Z Score Test Statistic Without Standard Deviation
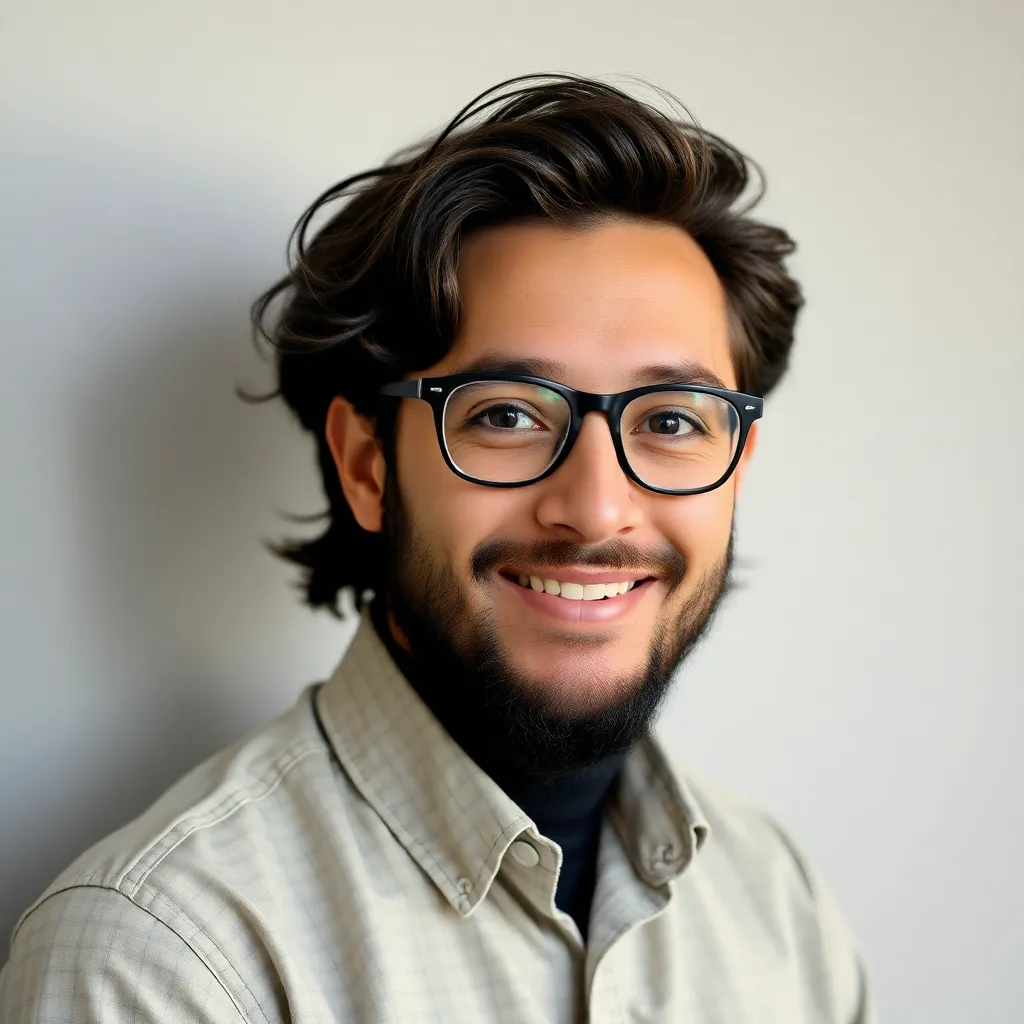
Treneri
Apr 11, 2025 · 6 min read
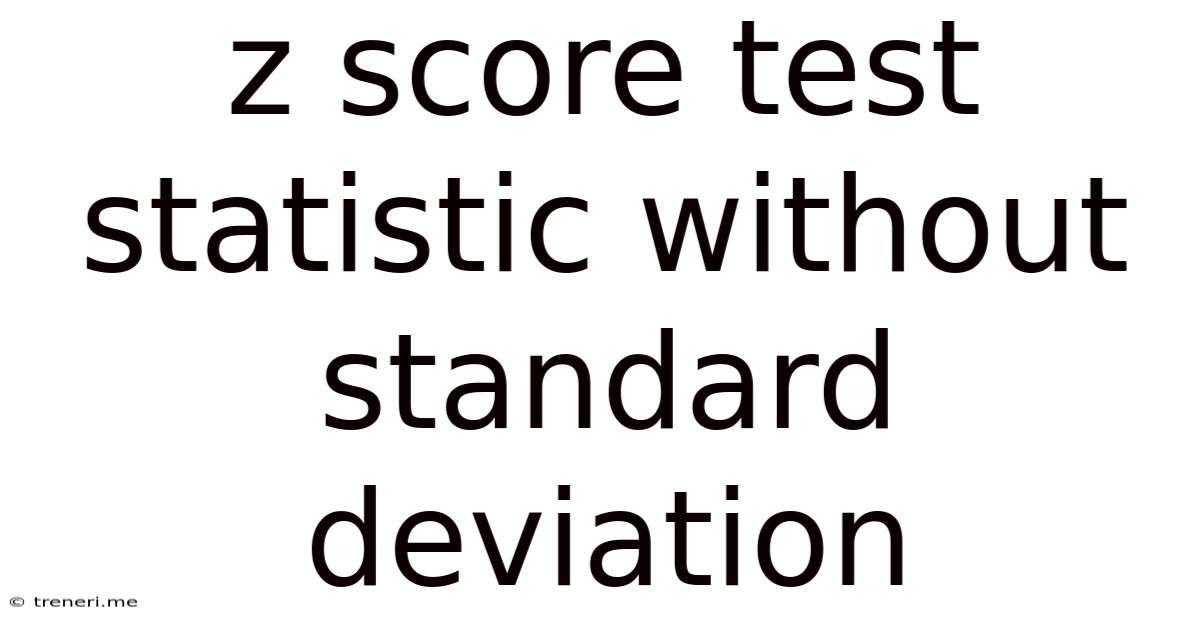
Table of Contents
Z-Score Test Statistic Without Standard Deviation: Understanding and Applications
The Z-score, a cornerstone of statistical analysis, is typically calculated using the population standard deviation. However, scenarios often arise where this parameter is unknown. This article delves into the intricacies of performing a Z-test when the population standard deviation is unavailable, exploring alternative approaches and their implications. We’ll examine the crucial role of the sample standard deviation, the conditions for valid application, and the practical implications in various statistical contexts.
Understanding the Z-Score and its Dependence on Standard Deviation
The Z-score quantifies the distance between a data point and the population mean in terms of standard deviations. A positive Z-score indicates the data point lies above the mean, while a negative Z-score signifies it's below the mean. The formula for calculating a Z-score is:
Z = (X - μ) / σ
Where:
- X is the individual data point
- μ is the population mean
- σ is the population standard deviation
The standard deviation (σ) acts as the scaling factor, normalizing the difference between X and μ. Without σ, we lose this crucial normalization, rendering the direct Z-score calculation impossible.
When the Population Standard Deviation is Unknown: Introducing the t-Test
When the population standard deviation (σ) is unknown, which is far more common in real-world applications, we must resort to an alternative approach. The most widely accepted method is to employ the t-test, replacing the population standard deviation with the sample standard deviation (s).
The formula for the t-statistic is:
t = (X̄ - μ) / (s / √n)
Where:
- X̄ is the sample mean
- μ is the population mean (often a hypothesized value)
- s is the sample standard deviation
- n is the sample size
Key Differences Between Z-test and t-test:
Feature | Z-test | t-test |
---|---|---|
Standard Deviation | Population standard deviation (σ) known | Population standard deviation (σ) unknown |
Distribution | Standard normal distribution | t-distribution (degrees of freedom = n-1) |
Sample Size | Typically large (n > 30) | Can be used with smaller sample sizes |
Critical Values | Obtained from the Z-table | Obtained from the t-table |
The Role of the Sample Standard Deviation (s)
The sample standard deviation (s) is an estimate of the population standard deviation (σ), calculated from the sample data. It measures the dispersion or spread of the data points within the sample. The accuracy of this estimate increases with larger sample sizes. Using 's' introduces variability into the test statistic, leading to the t-distribution instead of the normal distribution.
Understanding the t-Distribution:
Unlike the normal distribution, the t-distribution is characterized by its degrees of freedom (df), which are typically n-1 (sample size minus 1). With smaller sample sizes, the t-distribution has heavier tails than the normal distribution, reflecting the increased uncertainty in estimating σ using s. As the sample size increases (n approaches infinity), the t-distribution approaches the normal distribution.
Conditions for Valid Application of the t-Test:
The accuracy and validity of the t-test rely on certain assumptions:
- Random Sampling: The sample data should be randomly selected from the population to ensure representativeness.
- Independence: Observations within the sample should be independent of each other.
- Normality (approximately): The population data should be approximately normally distributed, or the sample size should be sufficiently large (often n > 30) due to the Central Limit Theorem. The Central Limit Theorem states that the sampling distribution of the sample mean approaches a normal distribution as the sample size increases, regardless of the shape of the population distribution. However, for small sample sizes, deviations from normality can significantly impact the results. Tests for normality, such as the Shapiro-Wilk test, can be performed to assess the assumption of normality.
- Data Type: The data should be continuous or at least ordinal for interval or ratio level analysis.
Interpreting t-Test Results:
The t-test statistic is compared to a critical value from the t-distribution based on the chosen significance level (alpha) and degrees of freedom. If the absolute value of the calculated t-statistic exceeds the critical value, the null hypothesis is rejected, suggesting a statistically significant difference between the sample mean and the hypothesized population mean.
Practical Applications of the t-Test When Standard Deviation is Unknown:
The t-test finds broad applicability across diverse fields:
-
Hypothesis Testing: Assessing whether there's a significant difference between a sample mean and a hypothesized population mean. For example, testing if the average height of students in a specific school differs significantly from the national average height.
-
Comparing Two Means: Comparing the means of two independent groups (independent samples t-test) or paired observations (paired samples t-test). For instance, comparing the effectiveness of two different medications on blood pressure.
-
Confidence Intervals: Constructing confidence intervals around the sample mean to estimate the range within which the true population mean likely lies. This provides a measure of uncertainty associated with the sample mean estimation.
Addressing Violations of Assumptions:
-
Non-Normality: If the normality assumption is violated, particularly with smaller sample sizes, non-parametric alternatives to the t-test can be considered, such as the Mann-Whitney U test (for independent samples) or the Wilcoxon signed-rank test (for paired samples). These tests do not assume normality.
-
Non-Independence: If data points are not independent (e.g., repeated measures on the same subject), alternative statistical models should be used, such as repeated measures ANOVA or mixed-effects models.
Choosing Between Z-Test and t-Test:
The choice between a Z-test and a t-test depends entirely on whether the population standard deviation is known. If the population standard deviation is known, a Z-test is appropriate. However, if the population standard deviation is unknown (which is almost always the case in real-world scenarios), then a t-test is the appropriate statistical tool. The t-test is more robust and widely applicable due to its ability to handle situations with unknown population standard deviations.
Conclusion:
While the Z-score formula directly utilizes the population standard deviation, its practical limitations lead us to the t-test when this parameter is unknown. Understanding the nuances of the t-distribution, its assumptions, and the role of the sample standard deviation are critical for accurate statistical inferences. The t-test provides a powerful and versatile tool for hypothesis testing and confidence interval estimation in numerous applications, significantly enhancing the analytical capabilities of researchers and practitioners across various domains. Always remember to check the assumptions of the t-test before interpreting the results, and to consider non-parametric alternatives if those assumptions are violated. Proper application ensures reliable and meaningful conclusions from your statistical analysis.
Latest Posts
Latest Posts
-
Cuanto Es El 3 De 100
May 09, 2025
-
Como Se Mide La Humedad Relativa
May 09, 2025
-
What Is The Equivalent Fraction For 5 6
May 09, 2025
-
What Is An Equivalent Fraction To 4 5
May 09, 2025
-
95 Grams Is How Many Ounces
May 09, 2025
Related Post
Thank you for visiting our website which covers about Z Score Test Statistic Without Standard Deviation . We hope the information provided has been useful to you. Feel free to contact us if you have any questions or need further assistance. See you next time and don't miss to bookmark.