What Is The Raw Score In Statistics
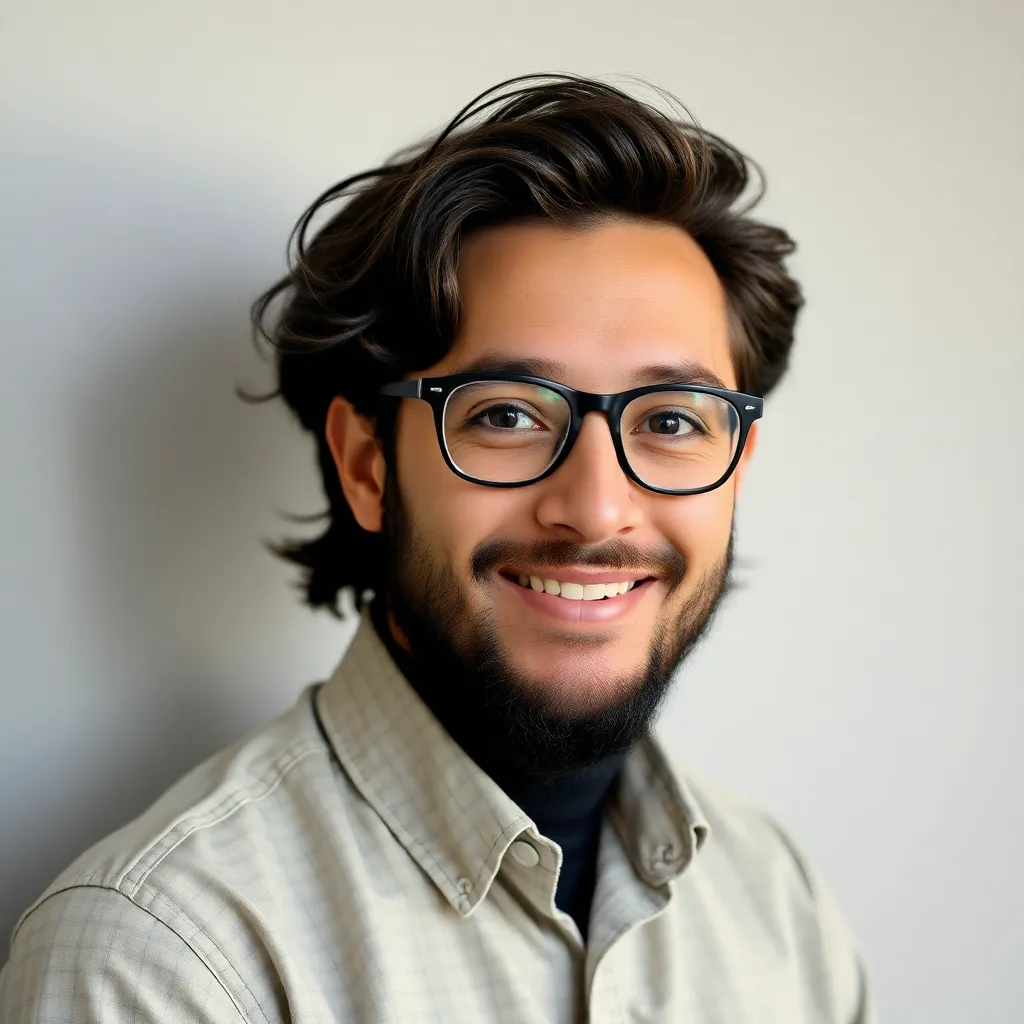
Treneri
May 14, 2025 · 7 min read
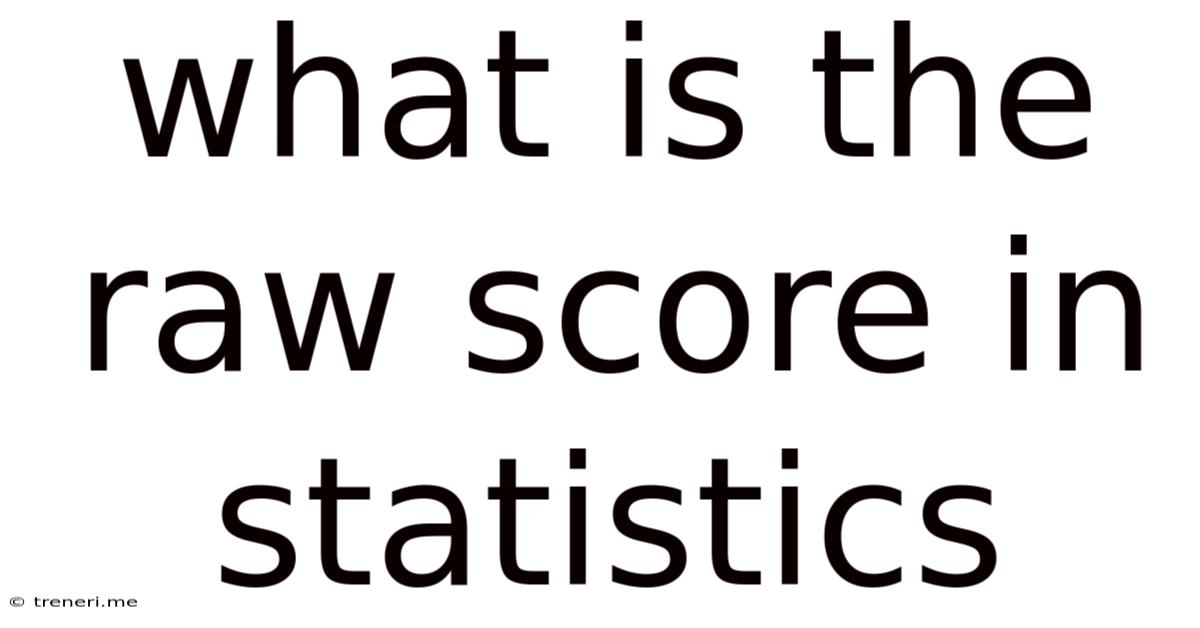
Table of Contents
What is a Raw Score in Statistics? A Comprehensive Guide
Understanding raw scores is fundamental to comprehending various statistical concepts. This comprehensive guide delves deep into the meaning, calculation, interpretation, and limitations of raw scores, providing a solid foundation for both beginners and those seeking a refresher. We'll explore how raw scores relate to other statistical measures and discuss their importance in various fields.
Defining Raw Scores: The Unprocessed Data
In statistics, a raw score is simply the original, unprocessed data point collected in a study or experiment. It represents a single observation without any transformation or manipulation. These scores are the basic building blocks upon which statistical analyses are built. Imagine you're conducting a study on student exam scores. Each individual student's score on the exam before any calculations are performed is a raw score. They are the initial, untransformed numerical values.
Examples of Raw Scores Across Different Contexts:
- Educational Testing: A student's score on a standardized test (e.g., 85 out of 100).
- Medical Research: A patient's blood pressure reading (e.g., 120/80 mmHg).
- Psychological Assessments: A participant's score on a personality inventory (e.g., a score of 15 on an anxiety scale).
- Sports Analytics: A basketball player's points scored in a game (e.g., 25 points).
- Market Research: The number of units of a product sold in a specific region (e.g., 5000 units).
The key characteristic of a raw score is that it's the direct result of measurement and hasn't been altered in any way. This makes them the starting point for all statistical analyses.
The Importance of Raw Scores in Statistical Analysis
While raw scores might seem simple, they are crucial for several reasons:
- Foundation for other statistical measures: Raw scores are the essential input for calculating numerous statistical measures, including the mean, median, mode, standard deviation, variance, and percentiles. These summary statistics provide a more concise and interpretable understanding of the data than raw scores alone.
- Understanding individual performance or characteristics: Raw scores directly reflect individual performance or characteristics without being masked by transformations or standardizations. This is particularly relevant in educational settings where a student's raw score on a test provides a direct measure of their performance.
- Basis for comparisons (within and between groups): Raw scores allow for direct comparisons between individuals within the same group or between different groups. However, interpreting these comparisons effectively requires additional statistical tools.
- Data integrity and verification: Maintaining the original raw scores ensures data integrity. If there are errors in the raw data, it will be reflected in any calculations based on it. Having access to raw scores enables error detection and correction.
Limitations of Raw Scores: Why Transformations are Necessary
Despite their importance, raw scores have significant limitations:
- Difficult to interpret on their own: A single raw score provides little information without context. For example, a score of 75 on an exam doesn't tell us much unless we know the average score and the distribution of scores.
- Difficult to compare across different scales: Raw scores from different tests or scales are inherently incomparable unless they're standardized. For example, comparing a student's raw score on a math test to their raw score on a history test is meaningless without understanding the scoring systems and distributions of each test.
- Sensitive to the scale of measurement: The interpretation of a raw score is strongly influenced by the scale used. For example, a weight of 150 pounds is different from a score of 150 on a personality inventory.
These limitations highlight the necessity of transforming raw scores into more interpretable and comparable measures.
Transforming Raw Scores: Standardization and Other Methods
To overcome the limitations of raw scores, various transformation methods are used, the most common of which is standardization.
Standardization: Z-scores and T-scores
Standardization converts raw scores into standard scores, which are expressed in terms of standard deviations from the mean. The most common standardized scores are:
-
Z-scores: Z-scores represent the number of standard deviations a raw score falls above or below the mean of the distribution. A Z-score of 0 indicates the raw score is equal to the mean, a positive Z-score indicates it is above the mean, and a negative Z-score indicates it is below the mean.
-
T-scores: T-scores are another type of standardized score, typically with a mean of 50 and a standard deviation of 10. They are often preferred over Z-scores because they avoid negative values, making them easier to interpret and understand, especially for individuals unfamiliar with negative numbers within statistical analysis.
The formulas for Z-scores and T-scores are as follows:
- Z-score = (Raw Score - Mean) / Standard Deviation
- T-score = (Z-score * 10) + 50
Standardization allows for meaningful comparisons of raw scores from different distributions and different scales. It creates a common metric for comparing performance across various tests or assessments.
Other Transformation Methods:
Besides Z-scores and T-scores, other transformations are also employed depending on the data's nature and the analysis's goals. These include:
- Percentile Ranks: This expresses a raw score in terms of the percentage of scores falling below it in a distribution. A percentile rank of 80 means that the individual scored higher than 80% of the individuals in the group.
- Rank-based transformations: These transformations assign ranks to raw scores based on their relative positions within a distribution. The highest score receives rank 1, the second highest receives rank 2, and so on.
Interpreting Raw Scores and Transformed Scores
The interpretation of raw scores and their transformations depends heavily on context. The following considerations are crucial:
- The nature of the data: Understanding whether the data is normally distributed is crucial, as many statistical methods assume normality.
- The scale of measurement: The type of scale used (nominal, ordinal, interval, ratio) affects how the scores are interpreted.
- The reference group: The interpretation of a raw score changes depending on the reference group used for comparison.
- The purpose of the analysis: The statistical methods used and their interpretations depend on the study's goal.
Raw Scores in Different Fields
Raw scores play a vital role in various disciplines:
Education:
In educational settings, raw scores are the initial test scores obtained by students. These scores are then used to calculate grades, compare student performance, and evaluate the effectiveness of teaching methods. Transformations like percentile ranks are often used to provide a more interpretable understanding of a student's position relative to their peers.
Psychology:
In psychology, raw scores are frequently obtained from psychological assessments like personality tests or intelligence tests. These scores are often transformed into standardized scores to facilitate comparisons between individuals and to diagnose psychological conditions.
Medicine:
In medicine, raw scores might represent measurements like blood pressure, heart rate, or the results of medical imaging. These raw scores are often used in conjunction with other clinical data to diagnose and monitor patient conditions.
Sports Analytics:
Sports analysts use raw scores, like points scored in a basketball game, to evaluate the performance of athletes. These raw scores are then often used to calculate more advanced metrics such as points per game or field goal percentage, which help in making strategic decisions.
Conclusion: The Unassuming Power of Raw Scores
Raw scores, though seemingly basic, are the cornerstone of statistical analysis. They represent the unprocessed data that forms the basis for more sophisticated measures and interpretations. While raw scores alone often lack context and comparability, their transformation into standardized scores or other interpretable measures allows researchers and practitioners to draw meaningful conclusions and make informed decisions across diverse fields. Understanding raw scores and their limitations is crucial for anyone working with data and interpreting statistical findings. Remember that the proper interpretation of raw scores always depends on careful consideration of the context, the method of data collection, and the goals of the analysis.
Latest Posts
Latest Posts
-
20 Divided By 3 With Remainder
May 14, 2025
-
90 Days From Oct 22 2024
May 14, 2025
-
How To Find The Orthocenter Of A Triangle With Coordinates
May 14, 2025
-
Least Common Multiple Of 24 And 9
May 14, 2025
-
How Many Inches Is Approximately 1 M
May 14, 2025
Related Post
Thank you for visiting our website which covers about What Is The Raw Score In Statistics . We hope the information provided has been useful to you. Feel free to contact us if you have any questions or need further assistance. See you next time and don't miss to bookmark.