Find Point Estimate Of Population Mean
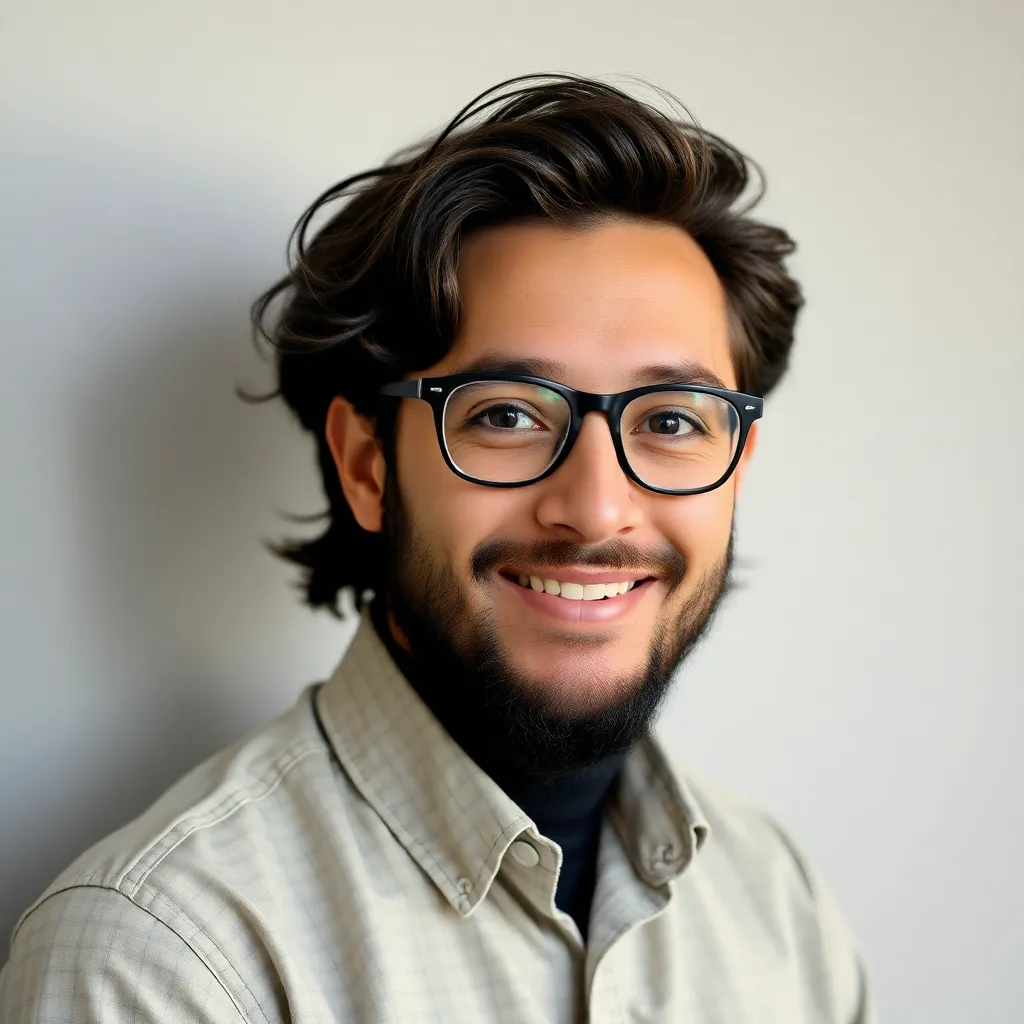
Treneri
Apr 06, 2025 · 6 min read

Table of Contents
Finding the Point Estimate of the Population Mean: A Comprehensive Guide
Estimating population parameters from sample data is a cornerstone of statistical inference. One of the most fundamental estimations is finding the point estimate of the population mean (μ). This article provides a comprehensive guide to understanding, calculating, and interpreting point estimates, exploring various scenarios and considerations along the way.
Understanding Point Estimates and the Population Mean
Before diving into calculations, let's clarify some key concepts. The population mean (μ) represents the average value of a characteristic across an entire population. However, it's often impractical or impossible to measure the entire population. This is where sampling comes in. We collect data from a representative sample and use it to estimate the population mean.
A point estimate is a single value derived from sample data that serves as the best guess for the population parameter. In the context of the population mean, the point estimate is typically the sample mean (x̄).
In simpler terms: Imagine you want to know the average height of all adult women in a country. Measuring every woman is impossible. Instead, you measure the height of a sample of women and calculate their average height. This average height from your sample is the point estimate of the population mean height.
Calculating the Sample Mean (x̄)
The sample mean is the most common and straightforward point estimate for the population mean. It's calculated by summing all the values in the sample and dividing by the number of observations. The formula is:
x̄ = Σx / n
Where:
- x̄ represents the sample mean
- Σx represents the sum of all values in the sample
- n represents the sample size
Example:
Let's say you have a sample of five test scores: 85, 92, 78, 88, and 95. To calculate the sample mean:
- Sum the values: 85 + 92 + 78 + 88 + 95 = 438
- Divide by the sample size: 438 / 5 = 87.6
Therefore, the sample mean (and point estimate of the population mean) is 87.6.
Properties of a Good Point Estimator
While the sample mean is the most commonly used point estimate, it's crucial to understand the properties that make an estimator desirable:
-
Unbiasedness: An unbiased estimator has an expected value equal to the population parameter. The sample mean is an unbiased estimator of the population mean. This means that if you were to take many samples and calculate the mean of each, the average of those sample means would be very close to the true population mean.
-
Efficiency: An efficient estimator has a smaller variance (less spread) compared to other estimators. The sample mean is a relatively efficient estimator, meaning it tends to provide estimates closer to the true population mean than some other methods.
-
Consistency: A consistent estimator’s accuracy increases as the sample size increases. The sample mean is consistent; as you take larger samples, the sample mean gets closer and closer to the true population mean.
-
Sufficiency: A sufficient estimator uses all the information available in the sample to estimate the parameter. The sample mean is a sufficient estimator of the population mean.
Confidence Intervals: Beyond Point Estimates
While a point estimate provides a single best guess, it doesn't capture the uncertainty inherent in using a sample to estimate a population parameter. To address this, we use confidence intervals. A confidence interval provides a range of values within which the true population mean is likely to fall, with a specified level of confidence.
For example, a 95% confidence interval means there's a 95% probability that the interval contains the true population mean. The formula for a confidence interval depends on whether the population standard deviation (σ) is known or unknown.
Confidence Interval when Population Standard Deviation (σ) is Known
When the population standard deviation is known, we use the z-distribution:
Confidence Interval = x̄ ± z(σ/√n)*
Where:
- x̄ is the sample mean
- z is the z-score corresponding to the desired confidence level (e.g., 1.96 for 95% confidence)
- σ is the population standard deviation
- n is the sample size
Confidence Interval when Population Standard Deviation (σ) is Unknown
In most real-world scenarios, the population standard deviation is unknown. In this case, we use the sample standard deviation (s) and the t-distribution:
Confidence Interval = x̄ ± t(s/√n)*
Where:
- x̄ is the sample mean
- t is the t-score corresponding to the desired confidence level and degrees of freedom (n-1)
- s is the sample standard deviation
- n is the sample size
Sample Size and Margin of Error
The margin of error is the distance between the point estimate and the upper or lower bound of the confidence interval. A smaller margin of error indicates a more precise estimate. The margin of error is influenced by:
- Sample size (n): Larger samples generally lead to smaller margins of error.
- Confidence level: Higher confidence levels require wider intervals and larger margins of error.
- Population standard deviation (σ or s): Larger standard deviations result in larger margins of error.
Choosing the Right Method: Considerations and Assumptions
The choice of method for calculating the point estimate and confidence interval depends on several factors:
-
Data Type: The methods described above assume numerical data. For categorical data, different techniques are necessary.
-
Sampling Method: The validity of the point estimate and confidence interval relies on the use of a proper random sampling method. Bias in the sampling process can lead to inaccurate estimations.
-
Population Distribution: The formulas assume that the population is approximately normally distributed, or that the sample size is large enough for the Central Limit Theorem to apply (generally, n ≥ 30). If the population is significantly non-normal and the sample size is small, non-parametric methods might be more appropriate.
-
Outliers: Outliers can heavily influence the sample mean. It's important to identify and potentially address outliers before calculating the point estimate.
Interpreting Results and Communicating Findings
Once you've calculated the point estimate and confidence interval, it's crucial to interpret the results clearly and communicate them effectively. For example, a statement like "Based on our sample, we estimate the average income to be $50,000, with a 95% confidence interval of $48,000 to $52,000" is much more informative than simply stating the point estimate.
Remember to highlight the limitations of the study, including the sample size, sampling method, and any assumptions made. This ensures that the findings are interpreted accurately and avoids overgeneralization.
Advanced Techniques and Further Exploration
This article provides a foundation for understanding point estimates of the population mean. There are more advanced techniques to explore, including:
-
Bayesian estimation: This approach incorporates prior knowledge about the population parameter into the estimation process.
-
Maximum likelihood estimation (MLE): MLE finds the parameter values that maximize the likelihood of observing the sample data.
-
Bootstrap methods: These methods use resampling techniques to estimate the sampling distribution of the point estimate, allowing for more robust inference.
-
Dealing with complex data structures: Methods for handling clustered data, longitudinal data, and other complex data structures exist, going beyond simple random sampling.
Conclusion
The point estimate of the population mean is a fundamental concept in statistical inference. Understanding how to calculate, interpret, and communicate these estimates is essential for anyone working with data. While the sample mean provides a straightforward and often effective point estimate, remember the importance of confidence intervals to quantify the uncertainty associated with the estimate. By considering the properties of good estimators and the assumptions underlying the methods, you can ensure the accuracy and reliability of your statistical inferences. Further exploration of more advanced techniques will enhance your ability to analyze data effectively and draw meaningful conclusions.
Latest Posts
Latest Posts
-
A Ramp Is In The Shape Of A Triangular Prism
May 09, 2025
-
Cuanto Es 100 Grados Fahrenheit A Centigrados
May 09, 2025
-
How Much Is 31 Km In Miles
May 09, 2025
-
How Many Calories Does The Elliptical Burn In 30 Minutes
May 09, 2025
-
What Is 1 Percent Of 15
May 09, 2025
Related Post
Thank you for visiting our website which covers about Find Point Estimate Of Population Mean . We hope the information provided has been useful to you. Feel free to contact us if you have any questions or need further assistance. See you next time and don't miss to bookmark.