Chi-square Calculator For Goodness Of Fit
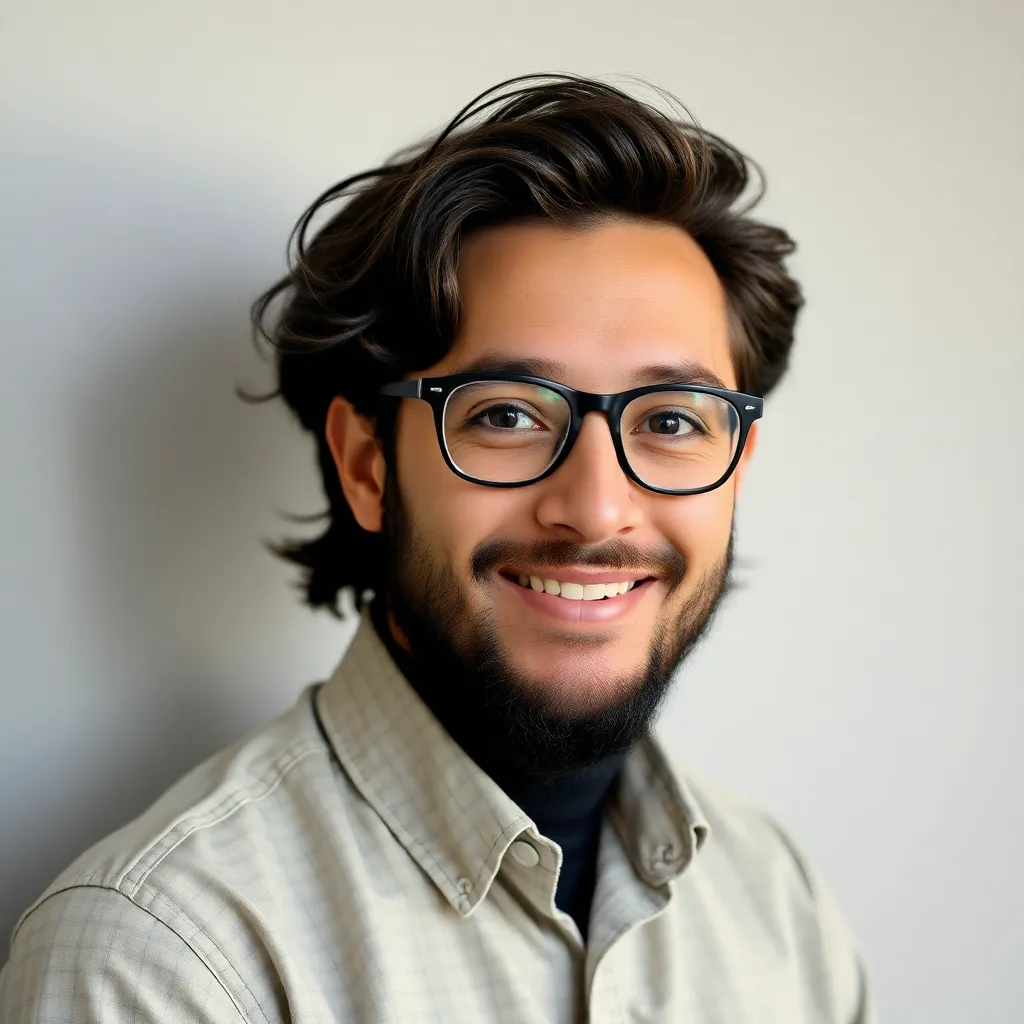
Treneri
May 15, 2025 · 6 min read
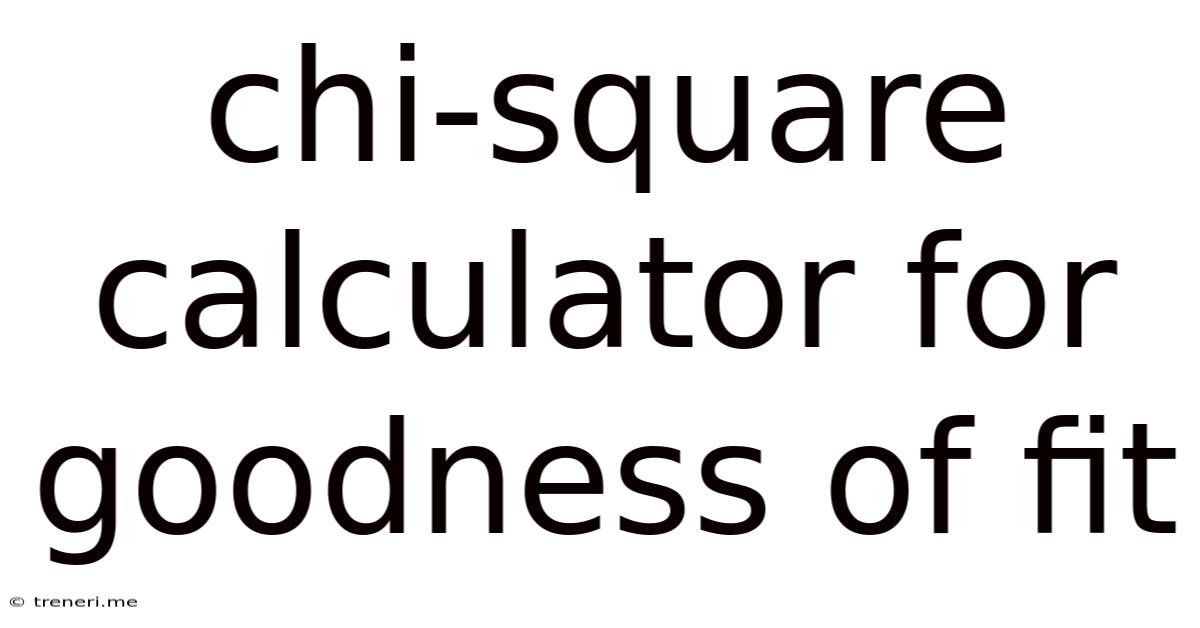
Table of Contents
Chi-Square Calculator for Goodness of Fit: A Comprehensive Guide
The chi-square goodness-of-fit test is a powerful statistical tool used to determine how well sample data fits a hypothesized distribution. This test is invaluable across numerous fields, from biology and medicine to social sciences and marketing, allowing researchers to assess the validity of their models and assumptions. Understanding how to use a chi-square calculator for goodness of fit is crucial for interpreting results accurately and drawing meaningful conclusions. This comprehensive guide will walk you through the process, explaining the underlying concepts, the steps involved, and providing practical examples.
Understanding the Chi-Square Goodness-of-Fit Test
Before diving into the mechanics of using a chi-square calculator, let's establish a clear understanding of the test's purpose and assumptions. The chi-square goodness-of-fit test assesses whether the observed frequencies of categorical data differ significantly from the expected frequencies based on a hypothesized distribution. In simpler terms, it helps determine if your sample data aligns with a specific theoretical model or distribution (e.g., uniform, normal, binomial).
Key Assumptions:
- Independence: The observations in your sample must be independent of each other. This means that one observation's outcome doesn't influence another.
- Random Sampling: The data must be collected through a random sampling method to ensure the sample accurately represents the population.
- Expected Frequencies: Each expected frequency (the number of observations you'd expect under the hypothesized distribution) should be at least 5. This assumption ensures the chi-square distribution is a reasonable approximation. If some expected frequencies are less than 5, you might need to combine categories or consider alternative tests.
Calculating the Chi-Square Statistic
The core of the chi-square goodness-of-fit test involves calculating the chi-square statistic (χ²), which measures the discrepancy between observed and expected frequencies. The formula for the chi-square statistic is:
χ² = Σ [(Oᵢ - Eᵢ)² / Eᵢ]
Where:
- Oᵢ = Observed frequency for category i
- Eᵢ = Expected frequency for category i
- Σ = Summation across all categories
Let's break this down: For each category, you calculate the difference between the observed and expected frequencies (Oᵢ - Eᵢ), square this difference, and then divide by the expected frequency. Finally, you sum these values across all categories to obtain the chi-square statistic.
Step-by-Step Calculation: A Practical Example
Imagine a study examining the distribution of eye color in a population. The hypothesized distribution is as follows: 40% brown, 30% blue, 20% green, and 10% hazel. A sample of 200 individuals yielded the following observed frequencies:
- Brown: 70
- Blue: 65
- Green: 45
- Hazel: 20
1. Calculate Expected Frequencies:
Based on the hypothesized distribution and sample size (200), the expected frequencies are:
- Brown: 200 * 0.40 = 80
- Blue: 200 * 0.30 = 60
- Green: 200 * 0.20 = 40
- Hazel: 200 * 0.10 = 20
2. Calculate the Chi-Square Statistic:
Now, we apply the formula:
- Brown: [(70 - 80)² / 80] = 1.25
- Blue: [(65 - 60)² / 60] = 0.42
- Green: [(45 - 40)² / 40] = 0.625
- Hazel: [(20 - 20)² / 20] = 0
χ² = 1.25 + 0.42 + 0.625 + 0 = 2.295
Interpreting the Chi-Square Statistic Using a Calculator
The calculated chi-square statistic (2.295 in our example) needs to be compared to a critical value obtained from a chi-square distribution table or using a chi-square calculator. This comparison helps determine the probability of obtaining the observed frequencies if the hypothesized distribution were true. This probability is called the p-value.
Degrees of Freedom:
Before using a chi-square calculator, you need to determine the degrees of freedom (df). For a goodness-of-fit test, the degrees of freedom are calculated as:
df = k - 1
Where 'k' is the number of categories. In our eye color example, k = 4, so df = 4 - 1 = 3.
Using a Chi-Square Calculator:
Many online calculators are available. Simply input the calculated chi-square statistic (2.295), the degrees of freedom (3), and select the significance level (alpha), usually 0.05. The calculator will provide the p-value.
Interpreting the p-value:
- p-value ≤ α: If the p-value is less than or equal to the significance level (e.g., 0.05), you reject the null hypothesis. This means there is sufficient evidence to conclude that the observed frequencies differ significantly from the expected frequencies, and the hypothesized distribution is not a good fit for the data.
- p-value > α: If the p-value is greater than the significance level, you fail to reject the null hypothesis. This means there is not enough evidence to reject the hypothesized distribution. The observed frequencies are reasonably consistent with the expected frequencies.
In our example, a chi-square calculator with α = 0.05 and df = 3 would likely yield a p-value greater than 0.05, leading to a failure to reject the null hypothesis. This suggests that the observed eye color distribution in the sample is reasonably consistent with the hypothesized distribution.
Advanced Considerations and Applications
While the basic chi-square goodness-of-fit test is straightforward, several advanced considerations can enhance its application and interpretation:
Yates' Correction for Continuity
When dealing with small sample sizes or small expected frequencies, Yates' correction for continuity can improve the accuracy of the test. This correction adjusts the chi-square statistic by subtracting 0.5 from the absolute difference between observed and expected frequencies before squaring. Many chi-square calculators offer the option to include Yates' correction.
Choosing the Right Significance Level (α)
The significance level (α) represents the probability of rejecting the null hypothesis when it is actually true (Type I error). The commonly used value is 0.05, but the choice depends on the context and the consequences of making a Type I error. A lower alpha reduces the risk of a Type I error but increases the risk of a Type II error (failing to reject a false null hypothesis).
Limitations of the Chi-Square Test
It's crucial to acknowledge the limitations:
- Sensitivity to Sample Size: With extremely large samples, even small deviations from the expected frequencies can lead to statistically significant results, even if the practical significance is minimal.
- Categorical Data Only: The chi-square goodness-of-fit test is designed for categorical data, not continuous data.
- Assumption Violations: Violating the assumptions (independence, random sampling, expected frequencies) can invalidate the results.
Real-World Applications of the Chi-Square Goodness-of-Fit Test
The versatility of the chi-square goodness-of-fit test makes it applicable across diverse fields:
- Genetics: Testing whether observed genotype frequencies match Hardy-Weinberg equilibrium expectations.
- Quality Control: Assessing whether the proportion of defective items in a production process conforms to a specified standard.
- Marketing: Determining if customer preferences for different product features align with market research predictions.
- Medicine: Comparing the distribution of a disease across different demographic groups to established epidemiological data.
- Sociology: Investigating whether the distribution of political affiliations in a sample reflects the known population distribution.
Conclusion
The chi-square goodness-of-fit test is a valuable tool for assessing how well sample data aligns with a theoretical distribution. Using a chi-square calculator simplifies the process of calculating the test statistic and obtaining the p-value, enabling researchers to draw informed conclusions about the fit of their data. Understanding the assumptions, limitations, and interpretations is critical for proper application and avoiding misinterpretations. By mastering the use of the chi-square calculator and understanding its nuances, researchers can confidently apply this powerful statistical technique in their work. Remember to always consider the context, sample size, and potential limitations when interpreting the results.
Latest Posts
Latest Posts
-
1 Divided By The Square Root Of 2
May 15, 2025
-
Which Square Root Is Between 4 And 5
May 15, 2025
-
Select The Binary Representation Of 51
May 15, 2025
-
What Is The Gcf Of 10 And 12
May 15, 2025
-
Common Factors Of 16 And 42
May 15, 2025
Related Post
Thank you for visiting our website which covers about Chi-square Calculator For Goodness Of Fit . We hope the information provided has been useful to you. Feel free to contact us if you have any questions or need further assistance. See you next time and don't miss to bookmark.