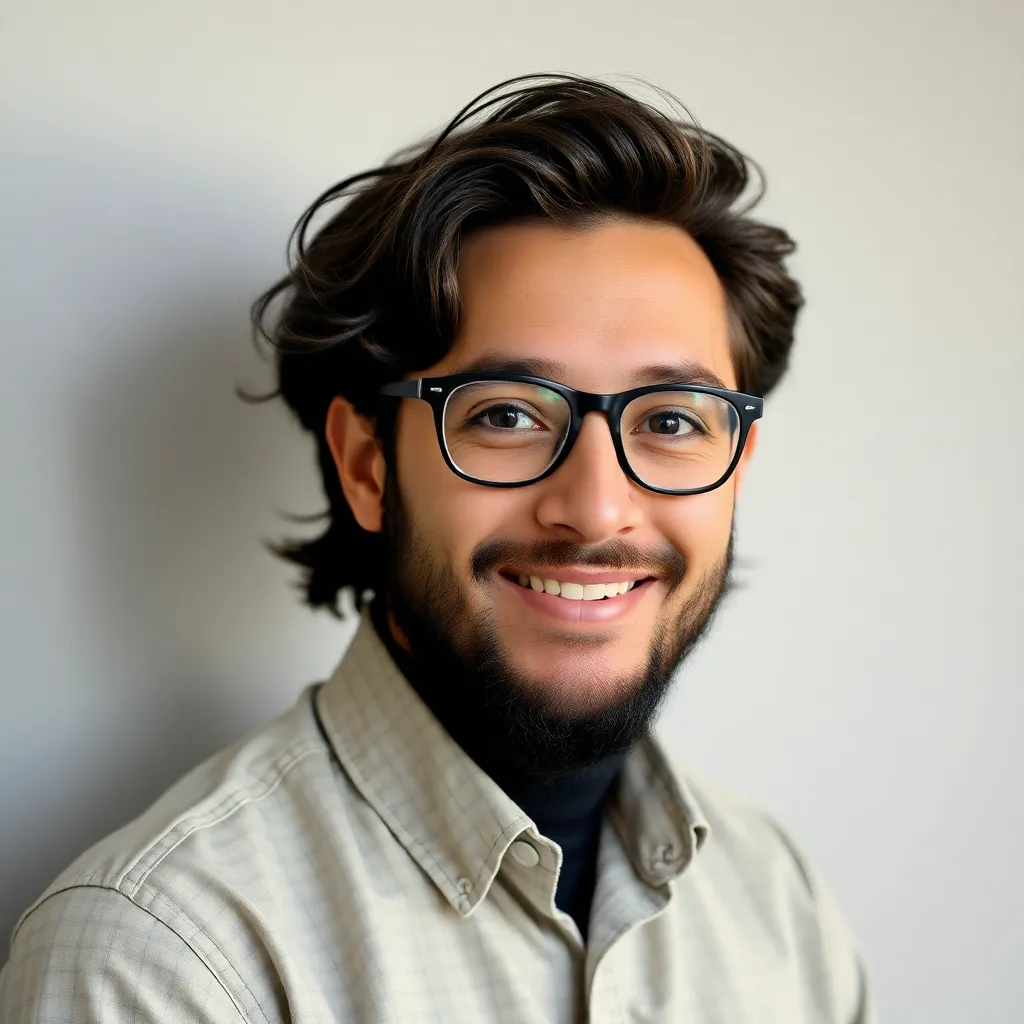
Treneri
Apr 17, 2025 · 6 min read

Table of Contents
The Standard Deviation of the Distribution of Sample Means: Understanding Standard Error
The standard deviation of the distribution of sample means, also known as the standard error of the mean (SEM), is a crucial concept in statistics. It quantifies the variability you'd expect to see in the average of multiple samples drawn from the same population. Understanding the SEM is essential for interpreting statistical results, constructing confidence intervals, and performing hypothesis testing. This article will delve deep into the SEM, explaining its calculation, its relationship to the population standard deviation, and its applications.
What is the Standard Error of the Mean (SEM)?
Imagine you're measuring the height of adult women. You could measure the height of every single woman in the world (the population), but this is practically impossible. Instead, you take a sample – say, 100 women – and calculate their average height. Now, if you repeated this process many times, drawing different samples of 100 women each, you'd get a slightly different average height each time. These sample means themselves form a distribution, and this distribution has its own standard deviation – that's the SEM.
In simpler terms: The SEM tells us how much the sample means are likely to vary from the true population mean. A smaller SEM indicates that the sample means are clustered tightly around the population mean, suggesting that our sample mean is a more reliable estimate. A larger SEM implies greater variability among sample means, indicating less precision in our estimate.
Calculating the Standard Error of the Mean
The SEM is calculated using the following formula:
SEM = σ / √n
Where:
- σ (sigma): Represents the population standard deviation. This is the standard deviation of the individual data points in the entire population.
- n: Represents the sample size. This is the number of data points in each sample.
Important Note: In most real-world scenarios, the true population standard deviation (σ) is unknown. Therefore, we usually estimate the SEM using the sample standard deviation (s) instead:
SEM ≈ s / √n
Where:
- s: Represents the sample standard deviation, calculated from your sample data.
This estimated SEM is an approximation, but it becomes increasingly accurate as the sample size (n) increases. The larger your sample, the closer the sample standard deviation (s) will be to the population standard deviation (σ).
The Relationship Between SEM and Sample Size
The formula clearly shows an inverse relationship between the SEM and the sample size (n). As the sample size increases, the SEM decreases. This makes intuitive sense: the larger your sample, the more representative it is of the population, and the less your sample mean will vary from the true population mean. Therefore, a larger sample size leads to a more precise estimate of the population mean.
This relationship is crucial for experimental design. If you want to achieve a smaller SEM and thus a more precise estimate, you need to increase your sample size. However, increasing sample size also increases the cost and time required for data collection. Researchers often need to balance the desired precision with practical constraints.
Understanding the Central Limit Theorem
The concept of the SEM is deeply connected to the Central Limit Theorem (CLT). The CLT states that, regardless of the shape of the population distribution, the distribution of sample means will approximate a normal distribution as the sample size (n) increases. This is true even if the original population data isn't normally distributed.
The CLT is fundamental because it allows us to use the properties of the normal distribution to make inferences about the population mean, even when we don't know the population distribution. This is a powerful tool in statistical inference.
Applications of the Standard Error of the Mean
The SEM has numerous applications in various fields, including:
-
Confidence Intervals: The SEM is a key component in calculating confidence intervals for the population mean. A confidence interval provides a range of values within which the true population mean is likely to lie with a certain degree of confidence (e.g., a 95% confidence interval). The formula for a confidence interval is:
Confidence Interval = Sample Mean ± (Critical Value * SEM)
The critical value depends on the desired confidence level and the sample size.
-
Hypothesis Testing: The SEM is used in hypothesis testing to determine whether there is a statistically significant difference between two groups or between a sample mean and a hypothesized population mean. Many statistical tests, such as t-tests and z-tests, use the SEM to calculate the test statistic.
-
Meta-Analysis: In meta-analysis, researchers combine results from multiple studies to obtain a more precise estimate of an effect size. The SEM from each individual study is used to weight the results in the meta-analysis, giving more weight to studies with smaller SEMs (i.e., more precise estimates).
-
Process Control: In quality control, the SEM is used to monitor the stability of a process. By tracking the SEM of samples taken over time, one can detect shifts in the process mean that may indicate problems.
Interpreting the Standard Error of the Mean
A smaller SEM indicates a more precise estimate of the population mean. A larger SEM suggests more variability in the sample means and therefore a less precise estimate. However, the absolute value of the SEM doesn't tell the whole story; it needs to be considered in relation to the sample mean itself.
For example, an SEM of 0.5 might be considered small if the sample mean is 100, but it would be considered large if the sample mean is only 2. This is why it's often more useful to consider the SEM as a percentage of the sample mean (SEM/Mean * 100%) to get a better sense of the precision of the estimate.
Distinguishing SEM from Standard Deviation
It's crucial to distinguish between the SEM and the standard deviation. The standard deviation (σ or s) measures the variability of individual data points within a sample or population. The SEM, on the other hand, measures the variability of sample means across multiple samples. The SEM is always smaller than the standard deviation (unless the sample size is 1).
Factors Affecting the Standard Error of the Mean
Several factors can influence the SEM:
- Population Variability: A population with high variability (high σ) will have a larger SEM than a population with low variability.
- Sample Size: As already discussed, a larger sample size leads to a smaller SEM.
- Sampling Method: The method used to select the sample can affect the SEM. A biased sampling method can lead to an inaccurate estimate of the SEM.
Conclusion
The standard error of the mean is a fundamental concept in inferential statistics. It provides a measure of the uncertainty associated with estimating the population mean from a sample. Understanding the SEM is crucial for interpreting statistical results, constructing confidence intervals, conducting hypothesis tests, and making informed decisions based on sample data. By understanding its calculation, its relationship to sample size, and its various applications, you can significantly enhance your ability to analyze and interpret statistical information. Remember to always consider the SEM in conjunction with the sample mean and other relevant statistical measures for a complete and accurate interpretation of your data.
Latest Posts
Latest Posts
-
How Long For Soda To Get Cold In Fridge
Apr 19, 2025
-
18 Is What Percent Of 100
Apr 19, 2025
-
What Is 25 Knots In Mph
Apr 19, 2025
-
How Many Milligrams Is 500 Mcg
Apr 19, 2025
-
How Many Weeks Are In 19 Days
Apr 19, 2025
Related Post
Thank you for visiting our website which covers about The Standard Deviation Of The Distribution Of Sample Means Is . We hope the information provided has been useful to you. Feel free to contact us if you have any questions or need further assistance. See you next time and don't miss to bookmark.