How To Find Point Estimate Of The Population Proportion
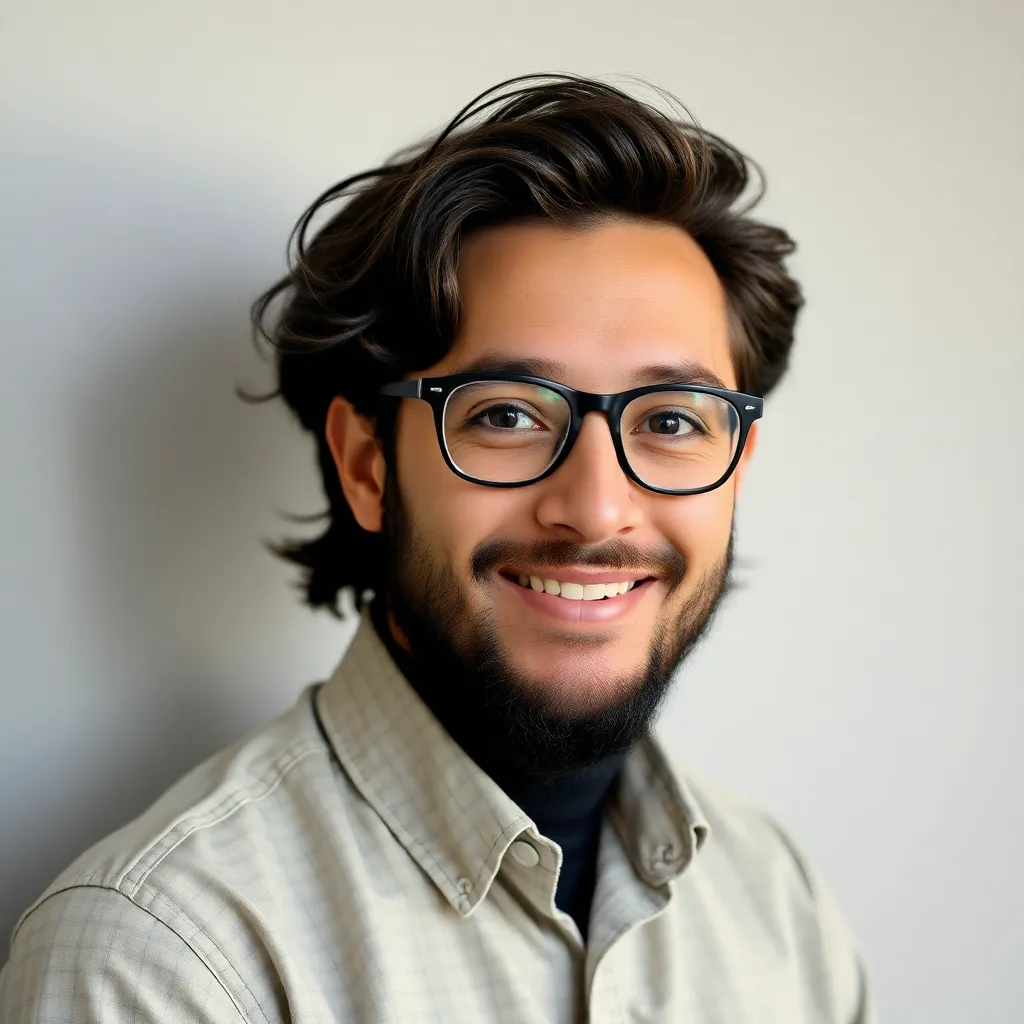
Treneri
May 13, 2025 · 6 min read

Table of Contents
How to Find the Point Estimate of the Population Proportion
Estimating population parameters from sample data is a cornerstone of statistical inference. One crucial parameter is the population proportion – the percentage of individuals in a population possessing a specific characteristic. Since examining an entire population is often impractical or impossible, we rely on sample data to provide a point estimate of this proportion. This article dives deep into the methods and considerations involved in finding this crucial estimate, covering everything from understanding the concept to tackling more complex scenarios.
Understanding Point Estimates and Population Proportions
Before delving into the mechanics, let's clarify the core concepts. A population proportion, denoted as p, represents the true proportion of individuals with a specific attribute within the entire population. For example, p could represent the proportion of voters who favor a particular candidate, the proportion of defective items in a manufacturing batch, or the proportion of adults with a specific medical condition.
A point estimate is a single value derived from sample data that serves as the best guess for the unknown population parameter. In the context of population proportions, the point estimate is denoted as p̂ (p-hat). It's a single number that we use to represent our best guess of the true population proportion. It's important to understand that a point estimate is just an estimate; it's unlikely to be exactly equal to the true population proportion, but it aims to be a close approximation.
Calculating the Point Estimate: The Simple Formula
The calculation of the point estimate for a population proportion is surprisingly straightforward. It involves only two pieces of information from your sample:
- x: The number of individuals in the sample possessing the characteristic of interest.
- n: The total sample size.
The formula for the point estimate is:
p̂ = x/n
Example: Let's say we're interested in estimating the proportion of students who prefer online learning. We randomly sample 100 students (n = 100), and 60 of them (x = 60) indicate a preference for online learning. The point estimate of the population proportion is:
p̂ = 60/100 = 0.6
This means our best guess for the proportion of students who prefer online learning in the entire student population is 0.6 or 60%.
Sampling Methods and Their Impact on Accuracy
The accuracy of your point estimate is heavily influenced by the sampling method employed. A random sample, where every member of the population has an equal chance of being selected, is crucial for minimizing bias and ensuring the estimate is representative of the population. Other methods, such as stratified sampling (dividing the population into strata and sampling from each) or cluster sampling (sampling clusters of individuals), can be useful in specific situations but require careful consideration to avoid introducing bias. Non-random sampling methods often lead to biased estimates that are not reliable representations of the population proportion.
Margin of Error and Confidence Intervals: Beyond the Point Estimate
While the point estimate provides a single value, it's crucial to acknowledge its inherent uncertainty. A point estimate alone doesn't convey the level of precision or the potential range within which the true population proportion might lie. This is where the concept of margin of error and confidence intervals become essential.
Understanding Margin of Error
The margin of error quantifies the uncertainty associated with the point estimate. It represents the likely range of error surrounding the point estimate. A smaller margin of error indicates higher precision. The margin of error is typically expressed as a plus or minus value added to and subtracted from the point estimate.
Constructing Confidence Intervals
A confidence interval provides a range of values within which we are confident the true population proportion lies. It's constructed using the point estimate, the margin of error, and a chosen confidence level (e.g., 95%, 99%). A 95% confidence interval means that if we were to repeat the sampling process many times, 95% of the resulting confidence intervals would contain the true population proportion.
The formula for a confidence interval is:
p̂ ± Z * √(p̂(1-p̂)/n)
Where:
p̂
is the sample proportionZ
is the Z-score corresponding to the desired confidence level (e.g., 1.96 for a 95% confidence level)n
is the sample size
Example: Using the previous example (p̂ = 0.6, n = 100), let's construct a 95% confidence interval:
0.6 ± 1.96 * √(0.6(1-0.6)/100)
This calculation yields a confidence interval of approximately (0.504, 0.696). We can be 95% confident that the true proportion of students who prefer online learning lies within this range.
Factors Affecting the Margin of Error and Confidence Interval Width
Several factors influence the width of the confidence interval (and therefore the margin of error):
- Sample Size (n): Larger sample sizes lead to narrower confidence intervals, providing more precise estimates. A larger sample is more representative of the population, reducing uncertainty.
- Confidence Level: Higher confidence levels (e.g., 99% vs. 95%) result in wider confidence intervals. To be more confident that the true population proportion is captured, a wider range is needed.
- Sample Proportion (p̂): The margin of error is largest when p̂ is close to 0.5. When p̂ is closer to 0 or 1, the margin of error decreases.
Handling Finite Populations
The formulas presented so far assume an infinitely large population or a very large population relative to the sample size. When dealing with a finite population (a population with a known size, N), a finite population correction factor should be applied to the margin of error formula to account for the fact that sampling without replacement from a finite population reduces variability. The adjusted formula incorporates a correction factor:
p̂ ± Z * √(p̂(1-p̂)/n) * √((N-n)/(N-1))
Where:
- N is the size of the finite population
Choosing the Appropriate Sample Size
Determining the appropriate sample size is crucial for achieving a desired level of precision. The required sample size depends on the desired margin of error, confidence level, and an estimated population proportion (often based on prior knowledge or a pilot study). Formulas exist to calculate the necessary sample size, but they often involve iterative calculations. Statistical software packages can simplify this process.
Beyond the Basics: Dealing with Complex Scenarios
The methods described above provide a solid foundation. However, more complex scenarios require adjustments:
- Stratified Sampling: When using stratified sampling, you'll calculate a point estimate and confidence interval for each stratum and then combine them using weighted averages, considering the size of each stratum.
- Cluster Sampling: Similar to stratified sampling, the analysis of cluster sampling involves adjusting the formulas to account for the clustering effect and potential increased variability.
- Non-response Bias: Non-response bias occurs when a significant portion of the selected sample fails to participate. This bias can affect the accuracy of the point estimate and necessitates careful consideration and potential adjustments in the analysis.
Conclusion
Finding the point estimate of the population proportion is a fundamental task in statistics. While the basic formula is straightforward, understanding the nuances of sampling methods, confidence intervals, margin of error, and appropriate sample sizes is crucial for accurate and reliable inference. By considering these factors and adapting the methods to the specific context, researchers and analysts can effectively estimate population proportions from sample data and draw meaningful conclusions. Remember, a point estimate provides a valuable initial understanding but should always be accompanied by a measure of uncertainty, such as a confidence interval, to provide a more complete picture.
Latest Posts
Latest Posts
-
How Much Is 250 Kilometers In Miles
May 13, 2025
-
What Is The Reciprocal Of 6 7
May 13, 2025
-
25 Out Of 32 As A Percentage
May 13, 2025
-
How Many Kilocalories Are In One Gram Of Carbohydrate
May 13, 2025
-
1 Raised To The Power Of 5
May 13, 2025
Related Post
Thank you for visiting our website which covers about How To Find Point Estimate Of The Population Proportion . We hope the information provided has been useful to you. Feel free to contact us if you have any questions or need further assistance. See you next time and don't miss to bookmark.