What Percent Is 3 Standard Deviations
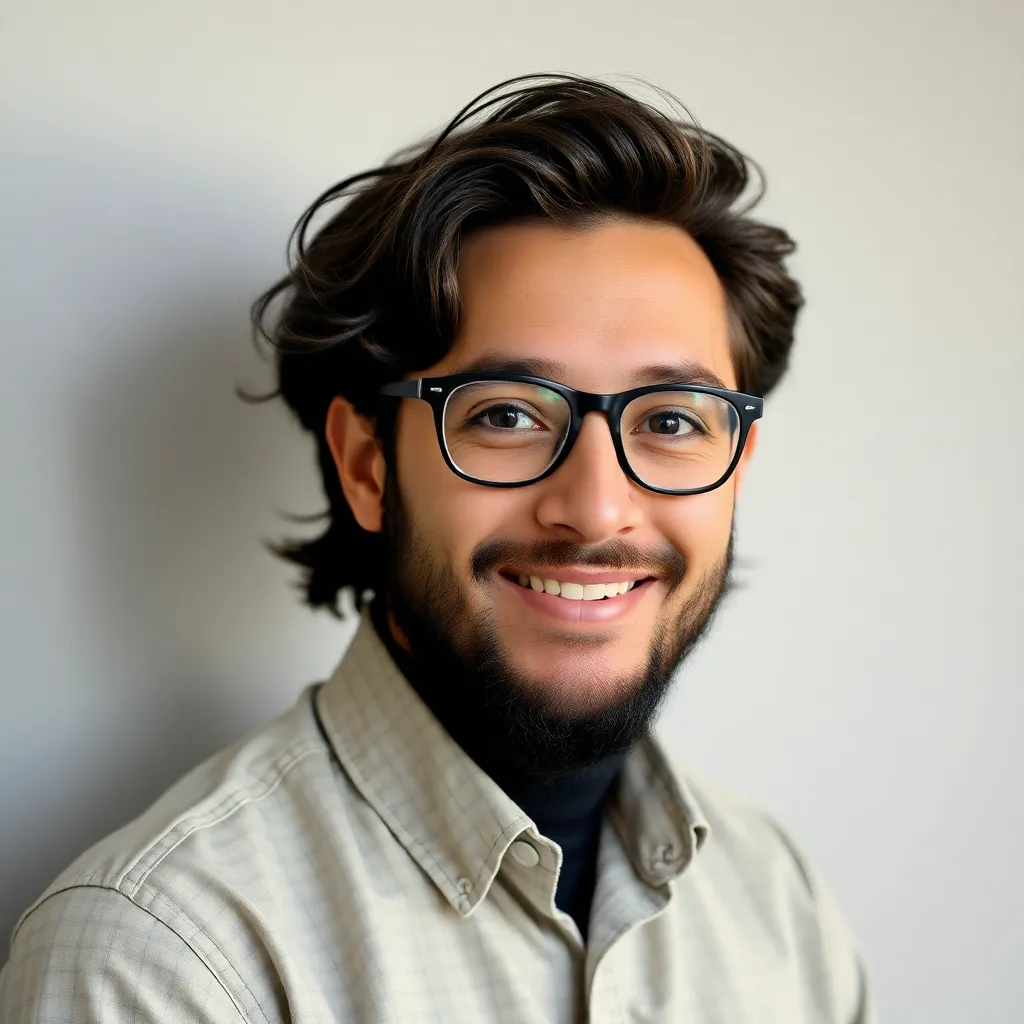
Treneri
May 15, 2025 · 5 min read
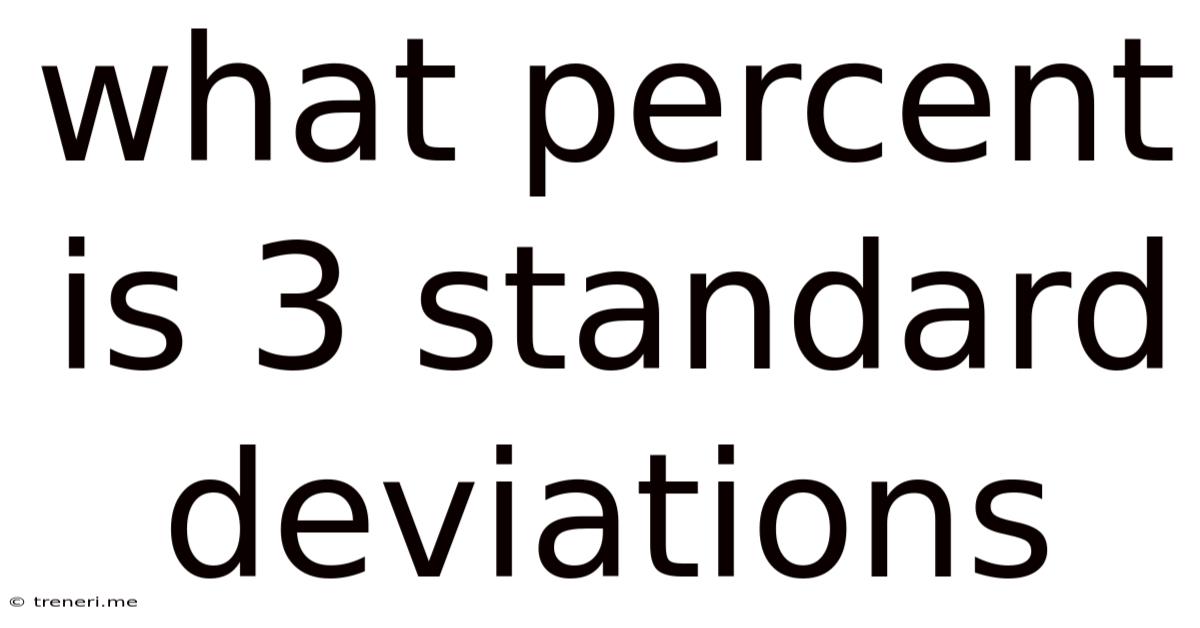
Table of Contents
What Percent is 3 Standard Deviations? A Comprehensive Guide
Understanding standard deviation and its relationship to probability is crucial in many fields, from statistics and finance to engineering and quality control. A common question that arises is: what percentage of data falls within three standard deviations of the mean? This article provides a detailed explanation, exploring the empirical rule, its limitations, and the implications for various applications.
The Empirical Rule (68-95-99.7 Rule)
The empirical rule, also known as the 68-95-99.7 rule, is a handy guideline for interpreting data distributed normally (or approximately normally). It states that for a normal distribution:
- Approximately 68% of data points fall within one standard deviation of the mean. This means the data lies between (mean - 1 standard deviation) and (mean + 1 standard deviation).
- Approximately 95% of data points fall within two standard deviations of the mean. This corresponds to the range (mean - 2 standard deviations) and (mean + 2 standard deviations).
- Approximately 99.7% of data points fall within three standard deviations of the mean. This is the range (mean - 3 standard deviations) and (mean + 3 standard deviations).
This rule provides a quick estimate of the probability of observing data within a certain distance from the mean. It's important to emphasize that these are approximate percentages. The true percentages depend on the precise shape of the normal distribution, although the deviations are generally small.
Visualizing the Empirical Rule
Imagine a bell curve, the classic representation of a normal distribution. The mean sits at the peak. One standard deviation to either side encompasses a significant portion of the data, two standard deviations even more, and three standard deviations virtually all of it. The percentages mentioned above represent the area under the curve within those respective ranges.
Beyond the Empirical Rule: Chebyshev's Inequality
While the empirical rule is useful for normally distributed data, it doesn't apply to all data sets. For data that isn't normally distributed, we can use Chebyshev's inequality. This theorem provides a lower bound for the proportion of data that falls within a specified number of standard deviations from the mean, regardless of the distribution's shape.
Chebyshev's inequality states that for any data set, the proportion of data within k standard deviations of the mean is at least 1 - 1/k², where k is any number greater than 1.
Let's apply this to three standard deviations (k = 3):
1 - 1/3² = 1 - 1/9 = 8/9 ≈ 0.889 or 88.9%
This tells us that, regardless of the distribution's shape, at least 88.9% of the data must fall within three standard deviations of the mean. This is a much less precise estimate than the empirical rule's 99.7%, but it holds true for any distribution, not just normal ones.
Comparing Empirical Rule and Chebyshev's Inequality
The key difference lies in their applicability:
- Empirical Rule: Applies specifically to approximately normal distributions. Provides a more precise estimate (99.7%).
- Chebyshev's Inequality: Applies to any distribution, regardless of its shape. Provides a lower bound, which is less precise (at least 88.9% for three standard deviations).
Practical Applications and Interpretations
Understanding the percentage of data within three standard deviations has significant practical implications across various fields:
1. Quality Control
In manufacturing, three-sigma limits (three standard deviations from the mean) are often used to define acceptable ranges for product quality. Data points falling outside this range might indicate a problem in the production process, requiring investigation and adjustments. The 99.7% from the empirical rule suggests that only a very small percentage of products should fall outside these limits if the process is stable.
2. Finance
In finance, three-standard deviation events are often considered rare or extreme. For example, a three-standard deviation move in a stock price might indicate significant market volatility or a potential outlier event requiring further analysis. This can trigger alerts for risk management systems.
3. Healthcare
In medical research, understanding the distribution of biological parameters can be critical. Three standard deviations from the mean might indicate an unusual result that needs further evaluation, especially if it relates to an indicator of health or disease.
4. Data Analysis and Anomaly Detection
Identifying outliers is essential in data analysis. Data points lying outside three standard deviations from the mean are frequently flagged as potential outliers. However, it's crucial to remember that outliers might not always be errors; they could represent significant findings or require further investigation to determine their cause.
Limitations and Considerations
It's crucial to acknowledge the limitations of relying solely on the empirical rule or Chebyshev's inequality:
- Non-Normal Distributions: The empirical rule is only accurate for approximately normal distributions. For skewed or heavily tailed distributions, the actual percentage within three standard deviations will differ significantly from 99.7%.
- Sample Size: The accuracy of both the empirical rule and Chebyshev's inequality improves with larger sample sizes. For small samples, the estimates might be less reliable.
- Outliers: Outliers can significantly distort the mean and standard deviation, affecting the accuracy of the estimates. Robust statistical methods might be necessary in such cases.
- False Positives/Negatives: In quality control or anomaly detection, using three-sigma limits might lead to false positives (flagging good data as problematic) or false negatives (missing true problems). Setting appropriate thresholds requires careful consideration of the specific context and potential risks.
Conclusion: Context is Key
While the statement that approximately 99.7% of data falls within three standard deviations of the mean is a valuable rule of thumb for normally distributed data, it's crucial to remember its limitations and consider the specific context. For non-normal distributions, Chebyshev's inequality offers a more general, although less precise, estimate. The choice between these approaches, and the interpretation of the results, should always be informed by a thorough understanding of the data and the implications of potential errors. Understanding standard deviation and its relation to probability empowers informed decision-making across numerous disciplines, allowing for better analysis and more effective strategies. Always remember to consider the potential limitations and adjust your interpretation accordingly for each specific application.
Latest Posts
Latest Posts
-
3 Oz Dry Pasta To Cooked
May 15, 2025
-
Greatest Common Factor Of 8 And 9
May 15, 2025
-
A Right Rectangular Prism Has A Height Of 9 Inches
May 15, 2025
-
A Desk Is How Many Meters High
May 15, 2025
-
Cuantas Calorias Debo Consumir Al Dia Para Ganar Masa Muscular
May 15, 2025
Related Post
Thank you for visiting our website which covers about What Percent Is 3 Standard Deviations . We hope the information provided has been useful to you. Feel free to contact us if you have any questions or need further assistance. See you next time and don't miss to bookmark.